Agent-based modeling in electrical energy markets using dynamic Bayesian networks
IEEE Transactions on Power Systems(2017)
摘要
Due to uncertainties in generation and load, optimal decision making in electrical energy markets is a complicated and challenging task. Participating agents in the market have to estimate optimal bidding strategies based on incomplete public information and private assessment of the future state of the market, to maximize their expected profit at different time scales. In this paper, we present an agent-based model to address the problem of short-term strategic bidding of conventional generation companies (GenCos) in a power pool. Based on the proposed model, each GenCo agent develops a private probabilistic model of the market (using dynamic Bayesian networks), employs an online learning algorithm to train the model (sparse Bayesian learning), and infers the future state of the market to estimate the optimal bidding function. We show that by using this multiagent framework, the agents will be able to predict and adapt to approximate Nash equilibrium of the market through time using local reasoning and incomplete publicly available data. The model is implemented in MATLAB and is tested on four test case systems: two generic systems with 5 and 15 GenCo agents, and two IEEE benchmarks (9-bus and 30-bus systems). Both the day-ahead (DA) and hour-ahead (HA) bidding schemes are implemented.
更多查看译文
关键词
Decision making,Bayes methods,Uncertainty,Mathematical model,Adaptation models,Programming,Load modeling
AI 理解论文
溯源树
样例
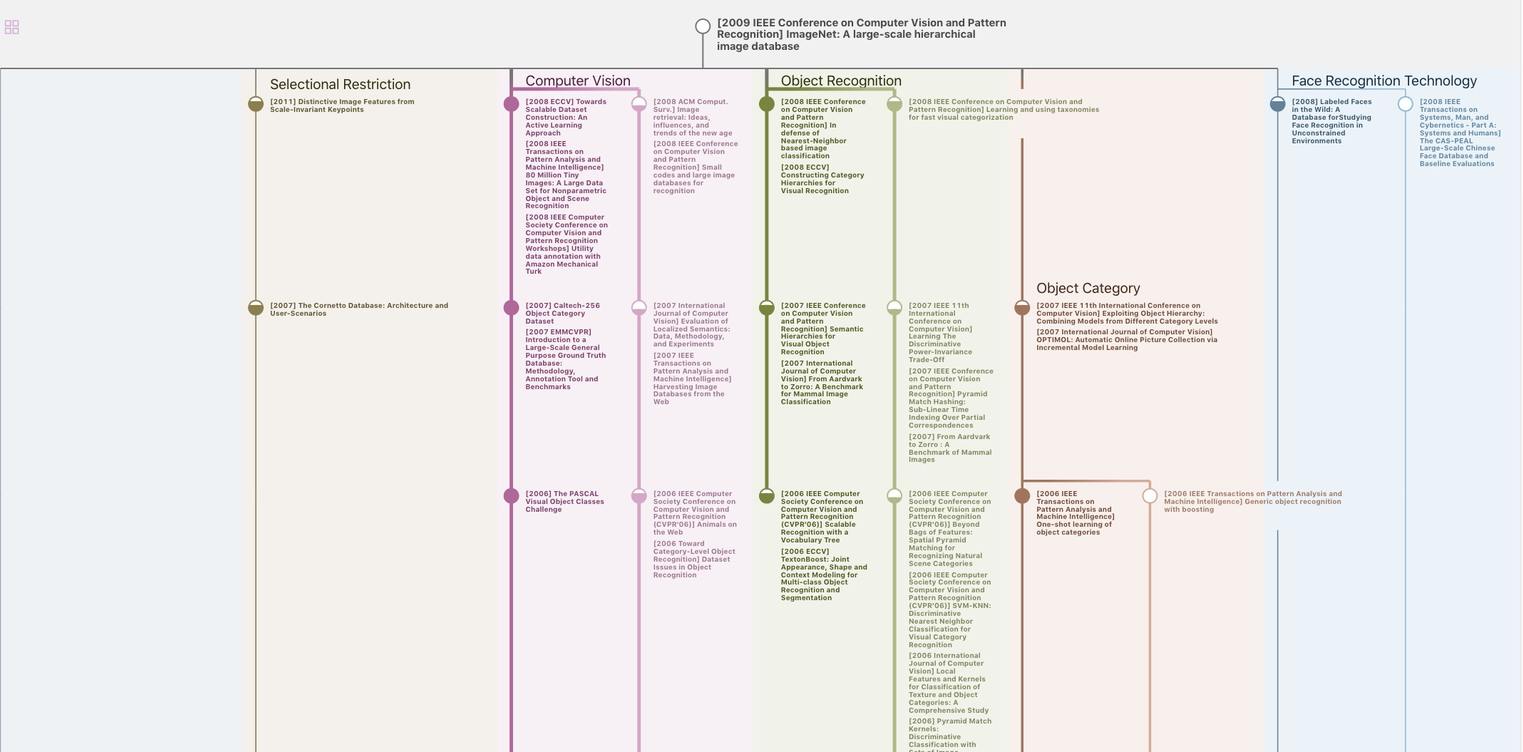
生成溯源树,研究论文发展脉络
Chat Paper
正在生成论文摘要