Memristive Accelerators for Dense and Sparse Linear Algebra: From Machine Learning to High-Performance Scientific Computing
IEEE Micro(2019)
摘要
Initial research shows that in situ accelerators can be architected to meet the requirements of applications beyond machine learning while still preserving the performance and energy benefits of in situ acceleration. However, challenges remain to improve the efficiency of these techniques. Although memristive accelerators significantly reduce data movement, coordinating computation, and marshaling data within a large accelerator, or between systems of multiple accelerators, remains largely unexplored. Additionally, many proposed in situ accelerators rely on the matrix being written infrequently such that writes can be amortized over a large number of MVM operations, limiting the applicability to workloads with infrequent matrix updates. If these challenges are addressed, in situ memristive computation can provide benefits to an enormous number of applications with linear algebra at their core.
更多查看译文
关键词
memristive accelerators,sparse linear algebra,high-performance
AI 理解论文
溯源树
样例
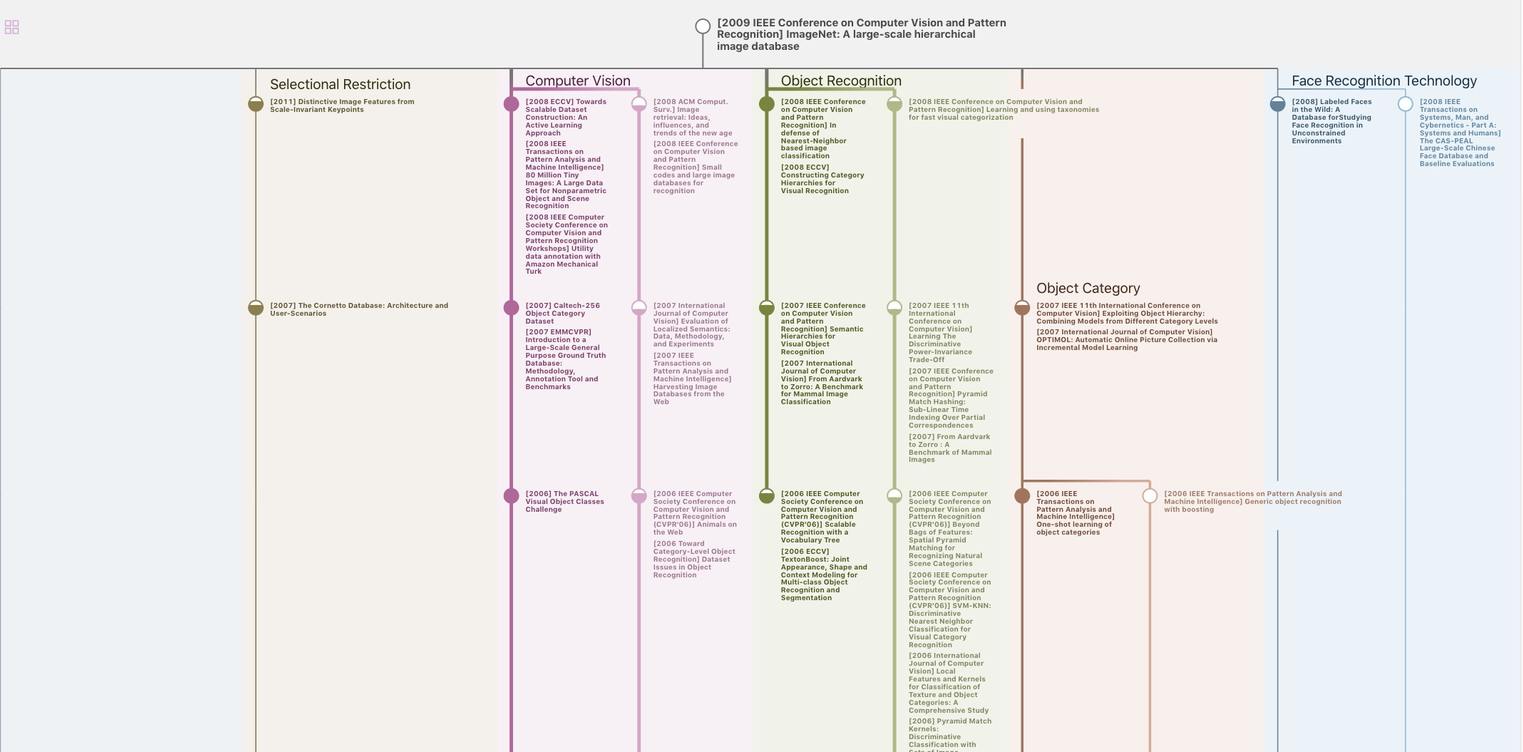
生成溯源树,研究论文发展脉络
Chat Paper
正在生成论文摘要