Deep Learning Forecasting Based on Auto-LSTM Model for Home Solar Power Systems
2018 IEEE 20th International Conference on High Performance Computing and Communications; IEEE 16th International Conference on Smart City; IEEE 4th International Conference on Data Science and Systems (HPCC/SmartCity/DSS)(2018)
摘要
The Internet of things is widely used to provide a lot of useful services such as human health care, security systems and green energy monitoring. It contributes to the sustainable development of smart cities in order to manage and integrate renewable energies. The swift growth of Home Solar Power Systems (HSPS) has enabled a large-scale collection of time series data. As advanced tools, smart meters can ensure the timely reading of HSPS data, automating metering and producing fine-grained data. However, to ensure the dynamic data management and a better understanding of HSPS operations, it is crucial to analyze and forecast these digital records for decision-making and smart control. Up to now, deep learning algorithms have only been applied sparsely in the field of renewable energy power forecasting. In this paper, we apply an auto-configurable middleware based on a Long Short Term Memory (LSTM) model for several forecasting time dimensions to choose the significant timescale for learning setting. The results show that our deep-learning model has good performances compared to the Support Vector Machine (SVM) model for the whole proposed learning timescale. However, the Auto-Regressive Integrated Moving Average (ARIMA) seems better than our proposed auto-LSTM algorithm, but it takes much execution time for 15 min and 30 min-ahead forecasting. Thus, the day-ahead forecasting is the most efficient timescale in our case.
更多查看译文
关键词
Auto-LSTM,Home Solar Power Systems,PV power forecasting,Smart meters
AI 理解论文
溯源树
样例
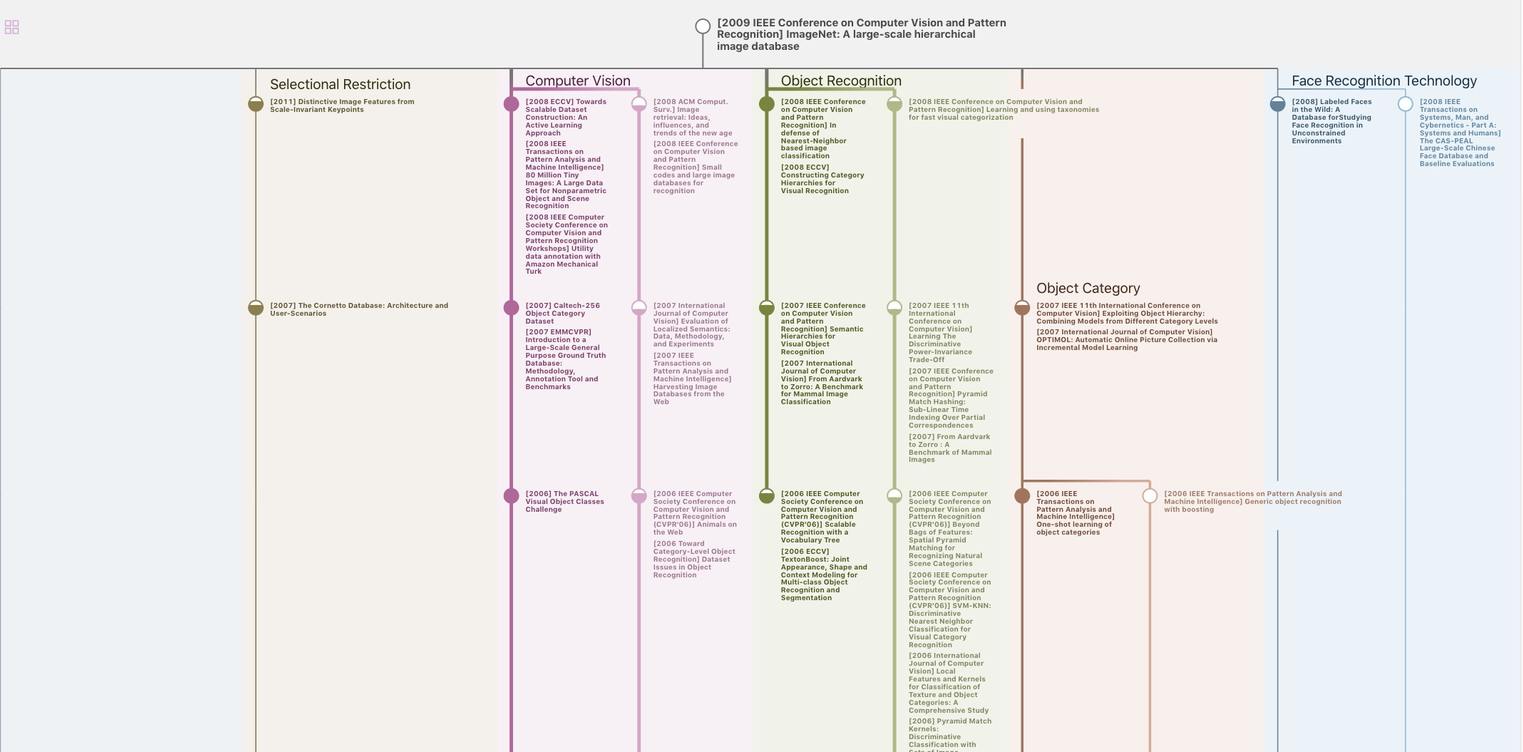
生成溯源树,研究论文发展脉络
Chat Paper
正在生成论文摘要