Reinforcement Learning Scheduler for Vehicle-to-Vehicle Communications Outside Coverage
2018 IEEE Vehicular Networking Conference (VNC)(2018)
摘要
Radio resources in vehicle-to-vehicle (V2V) communication can be scheduled either by a centralized scheduler residing in the network (e.g., a base station in case of cellular systems) or a distributed scheduler, where the resources are autonomously selected by the vehicles. The former approach yields a considerably higher resource utilization in case the network coverage is uninterrupted. However, in case of intermittent or-of-coverage, due to not having input from centralized scheduler, vehicles need to revert to distributed scheduling.Motivated by recent advances in reinforcement learning (RL), we investigate whether a centralized learning scheduler can be taught to efficiently pre-assign the resources to vehicles for-of-coverage V2V communication. Specifically, we use the actor-critic RL algorithm to train the centralized scheduler to provide non-interfering resources to vehicles before they enter the-of-coverage area.Our initial results show that a RL-based scheduler can achieve performance as good as or better than the state-of-art distributed scheduler, often outperforming it. Furthermore, the learning process completes within a reasonable time (ranging from a few hundred to a few thousand epochs), thus making the RL-based scheduler a promising solution for V2V communications with intermittent network coverage.
更多查看译文
关键词
V2V,Out of Coverage,Radio Resource Allocation,Scheduling,Reinforcement Learning
AI 理解论文
溯源树
样例
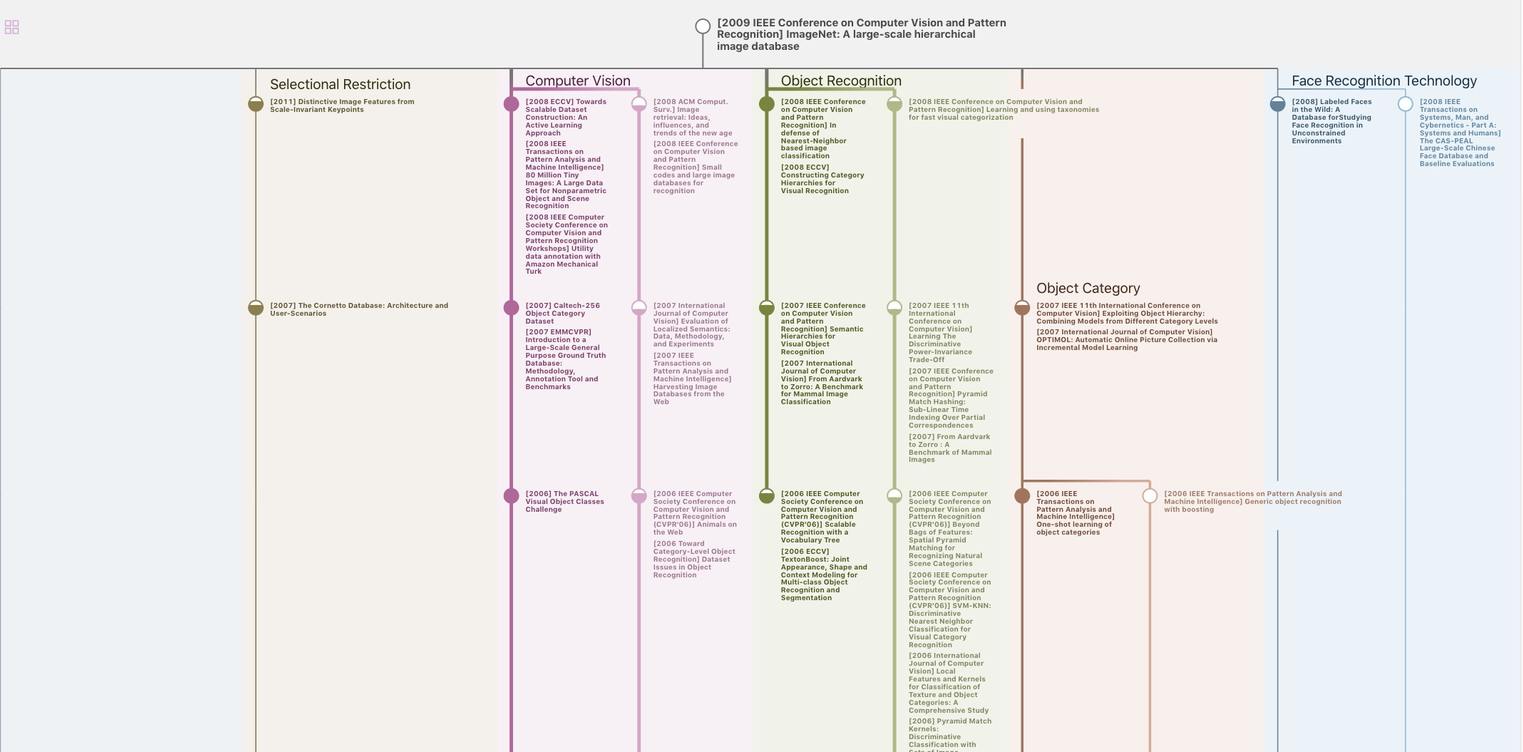
生成溯源树,研究论文发展脉络
Chat Paper
正在生成论文摘要