Feeding the Multitude: A Polynomial-time Algorithm to Improve Sampling
PHYSICAL REVIEW E(2019)
摘要
A wide variety of optimization techniques, both exact and heuristic, tend to be biased samplers. This means that when attempting to find multiple uncorrelated solutions of a degenerate Boolean optimization problem a subset of the solution space tends to be favored while, in the worst case, some solutions can never be accessed by the algorithm used. Here we present a simple postprocessing technique that improves sampling for any optimization approach, either quantum or classical. More precisely, starting from a pool of a few optimal configurations, the algorithm generates potentially new solutions via rejection-free cluster updates at zero temperature. Although the method is not ergodic and there is no guarantee that all the solutions can be found, fair sampling is typically improved. We illustrate the effectiveness of our method by improving the exponentially biased data produced by the D-Wave 2X quantum annealer [S. Mandra et al., Phys. Rev. Lett. 118, 070502 (2017)], as well as data from three-dimensional Ising spin glasses. As part of the study, we also show that sampling is improved when suboptimal states are included and discuss sampling at a finite fixed temperature.
更多查看译文
AI 理解论文
溯源树
样例
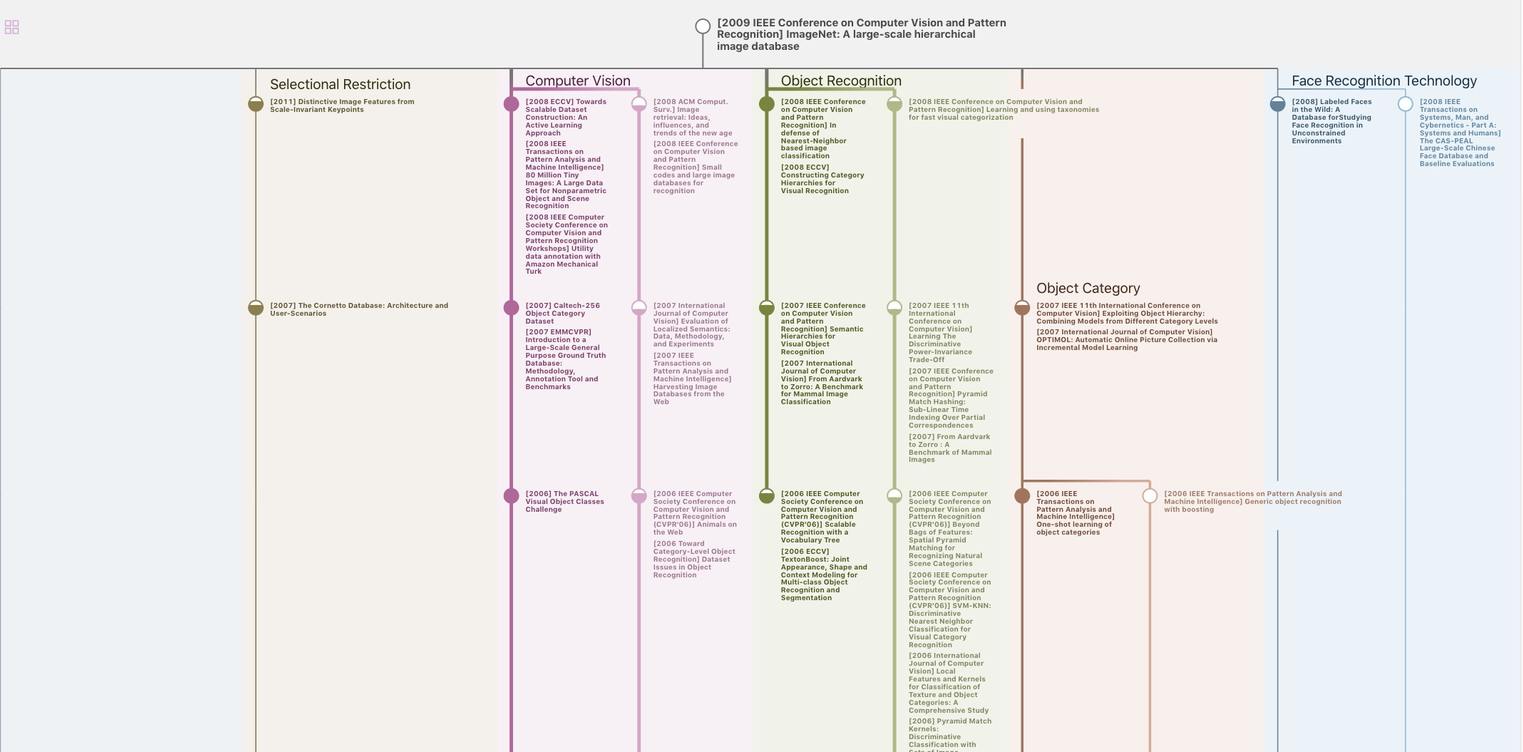
生成溯源树,研究论文发展脉络
Chat Paper
正在生成论文摘要