Predicting future monthly residential energy consumption using building characteristics and climate data: A statistical learning approach
Energy and Buildings(2016)
摘要
Abstract In this paper a large-scale study is presented that applies statistical learning methods to predict future monthly energy consumption for single-family detached homes using building attributes and monthly climate data. Building data is collected from over 426,305 homes in Bexar County, TX with four years of monthly energy consumption (natural gas and electricity). The goal of this study is to establish reliable models for forecasting residential energy consumption, understand the predictive value of building attributes, identify differences in predictability between households, and measure the robustness in model performance given uncertainty in climate forecasts. Assuming accurate climate forecasts, results show future monthly energy consumption can reasonably be predicted for out-of-sample households, with 74% accuracy at the household level and over 90% accuracy for predicting aggregate monthly energy usage. However, model performance is significantly different between households with distinct fuel types. Using historical climate forecast, results also demonstrate that model predictability significantly decays at both the household and aggregate level, but is robust at the household level when measured by the median home. Model selection and variable importance plots illustrate several building characteristics significantly contribute to predicting monthly energy consumption while most provide marginal predictive value.
更多查看译文
关键词
Forecasting energy,Multivariate adaptive regression splines,Regression trees,Model validation
AI 理解论文
溯源树
样例
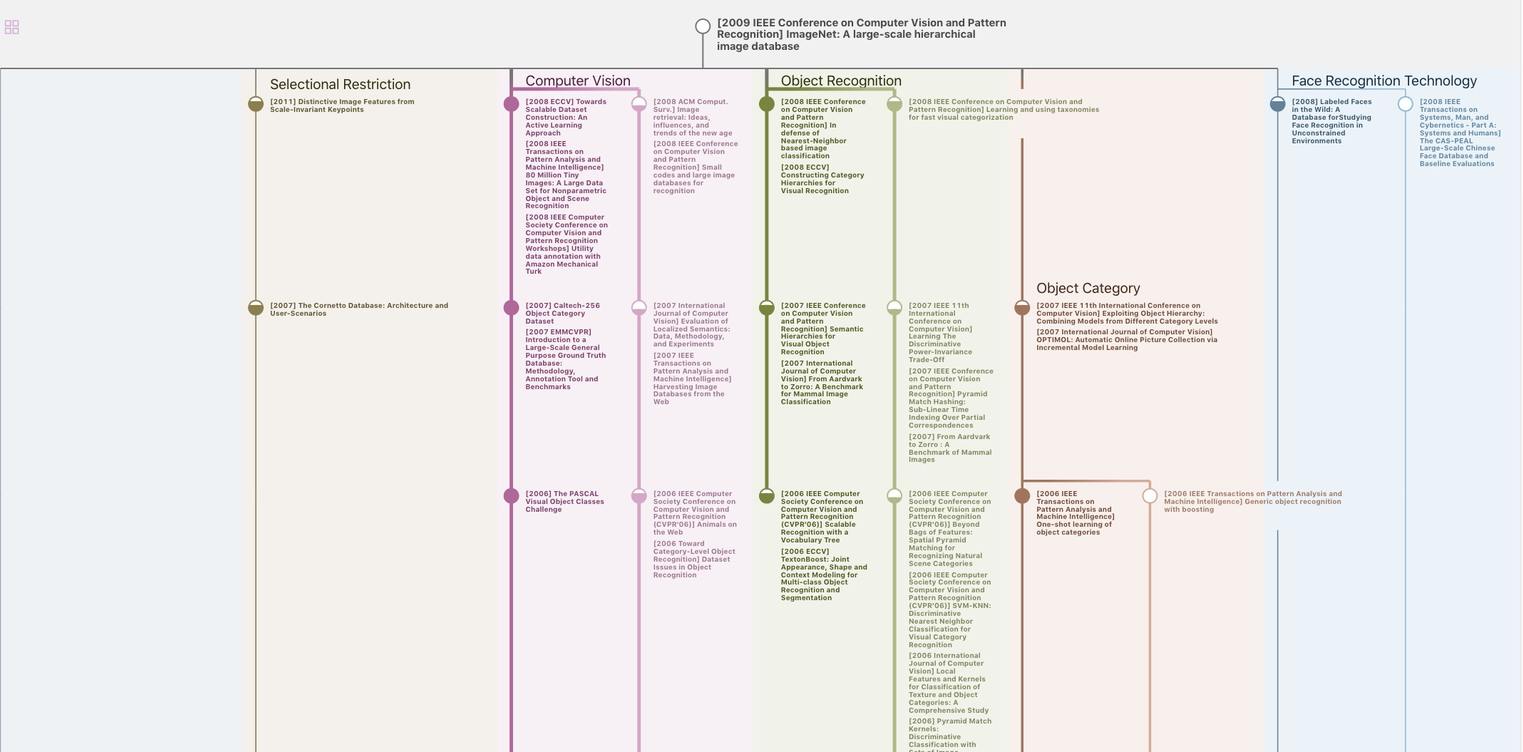
生成溯源树,研究论文发展脉络
Chat Paper
正在生成论文摘要