Optimizing Timeliness and Cost in Geo-Distributed Streaming Analytics
IEEE Transactions on Cloud Computing(2020)
摘要
Rapid data streams are generated continuously from diverse sources including users, devices, and sensors located around the globe. This results in the need for efficient geo-distributed streaming analytics to extract timely information. A typical geo-distributed analytics service uses a hub-and-spoke model, comprising multiple edges connected by a wide-area-network (WAN) to a central data warehouse. In this paper, we focus on the widely used primitive of
windowed grouped aggregation
, and examine the question of
how much computation should be performed at the edges versus the center
. We develop algorithms to optimize two key metrics:
WAN traffic
and
staleness
(delay in getting results). We present a family of optimal offline algorithms that
jointly minimize
these metrics, and we use these to guide our design of practical online algorithms based on the insight that windowed grouped aggregation can be modeled as a
caching
problem where the cache size varies over time. We evaluate our algorithms through an implementation in Apache Storm deployed on PlanetLab. Using workloads derived from anonymized traces of a popular analytics service from a large commercial CDN, our experiments show that our online algorithms achieve near-optimal traffic and staleness for a variety of system configurations, stream arrival rates, and queries.
更多查看译文
关键词
Algorithm design and analysis,Wide area networks,Delays,Bandwidth,Aggregates
AI 理解论文
溯源树
样例
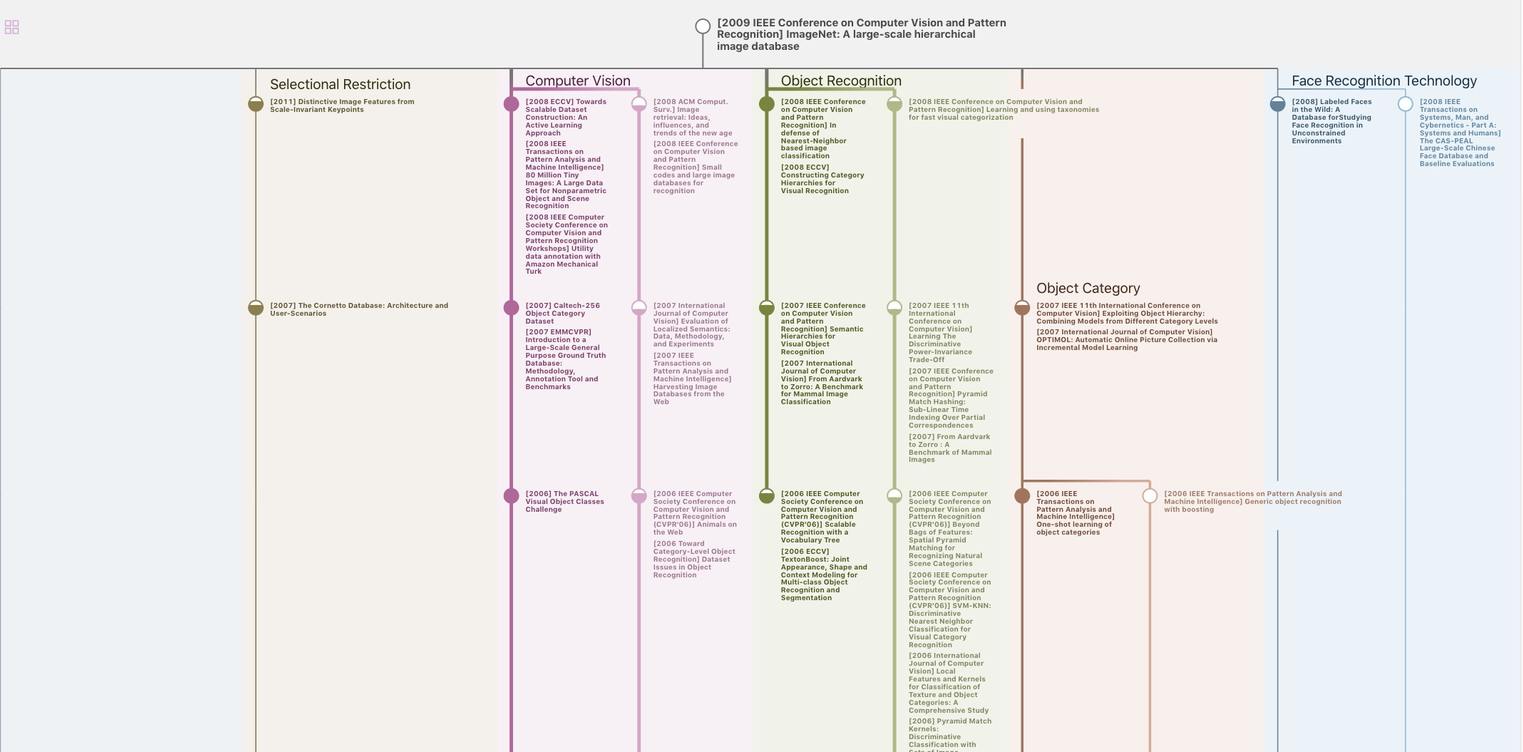
生成溯源树,研究论文发展脉络
Chat Paper
正在生成论文摘要