STANN: A Spatio-Temporal Attentive Neural Network for Traffic Prediction.
IEEE ACCESS(2019)
摘要
Recently, traffic prediction based on deep learning methods has attracted much attention. However, there still exist two major challenges, namely, dynamic spatio-temporal dependences among network-wide links and long-term traffic prediction for the next few hours. To address these two challenges, this paper proposes a spatio-temporal attentive neural network (STANN) for the network-wide and long-term traffic prediction. The STANN captures the spatial-temporal dependences based on the encoder-decoder architecture with the attention mechanisms. In the encoder, the STANN learns the spatio-temporal dependences from historical traffic series using a recurrent neural network (RNN) with long short-term memory (LSTM) units, in which a new spatial attention model is developed to consider the contribution of each link to the network-wide prediction. In the decoder, the STANN exploits another RNN with LSTM units and a temporal attention model to select the relevant and important historical spatio-temporal dependences from the encoder for long-term traffic prediction. Finally, we conduct extensive experiments to evaluate STANN on three real-world traffic datasets. The experimental results show that the STANN is significantly better than other state-of-the-art models.
更多查看译文
关键词
Spatio-temporal data,deep neural network,attention mechanism,traffic prediction
AI 理解论文
溯源树
样例
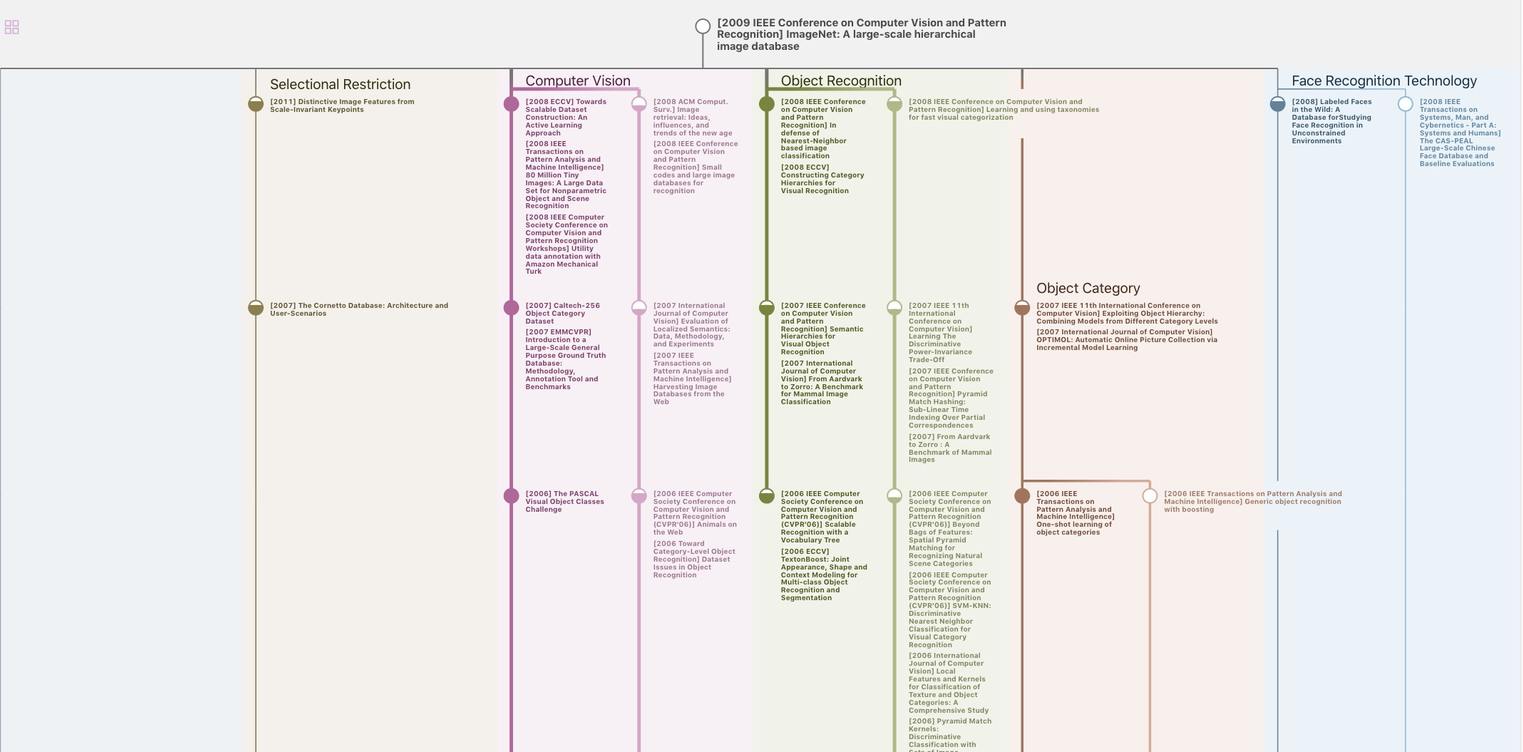
生成溯源树,研究论文发展脉络
Chat Paper
正在生成论文摘要