Using Deep Learning To Bridge The Gap Between Perception And Intelligence
UNMANNED SYSTEMS TECHNOLOGY XIX(2017)
摘要
We propose a method to for robot planning using deep learning to integrate object detection and natural language understanding. This is different from other techniques, such the RCTA World Model, which explicitly defines the interfaces between each module. These boundaries simplify the design and testing of complex robotic systems, but also introduce constraints that may reduce overall system performance. For example, perception tasks generate large amounts of data, but much of it is discarded to simplify interpretation by higher level tasks, e.g., a 3D object becomes a point in space, or a distribution over classifications is reduced to its mode. Further, errors in the robot's overal task are not back-propagated to lower level tasks, and therefore, these tasks never adapt themselves to improve robot performance. We intend to address this by using a deep learning framework to replace these interfaces with learned interfaces that select what data is shared between modules and allow for error back-propagation that could adapt each module to the robot's task. We will do this in a simplified system that accepts aerial orthographic images and simple commands and generates paths to achieve the command. Paths are learned from expert example via inverse optimal control. In time, we hope to evolve this simplified architecture towards something more complex and practical.
更多查看译文
关键词
Robotics Collaborative Technology Alliance, Robotics, Unmanned Ground Vehicles, Deep Learning, Perception, Planning
AI 理解论文
溯源树
样例
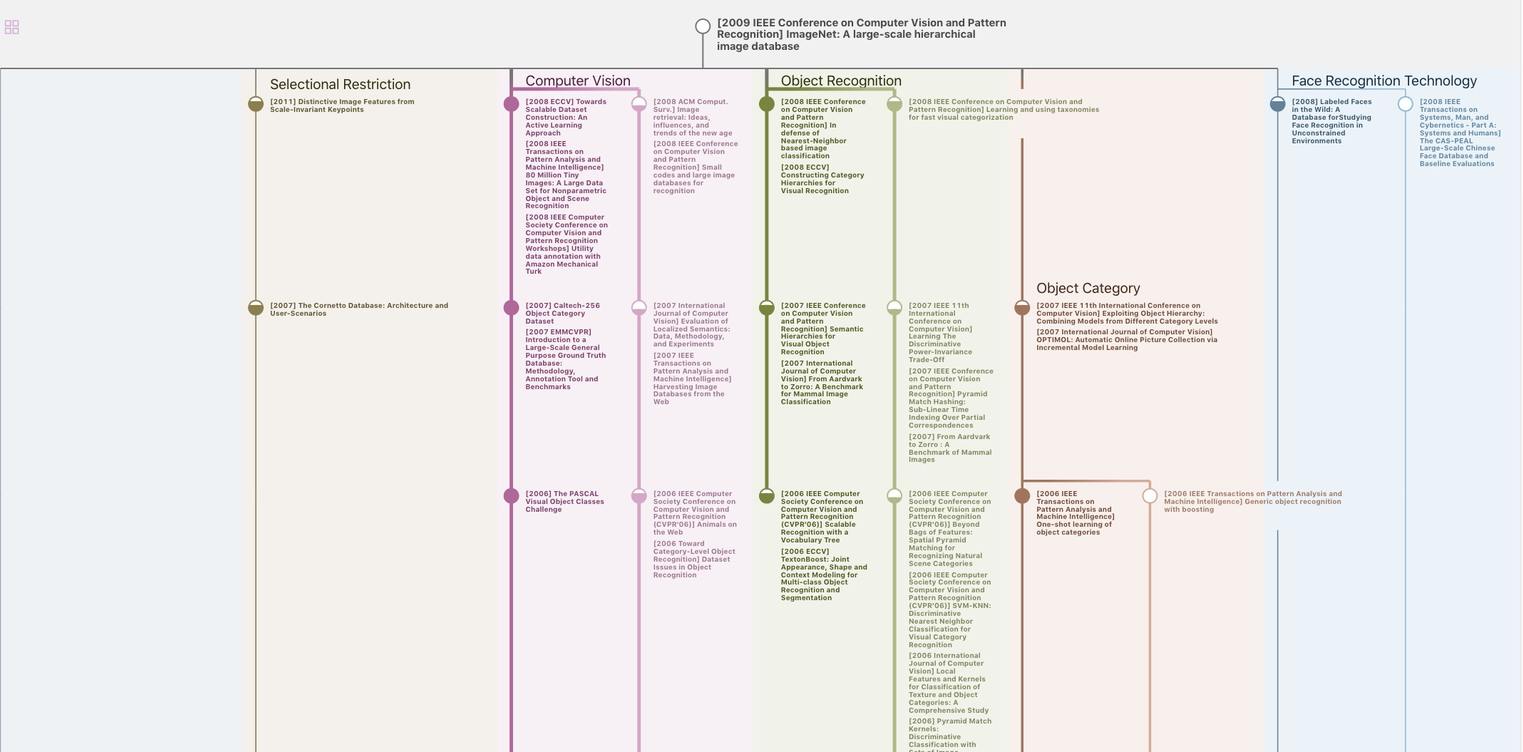
生成溯源树,研究论文发展脉络
Chat Paper
正在生成论文摘要