Transforming Mixed Data Bases For Machine Learning: A Case Study
ADVANCES IN SOFT COMPUTING, MICAI 2018, PT I(2018)
摘要
Structured Data Bases which include both numerical and categorical attributes (Mixed Databases or MD) ought to be adequately pre-processed so that machine learning algorithms may be applied to their analysis and further processing. Of primordial importance is that the instances of all the categorical attributes be encoded so that the patterns embedded in the MD be preserved. We discuss CESAMO, an algorithm that achieves this by statistically sampling the space of possible codes. CESAMO's implementation requires the determination of the moment when the codes distribute normally. It also requires the approximation of an encoded attribute as a function of other attributes such that the best code assignment may be identified. The MD's categorical attributes are thusly mapped into purely numerical ones. The resulting numerical database (ND) is then accessible to supervised and non-supervised learning algorithms. We discuss CESAMO, normality assessment and functional approximation. A case study of the US census database is described. Data is made strictly numerical using CESAMO. Neural Networks and Self-Organized Maps are then applied. Our results are compared to classical analysis. We show that CESAMO's application yields better results.
更多查看译文
关键词
Machine Learning, Mixed Databases, Non-linear regression, Goodness-of-fit
AI 理解论文
溯源树
样例
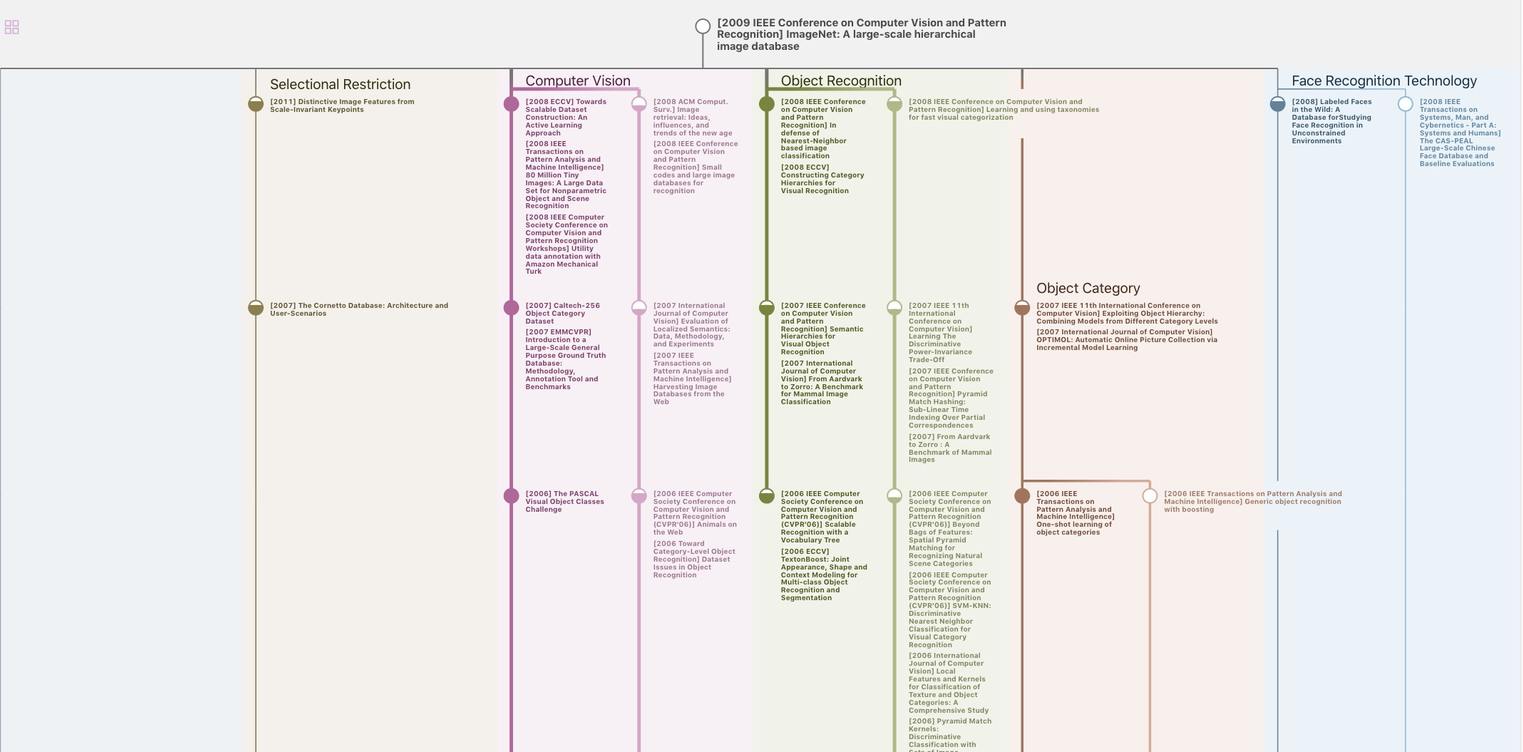
生成溯源树,研究论文发展脉络
Chat Paper
正在生成论文摘要