Online Estimation of Multiple Dynamic Graphs in Pattern Sequences
2019 INTERNATIONAL JOINT CONFERENCE ON NEURAL NETWORKS (IJCNN)(2019)
摘要
Sequences of correlated binary patterns can represent many time-series data including text, movies, and biological signals. These patterns may be described by weighted combinations of a few dominant structures that underpin specific interactions among the binary elements. To extract the dominant correlation structures and their contributions to generating data in a time-dependent manner, we model the dynamics of binary patterns using the state-space model of an Ising-type network that is composed of multiple undirected graphs. We provide a sequential Bayes algorithm to estimate the dynamics of weights on the graphs while gaining the graph structures online. This model can uncover overlapping graphs underlying the data better than a traditional orthogonal decomposition method, and outperforms an original time-dependent Ising model. We assess the performance of the method by simulated data, and demonstrate that spontaneous activity of cultured hippocampal neurons is represented by dynamics of multiple graphs.
更多查看译文
关键词
online estimation,multiple dynamic graphs,pattern sequences,correlated binary patterns,biological signals,binary elements,dominant correlation structures,state-space model,Ising-type network,sequential Bayes algorithm,graph structures,time-series data,undirected graphs,orthogonal decomposition method,time-dependent Ising model,cultured hippocampal neurons
AI 理解论文
溯源树
样例
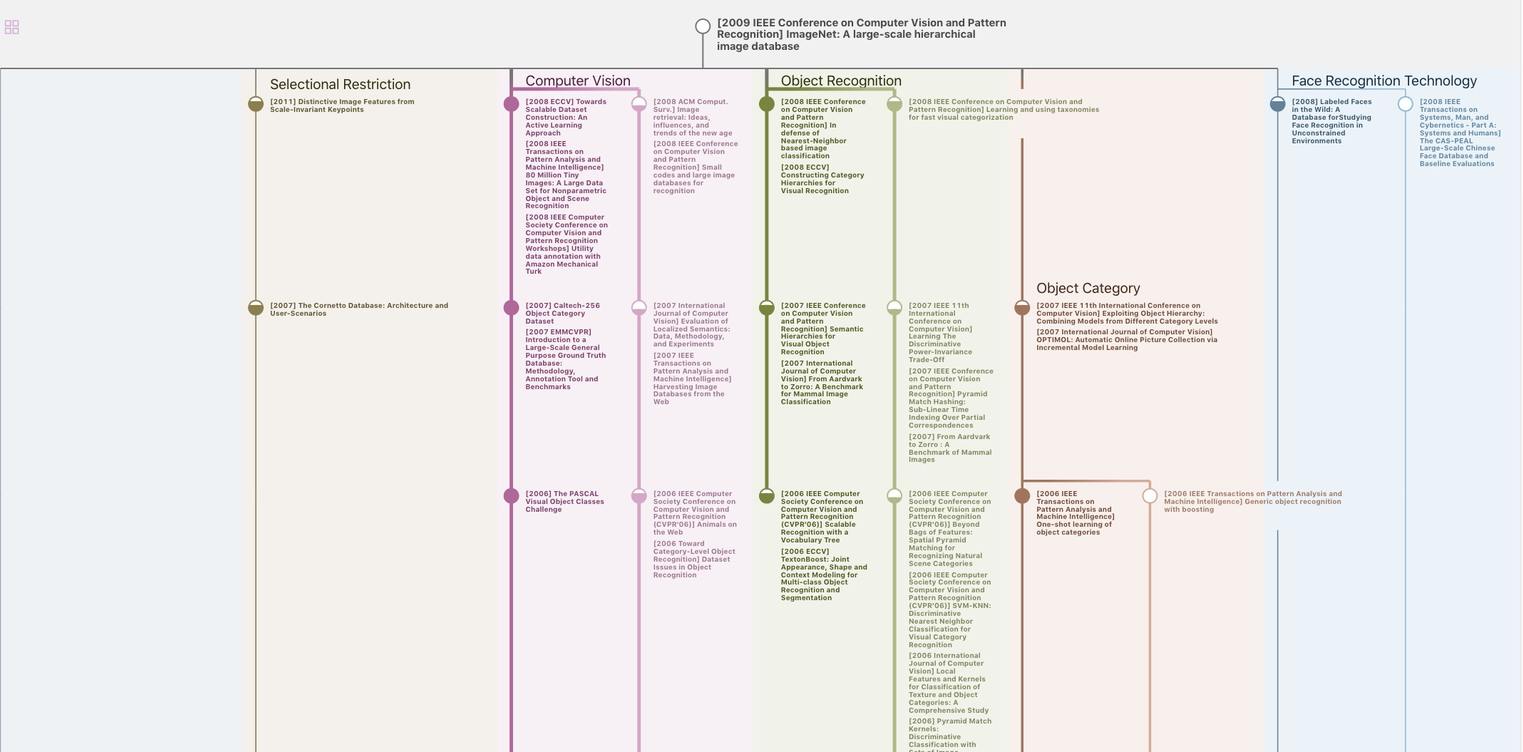
生成溯源树,研究论文发展脉络
Chat Paper
正在生成论文摘要