Iterative Relevance Feedback for Answer Passage Retrieval with Passage-level Semantic Match
arXiv: Information Retrieval(2018)
摘要
Relevance feedback techniques assume that users provide relevance judgments for the top k (usually 10) documents and then re-rank using a new query model based on those judgments. Even though this is effective, there has been little research recently on this topic because requiring users to provide substantial feedback on a result list is impractical in a typical web search scenario. In new environments such as voice-based search with smart home devices, however, feedback about result quality can potentially be obtained during users' interactions with the system. Since there are severe limitations on the length and number of results that can be presented in a single interaction in this environment, the focus should move from browsing result lists to iterative retrieval and from retrieving documents to retrieving answers. In this paper, we study iterative relevance feedback techniques with a focus on retrieving answer passages. We first show that iterative feedback is more effective than the top-k approach for answer retrieval. Then we propose an iterative feedback model based on passage-level semantic match and show that it can produce significant improvements compared to both word-based iterative feedback models and those based on term-level semantic similarity.
更多查看译文
关键词
Iterative relevance feedback, Answer passage retrieval, Passage embeddings
AI 理解论文
溯源树
样例
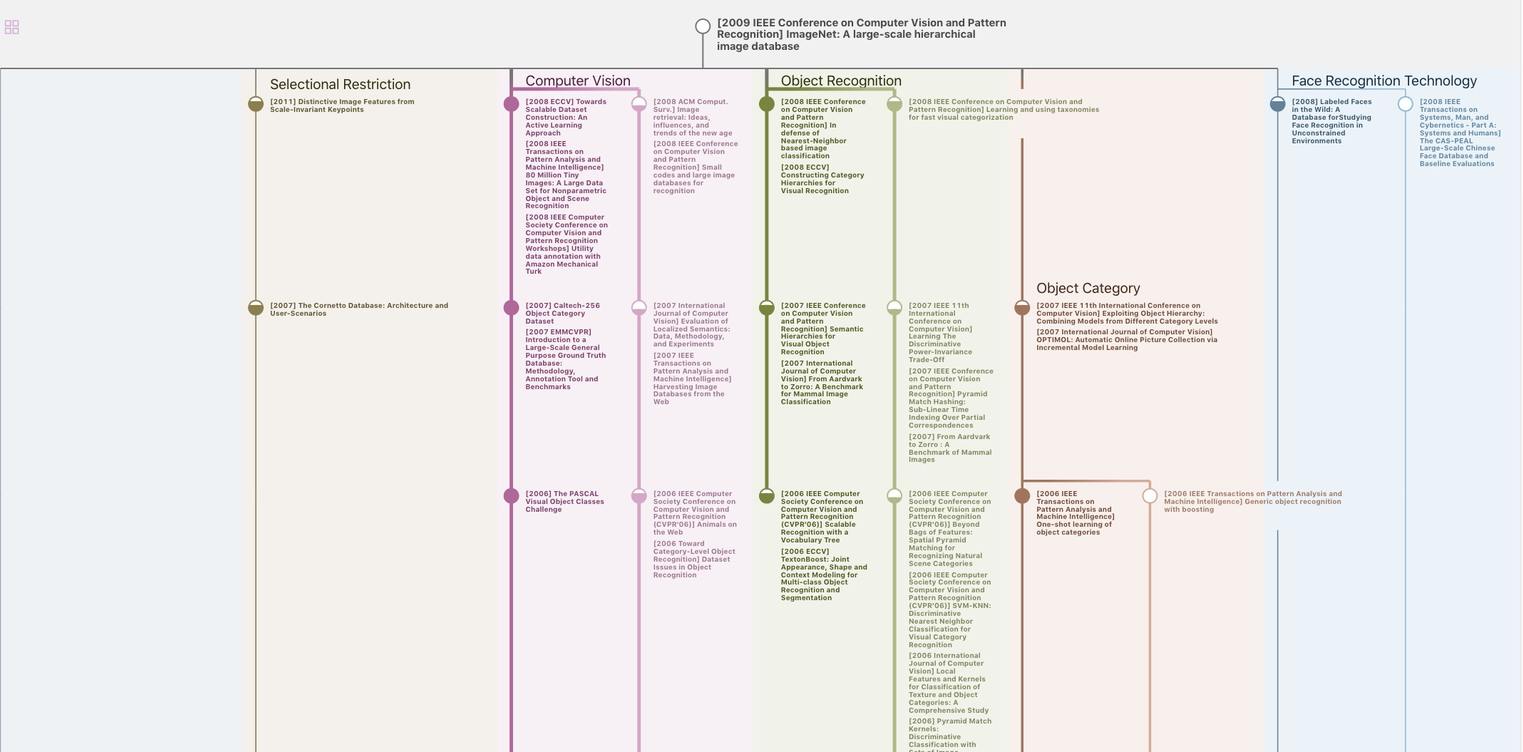
生成溯源树,研究论文发展脉络
Chat Paper
正在生成论文摘要