Yeast viability and concentration analysis using lens-free computational microscopy and machine learning
Proceedings of SPIE(2017)
摘要
Research laboratories and the industry rely on yeast viability and concentration measurements to adjust fermentation parameters such as pH, temperature, and pressure. Beer-brewing processes as well as biofuel production can especially utilize a cost-effective and portable way of obtaining data on cell viability and concentration. However, current methods of analysis are relatively costly and tedious. Here, we demonstrate a rapid, portable, and cost-effective platform for imaging and measuring viability and concentration of yeast cells. Our platform features a lens-free microscope that weighs 70 g and has dimensions of 12 x 4 x 4 cm. A partially-coherent illumination source (a light-emitting-diode), a band-pass optical filter, and a multimode optical fiber are used to illuminate the sample. The yeast sample is directly placed on a complementary metal-oxide semiconductor (CMOS) image sensor chip, which captures an in-line hologram of the sample over a large field-of-view of >20 mm(2). The hologram is transferred to a touch-screen interface, where a trained Support Vector Machine model classifies yeast cells stained with methylene blue as live or dead and measures cell viability as well as concentration. We tested the accuracy of our platform against manual counting of live and dead cells using fluorescent exclusion staining and a bench-top fluorescence microscope. Our regression analysis showed no significant difference between the two methods within a concentration range of 1.4 x 105 to 1.4 x 10(6) cells/mL. This compact and cost-effective yeast analysis platform will enable automatic quantification of yeast viability and concentration in field settings and resource-limited environments.
更多查看译文
关键词
Lens-free microscopy,lensless imaging,on-chip imaging,yeast viability testing,machine learning,computational imaging
AI 理解论文
溯源树
样例
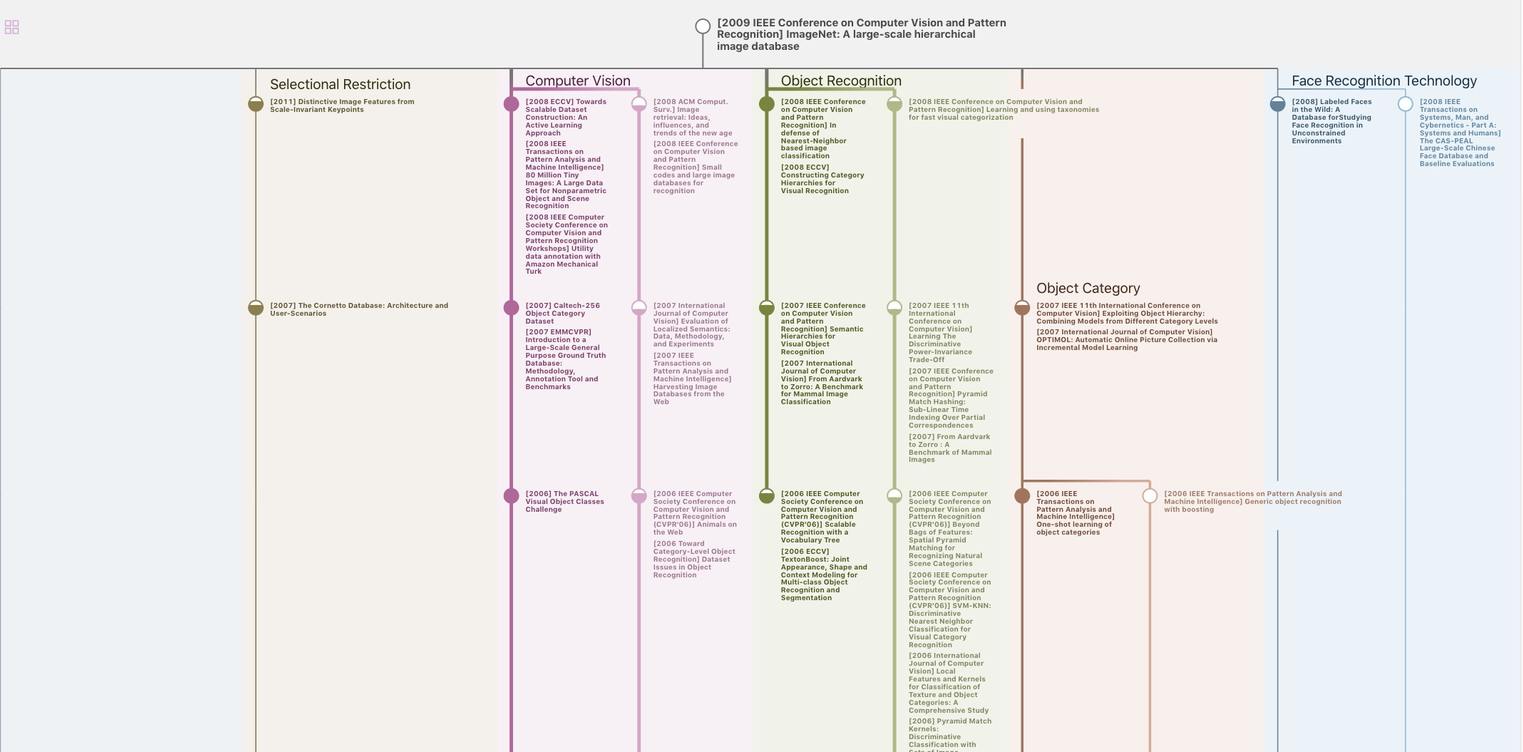
生成溯源树,研究论文发展脉络
Chat Paper
正在生成论文摘要