Dynamic Linear Discriminant Analysis in High Dimensional Space
BERNOULLI(2019)
摘要
High-dimensional data that evolve dynamically feature predominantly in the modern data era. As a partial response to this, recent years have seen increasing emphasis to address the dimensionality challenge. However, the non-static nature of these datasets is largely ignored. This paper addresses both challenges by proposing a novel yet simple dynamic linear programming discriminant (DLPD) rule for binary classification. Different from the usual static linear discriminant analysis, the new method is able to capture the changing distributions of the underlying populations by modeling their means and covariances as smooth functions of covariates of interest. Under an approximate sparse condition, we show that the conditional misclassification rate of the DLPD rule converges to the Bayes risk in probability uniformly over the range of the variables used for modeling the dynamics, when the dimensionality is allowed to grow exponentially with the sample size. The minimax lower bound of the estimation of the Bayes risk is also established, implying that the misclassification rate of our proposed rule is minimax-rate optimal. The promising performance of the DLPD rule is illustrated via extensive simulation studies and the analysis of a breast cancer dataset.
更多查看译文
关键词
Bayes rule, discriminant analysis, dynamic linear programming, high-dimensional data, kernel estimation, sparsity
AI 理解论文
溯源树
样例
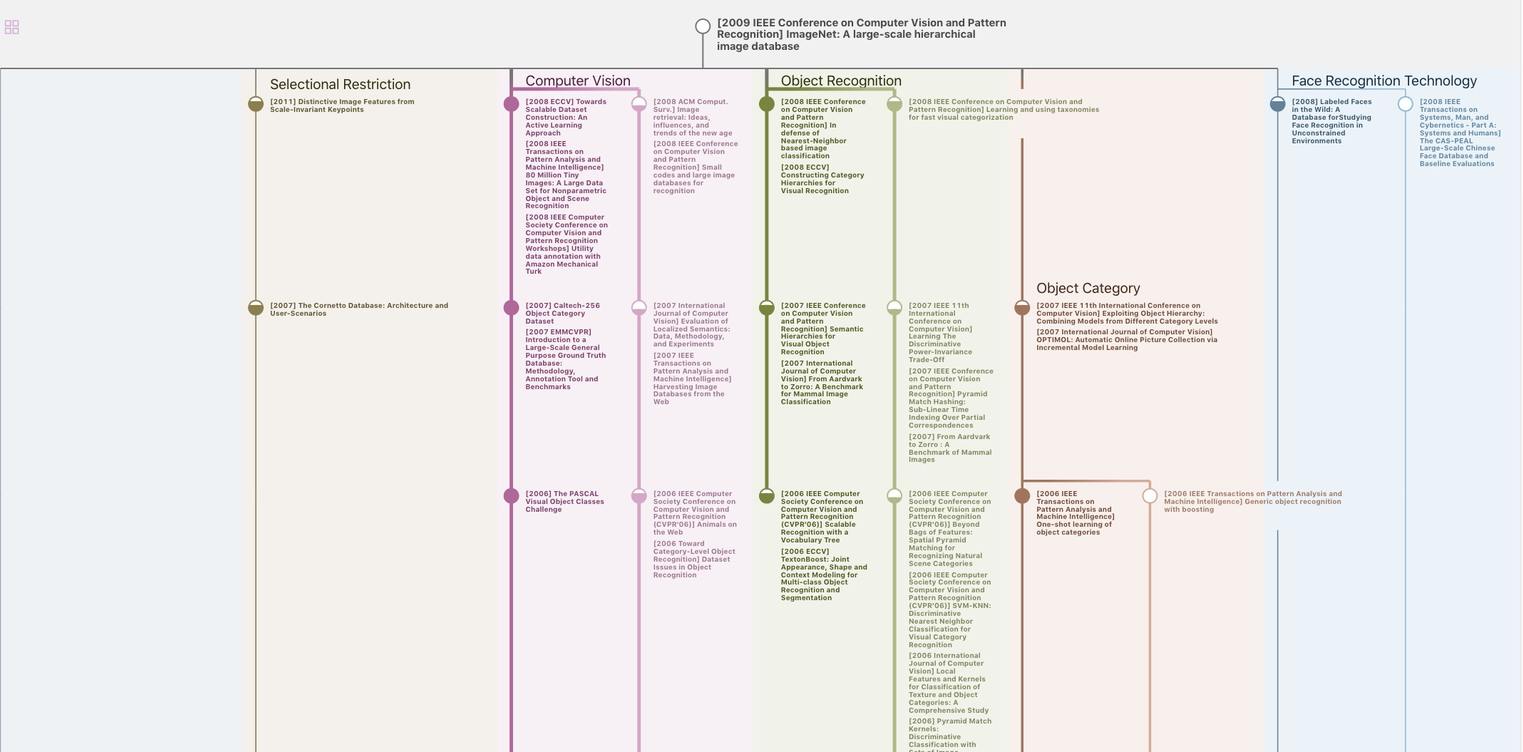
生成溯源树,研究论文发展脉络
Chat Paper
正在生成论文摘要