A unified neural model for review-based rating prediction by leveraging multi-criteria ratings and review text
Cluster Computing(2018)
摘要
The problem of personalized review-based rating prediction aims at inferring users’ ratings over their unrated items using historical ratings and reviews. Most of existing methods solve this problem by integrating latent factor model and topic model to learn interpretable user and items factors. However, these methods ignore the word order in reviews and the learned topic factors are limited to review text, which cannot fully reveal the complicated interaction relations between reviews and ratings. Moreover, they only utilize overall ratings instead of multi-criteria ratings which can represent more detailed preferences of users. In this paper, we propose a deep learning framework named NRPMT in which multi-criteria ratings and user reviews can complement each other to improve recommendation accuracy. The proposed model can simultaneously predict accurate ratings and generate review content expressing user experience and feelings. For rating prediction, a neural factorization machines-based regression model are used to project the feature interactions between user, item and criteria into rating. For review generation, gated recurrent neural networks are employed to “translate” the feature representation of user, item and criteria into a review. Extensive experiments on three real-world datasets demonstrate that NRPMT achieves significant improvement over the several competitive baselines.
更多查看译文
关键词
Review rating prediction,Multi-criteria ratings,Review generation,Deep learning
AI 理解论文
溯源树
样例
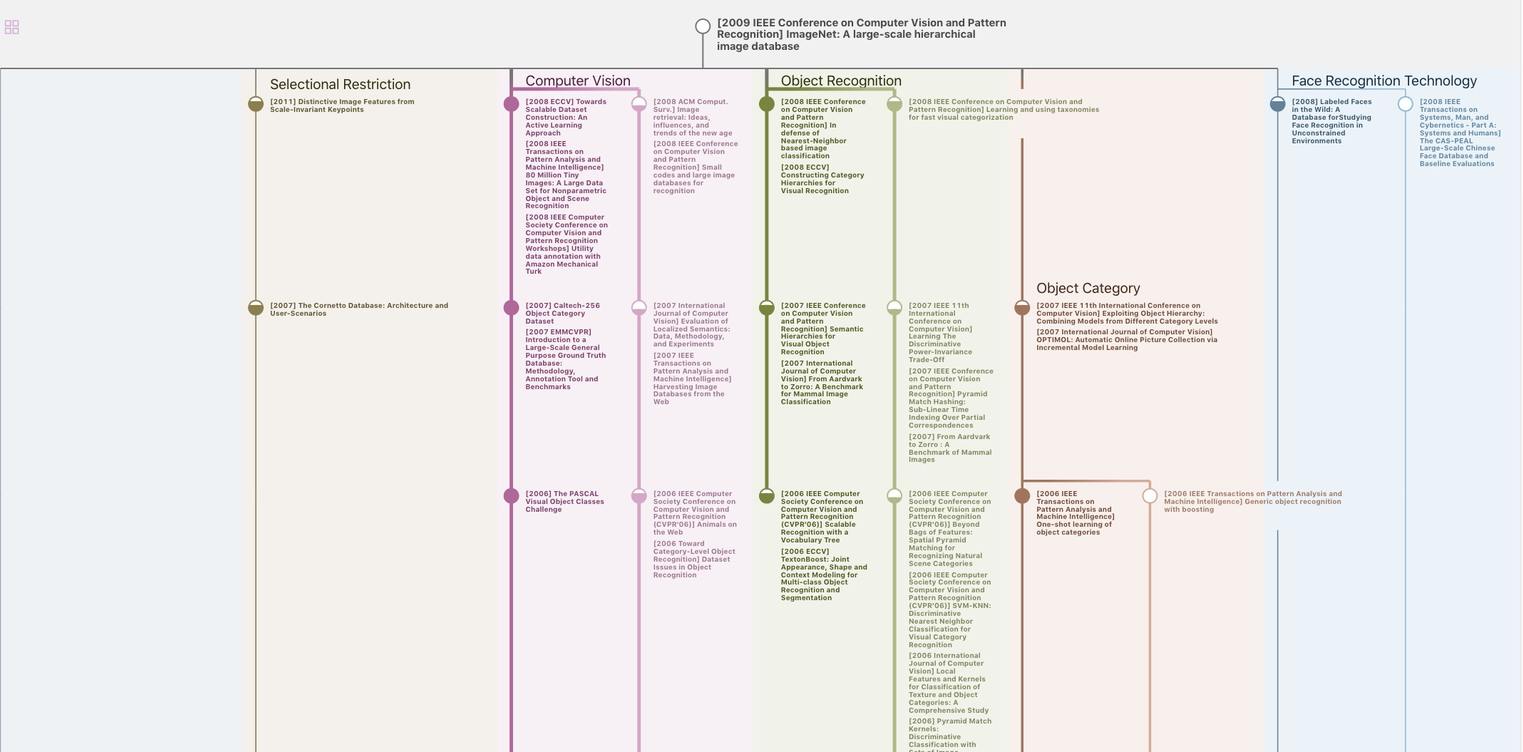
生成溯源树,研究论文发展脉络
Chat Paper
正在生成论文摘要