Gene Regulatory Network Inference Using Time-Stamped Cross-Sectional Single Cell Expression Data
IFAC-PapersOnLine(2016)
摘要
In this paper we presented a novel method for inferring gene regulatory network (GRN) from time-stamped cross-sectional single cell data. Our strategy, called SNIFS (Sparse Network Inference For Single cell data) seeks to recover the causal relationships among genes by analyzing the evolution of the distribution of gene expression levels over time, more specifically using Kolmogorov-Smirnov (KS) distance. In the proposed method, we formulated the GRN inference as a linear regression problem, where we used Lasso regularization to obtain the optimal sparse solution. We tested SNIFS using in silico single cell data from 10 - and 20-gene GRNs, and compared the performance of our method with Time Series Network Inference (TSNI), GEne Network Inference with Ensemble of trees (GENIE3), and an extension of GENIE3 for time series data called JUMP3. The results showed that SNIFS outperformed existing algorithms based on the Area Under the Receiver Operating Characteristic (AUROC) and Area Under the Precision-Recall (AUPR) curves.
更多查看译文
关键词
network inference,single cell,gene expression,gene regulatory network
AI 理解论文
溯源树
样例
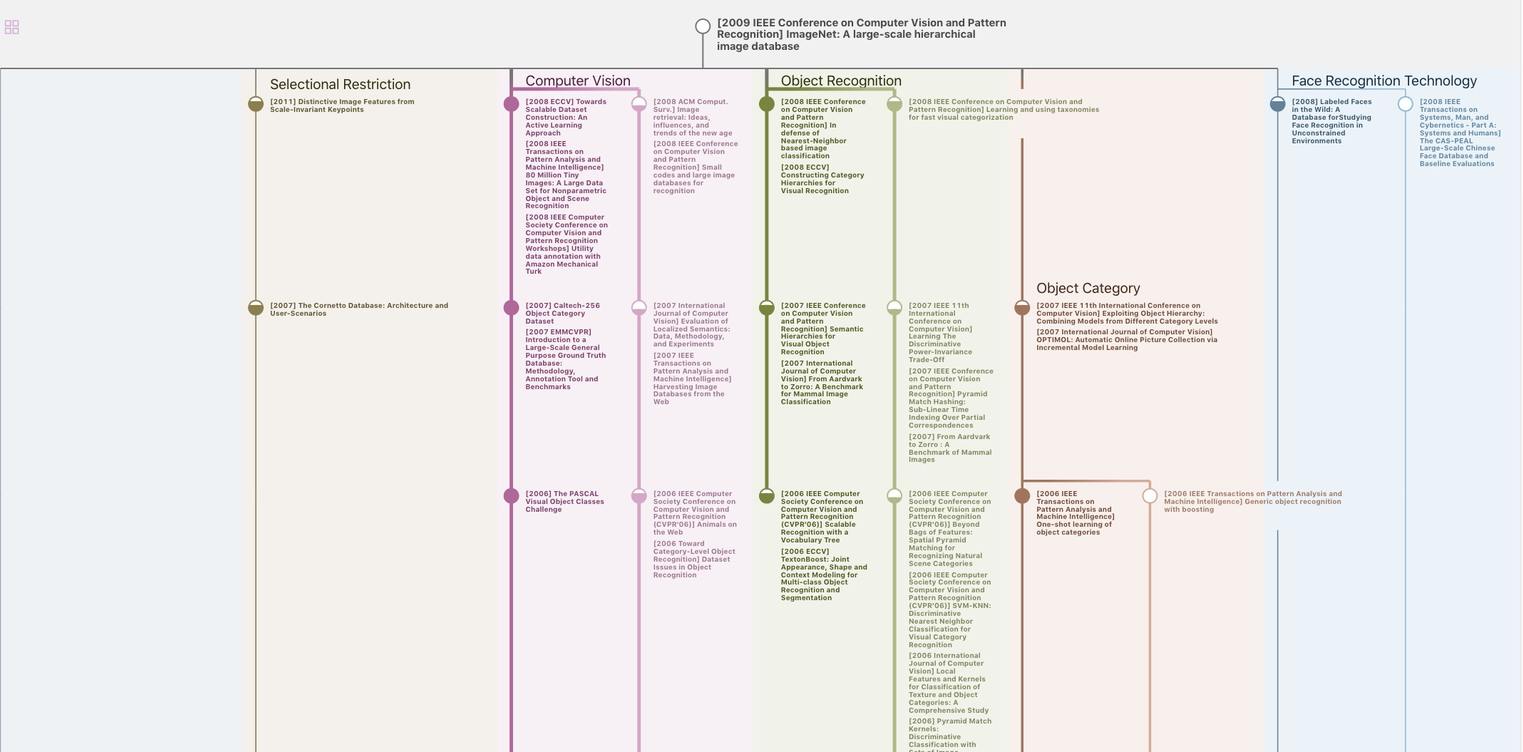
生成溯源树,研究论文发展脉络
Chat Paper
正在生成论文摘要