Optimal adaptive estimation of linear functionals under sparsity
ANNALS OF STATISTICS(2018)
摘要
We consider the problem of estimation of a linear functional in the Gaussian sequence model where the unknown vector theta is an element of R-d belongs to a class of s-sparse vectors with unknown s. We suggest an adaptive estimator achieving a nonasymptotic rate of convergence that differs from the minimax rate at most by a logarithmic factor. We also show that this optimal adaptive rate cannot be improved when s is unknown. Furthermore, we address the issue of simultaneous adaptation to s and to the variance sigma(2) of the noise. We suggest an estimator that achieves the optimal adaptive rate when both s and sigma(2) are unknown.
更多查看译文
关键词
Nonasymptotic minimax estimation,adaptive estimation,linear functional,sparsity,unknown noise variance
AI 理解论文
溯源树
样例
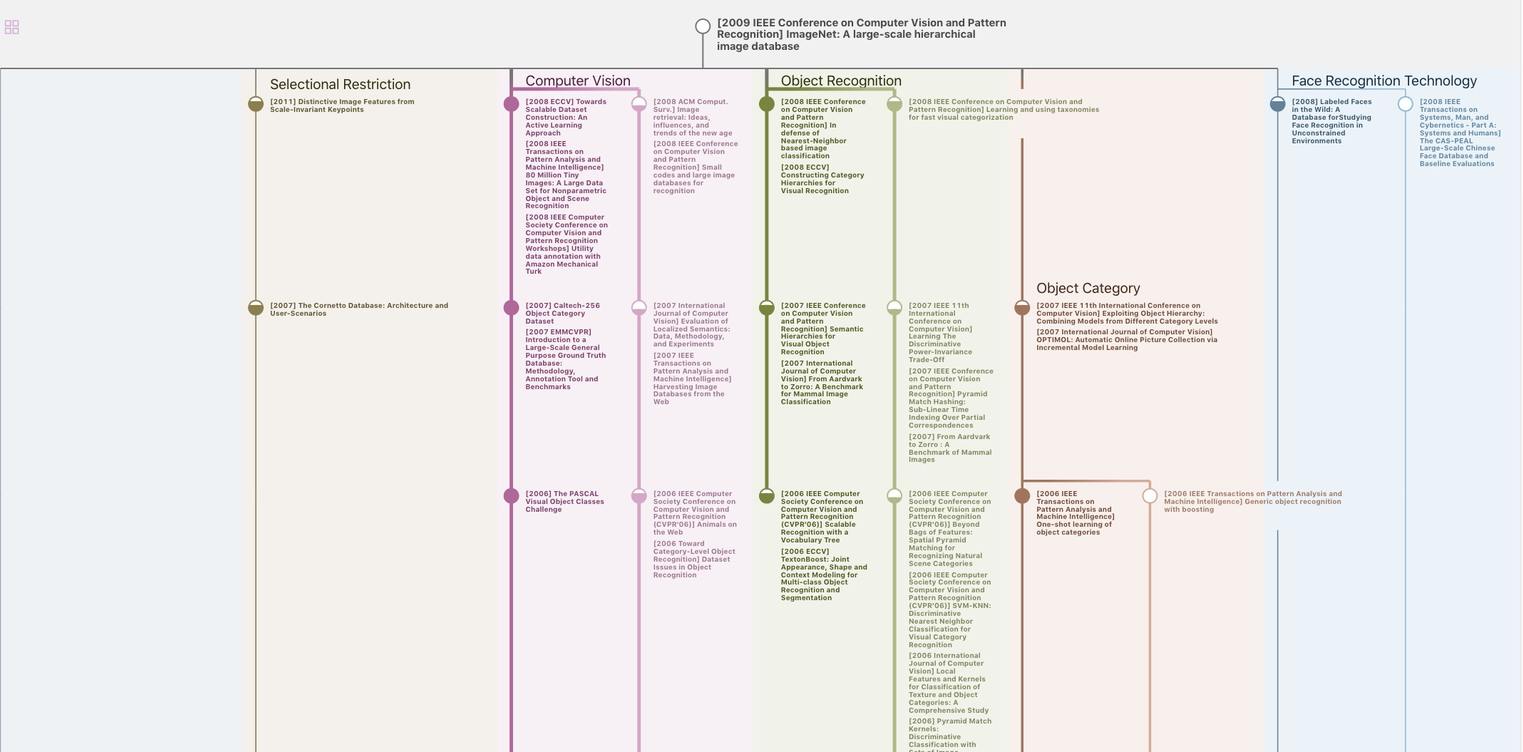
生成溯源树,研究论文发展脉络
Chat Paper
正在生成论文摘要