Uncertainty Modelling in Deep Networks: Forecasting Short and Noisy Series
MACHINE LEARNING AND KNOWLEDGE DISCOVERY IN DATABASES, ECML PKDD 2018, PT III(2018)
摘要
Deep Learning is a consolidated, state-of-the-art Machine Learning tool to fit a function when provided with large data sets of examples. However, in regression tasks, the straightforward application of Deep Learning models provides a point estimate of the target. In addition, the model does not take into account the uncertainty of a prediction. This represents a great limitation for tasks where communicating an erroneous prediction carries a risk. In this paper we tackle a real-world problem of forecasting impending financial expenses and incomings of customers, while displaying predictable monetary amounts on a mobile app. In this context, we investigate if we would obtain an advantage by applying Deep Learning models with a Heteroscedastic model of the variance of a network's output. Experimentally, we achieve a higher accuracy than non-trivial baselines. More importantly, we introduce a mechanism to discard low-confidence predictions, which means that they will not be visible to users. This should help enhance the user experience of our product.
更多查看译文
关键词
Deep Learning,Uncertainty,Aleatoric models,Time-series
AI 理解论文
溯源树
样例
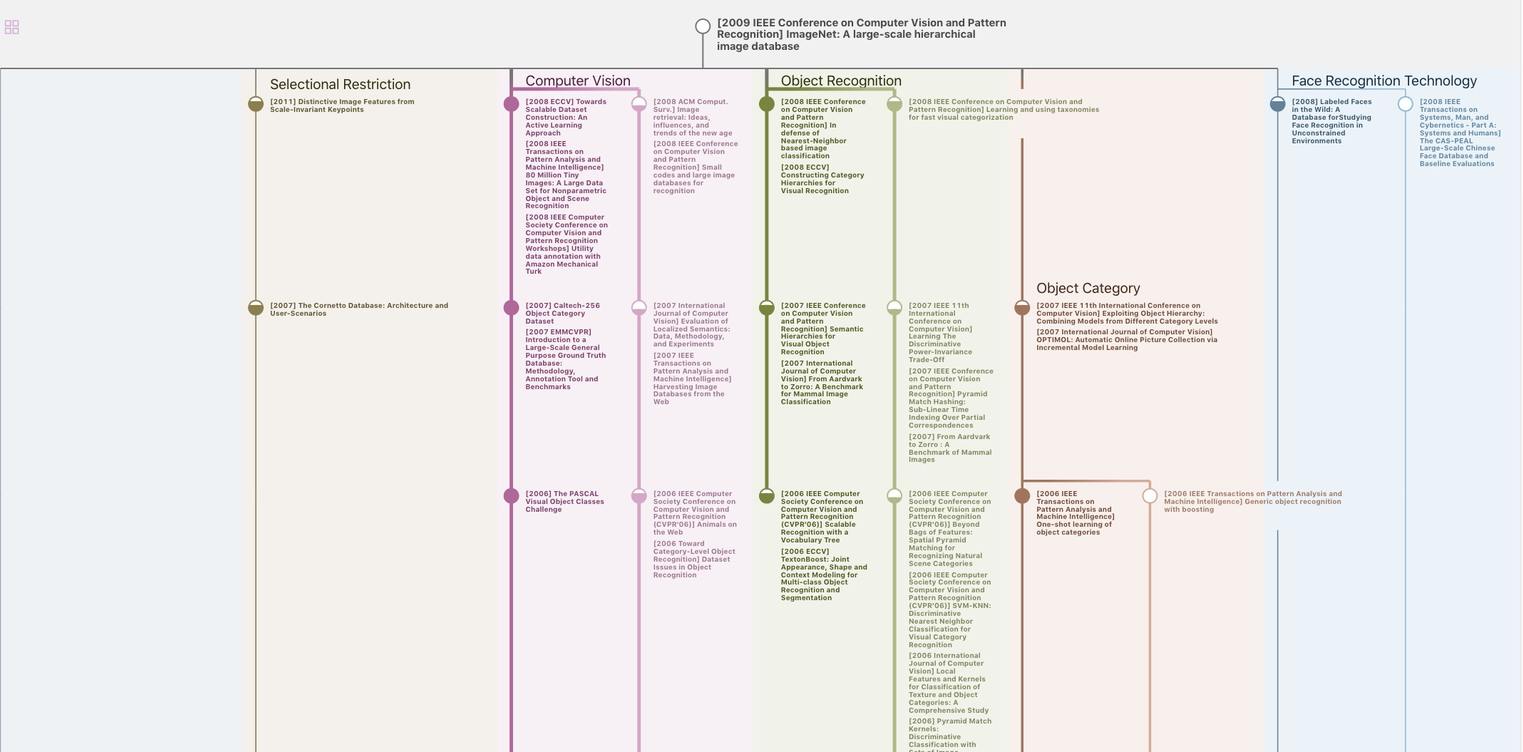
生成溯源树,研究论文发展脉络
Chat Paper
正在生成论文摘要