Topic Discovery via Convex Polytopic Model: A Case Study with Small Corpora
2018 9th IEEE International Conference on Cognitive Infocommunications (CogInfoCom)(2018)
摘要
Topic discovery is an important problem in text processing. Topic modeling approaches such as latent Dirichlet allocation (LDA) has been applied quite successfully in extracting topics. However, there still exists several directions for further improvement. Short texts (e.g. tweets and news titles) present the problem of data sparsity for LDA. Second, there needs to be greater transparency in the process of topic discovery in order to enhance interpretability for humans. Third, the robustness of the model needs to be further enhanced to avoid sensitivity to the choice of hyper-parameters. In this paper, we propose a novel geometric approach based on convex polytopic model (CPM) which can discover representative and interpretable topical features from the given corpus. By embedding all documents into a low-dimensional affine subspace, we show that the topics can be obtained geometrically as the vertices of a compact polytope which encloses all the embedded documents. We further interpret the features acquired as topics and use them to obtain a convex polytopic document representation for every document. We studied the properties of CPM by two small corpora of short texts. Results reveal that the proposed CPM can discover interpretable topics even for short texts. We also discover that the geometric nature of CPM enhances model transparency and topic interpretability, as well as robustness to hyper-parameter selection.
更多查看译文
关键词
Feature extraction,Semantics,Hidden Markov models,Robustness,Task analysis,Analytical models,Principal component analysis
AI 理解论文
溯源树
样例
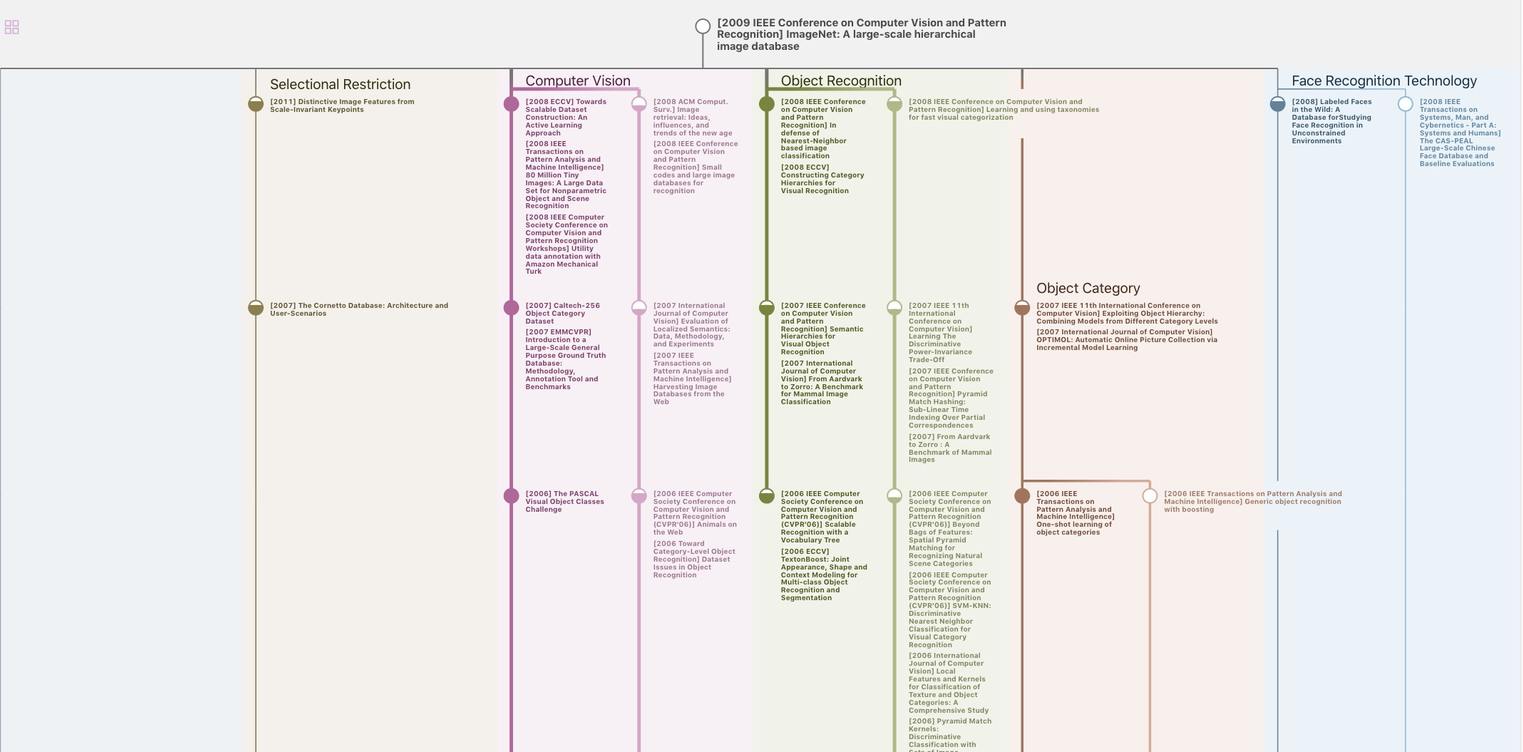
生成溯源树,研究论文发展脉络
Chat Paper
正在生成论文摘要