Towards improving the convolutional neural networks for deep learning using the distributed artificial bee colony method
International Journal of Machine Learning and Cybernetics(2018)
摘要
During the past decade, the dramatic increase in the computational capabilities of chip processing and the lower costs of computing hardware have led to the emergence of deep learning, which refers to a sub-field of machine learning that focuses on learning features extracted from data and classifying them through multiple layers in the hierarchical architectures of neural networks. Using convolution neural networks (CNN) is one of the most promising deep learning methods for dealing with several pattern recognition tasks. However, as with most artificial neural networks, CNNs are susceptible to multiple local optima. Hence, in order to avoid becoming trapped within the local optima, improvement of the CNNs is thus required. The optimization methods based on a metaheuristic are very powerful in solving optimization problems. However, research on the use of metaheuristics to optimize CNNs is rarely conducted. In this work, the artificial bee colony (ABC) method, one of the most popular metaheuristic methods, is proposed as an alternative approach to optimizing the performance of a CNN. In other words, we aim to minimize the classification errors by initializing the weights of the CNN classifier based on solutions generated by the ABC method. Moreover, the distributed ABC is also presented as a method to maintain the amount of time needed to execute the process when working with large training datasets. The results of the experiment demonstrate that the proposed method can improve the performance of the ordinary CNNs in both recognition accuracy and computing time.
更多查看译文
关键词
Deep learning,Convolution neural networks,Distributed artificial bee colony,Pattern recognition,Classification
AI 理解论文
溯源树
样例
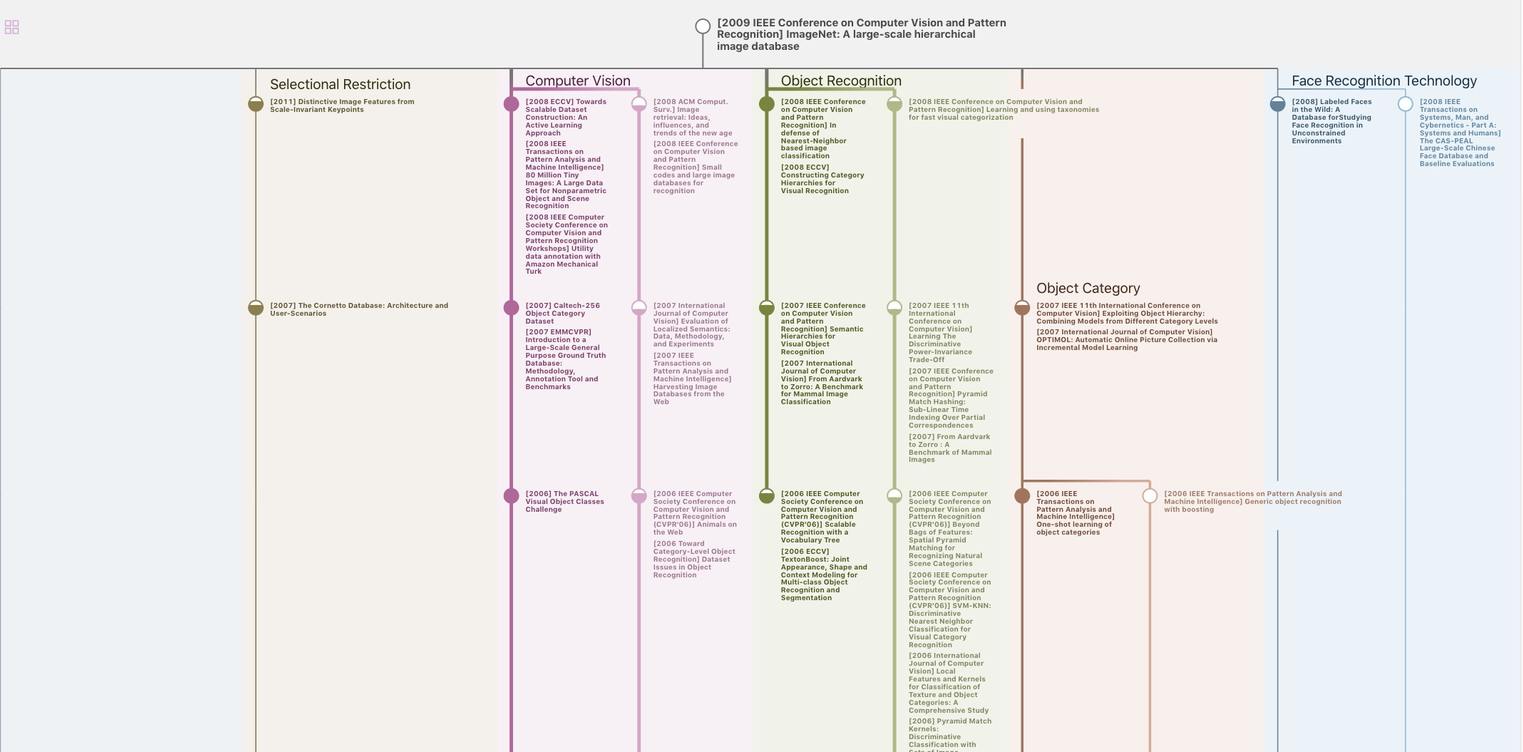
生成溯源树,研究论文发展脉络
Chat Paper
正在生成论文摘要