Learning minimum variance discrete hedging directly from the market
QUANTITATIVE FINANCE(2018)
摘要
Option hedging is a critical risk management problem in finance. In the Black-Scholes model, it has been recognized that computing a hedging position from the sensitivity of the calibrated model option value function is inadequate in minimizing variance of the option hedge risk, as it fails to capture the model parameter dependence on the underlying price (see e.g. Coleman et al., J. Risk, 2001, 5(6), 63-89; Hull and White, J. Bank. Finance, 2017, 82, 180-190). In this paper, we demonstrate that this issue can exist generally when determining hedging position from the sensitivity of the option function, either calibrated from a parametric model from current option prices or estimated nonparametricaly from historical option prices. Consequently, the sensitivity of the estimated model option function typically does not minimize variance of the hedge risk, even instantaneously. We propose a data-driven approach to directly learn a hedging function from the market data by minimizing variance of the local hedge risk. Using the S&P 500 index daily option data for more than a decade ending in August 2015, we show that the proposed method outperforms the parametric minimum variance hedging method proposed in Hull and White [J. Bank. Finance, 2017, 82, 180-190], as well as minimum variance hedging corrective techniques based on stochastic volatility or local volatility models. Furthermore, we show that the proposed approach achieves significant gain over the implied BS delta hedging for weekly and monthly hedging.
更多查看译文
关键词
Machine learning,Dynamic hedging,Risk management,Kernels,Regularized network
AI 理解论文
溯源树
样例
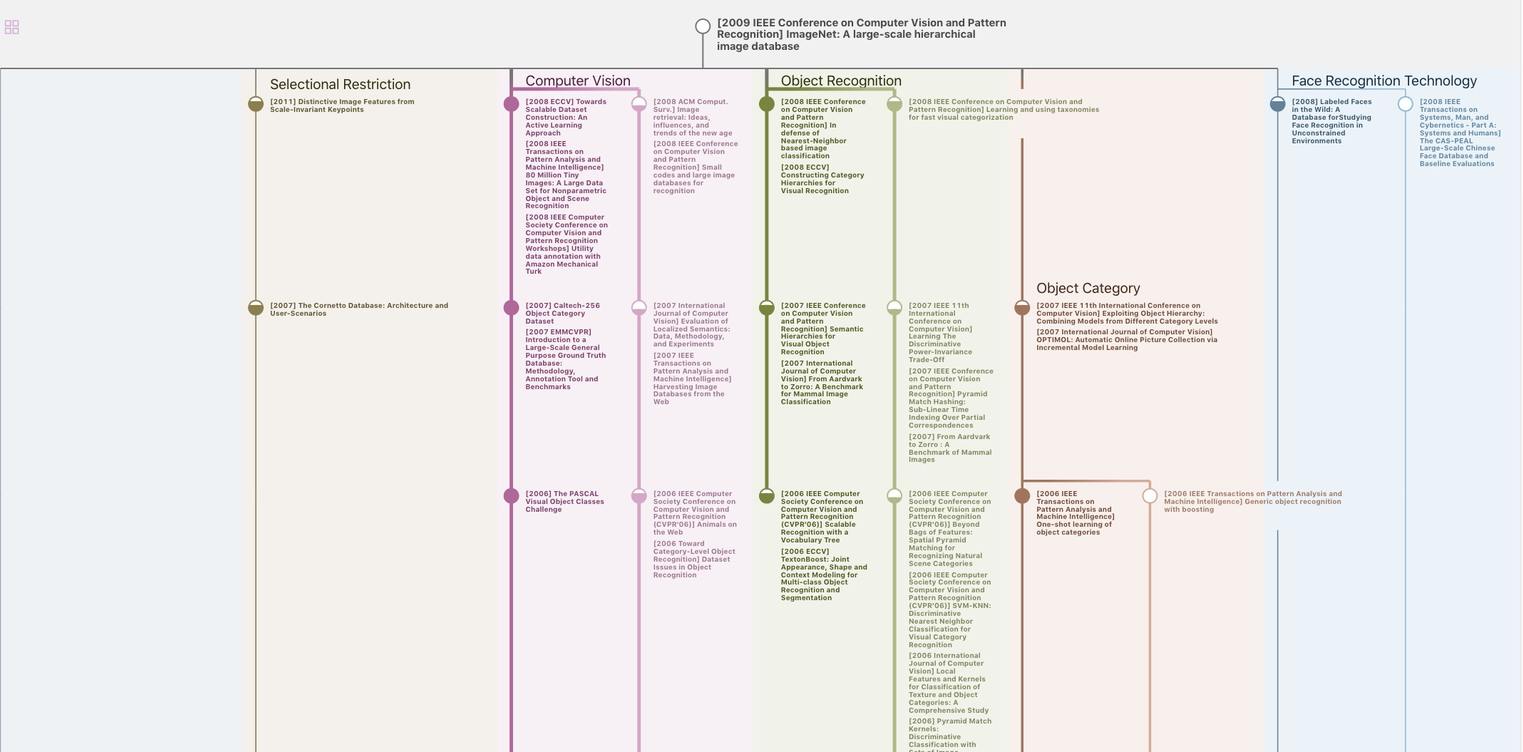
生成溯源树,研究论文发展脉络
Chat Paper
正在生成论文摘要