MetaBags: Bagged Meta-Decision Trees for Regression
MACHINE LEARNING AND KNOWLEDGE DISCOVERY IN DATABASES, ECML PKDD 2018, PT I(2018)
摘要
Methods for learning heterogeneous regression ensembles have not yet been proposed on a large scale. Hitherto, in classical ML literature, stacking, cascading and voting are mostly restricted to classification problems. Regression poses distinct learning challenges that may result in poor performance, even when using well established homogeneous ensemble schemas such as bagging or boosting. In this paper, we introduce MetaBags, a novel stacking framework for regression. MetaBags learns a set of meta-decision trees designed to select one base model (i.e. expert) for each query, and focuses on inductive bias reduction. Finally, these predictions are aggregated into a single prediction through a bagging procedure at meta-level. MetaBags is designed to learn a model with a fair bias-variance trade-off, and its improvement over base model performance is correlated with the prediction diversity of different experts on specific input space subregions. An exhaustive empirical testing of the method was performed, evaluating both generalization error and scalability of the approach on open, synthetic and real-world application datasets. The obtained results show that our method outperforms existing state-of-the-art approaches.
更多查看译文
关键词
Stacking,Regression,Meta-learning,Landmarking
AI 理解论文
溯源树
样例
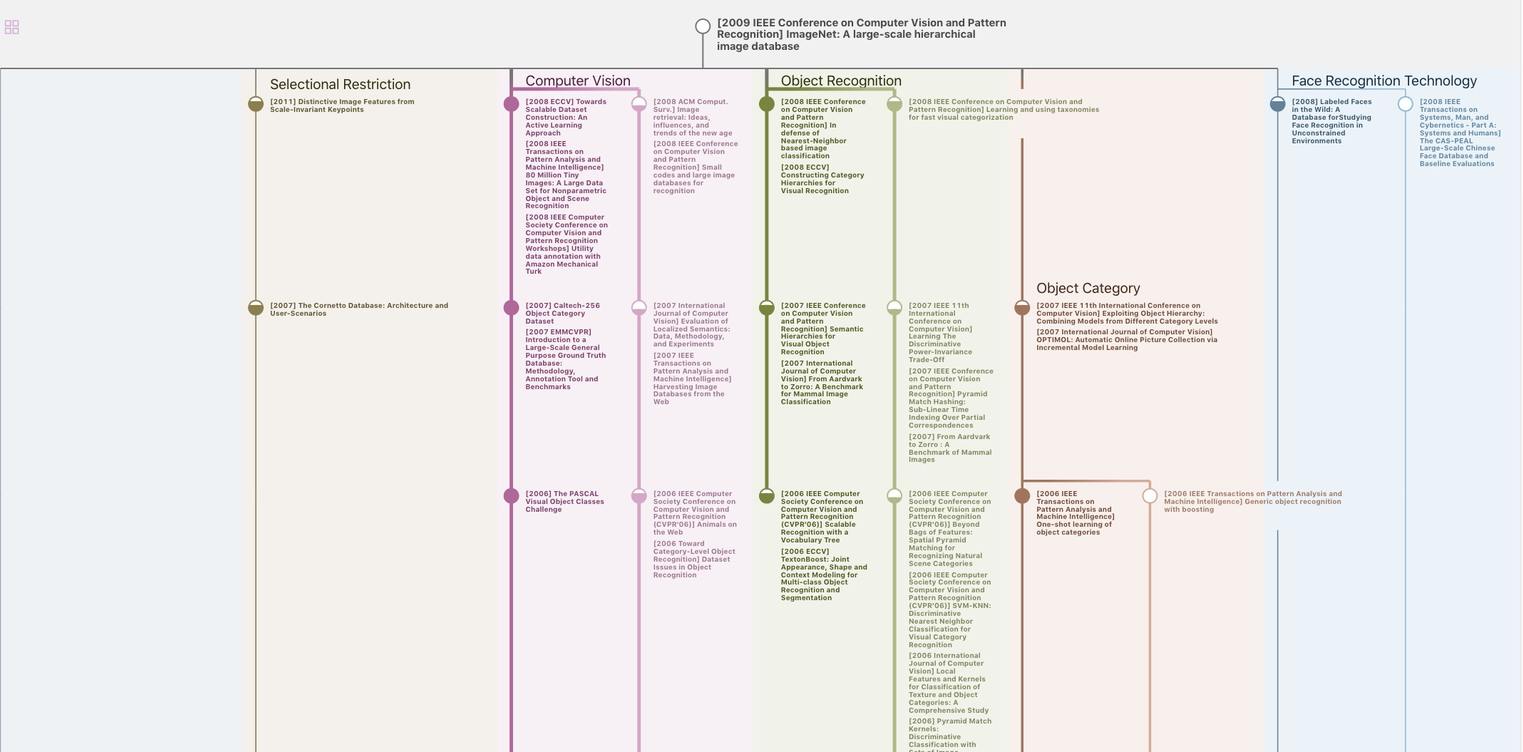
生成溯源树,研究论文发展脉络
Chat Paper
正在生成论文摘要