Split Regularized Regression
arxiv(2019)
摘要
We propose an approach for fitting linear regression models that splits the set of covariates into groups. Essentially, the regression coefficients of the variables in each group are estimated separately from the other groups. The estimated coefficients are then pooled together to form the final fit. The optimal split of the variables into groups and the regularized estimation of the regression coefficients are performed by minimizing an objective function that encourages sparsity within each group and diversity among them. Our procedure works on top of a given penalized linear regression estimator (e.g., Lasso, elastic net) by fitting it to possibly overlapping groups of features, encouraging diversity among these groups to reduce the correlation of the corresponding predictions. For the case of an elastic net penalty and orthogonal predictors, we give a closed form solution for the regression coefficients in each group. We establish the consistency of our method with the number of predictors possibly increasing with the sample size. An extensive simulation study and real-data applications show that in general the proposed method improves the prediction accuracy of the base estimator used in the procedure. Possible extensions to GLMs and other models are discussed.
更多查看译文
关键词
Elastic net,High-dimensional data,Linear regression,Small sample size
AI 理解论文
溯源树
样例
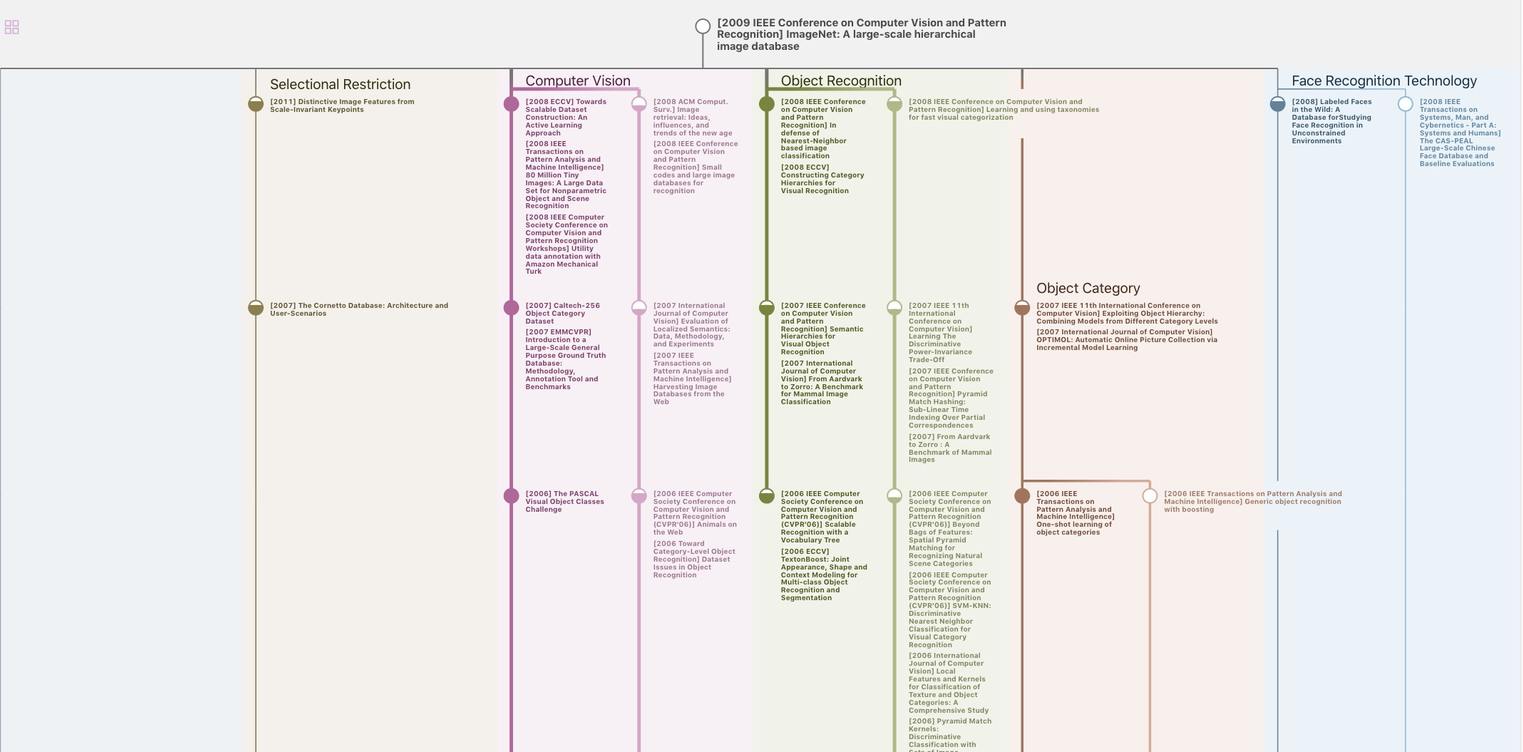
生成溯源树,研究论文发展脉络
Chat Paper
正在生成论文摘要