Feature Fusing of Feature Pyramid Network for Multi-Scale Pedestrian Detection
2018 15th International Computer Conference on Wavelet Active Media Technology and Information Processing (ICCWAMTIP)(2018)
摘要
Pedestrian detection is a fundamental component in many real-world applications such as automatic driving, intelligent surveillance, person re-identification and robotics. Therefore, it has attracted massive attention in the last decades. However, pedestrians in an images always exhibit different scales, which constitutes a significant mode of intra-class variability and affect the performance of pedestrian detection algorithm. To address this problem, we apply FPN (Feature Pyramid Network)for pedestrian detection. FPN exploits the inherent multi-scale structure of a deep convolutional network to construct a feature pyramid that has rich semantics at all levels and facilitates the detection of objects at different scales. To leverage the information from different levels of the feature pyramid, we extend the FPN-based pedestrian detection by fusing the feature of each level with adaptive feature pooling. Furthermore, we also integrate a Squeeze and Excitation module to the ROI pooled features from each level before the feature fusion. The experiment result on Caltech dataset shows that our approach outperforms the basic FPN-based pedestrian detection and robust towards to various scale of pedestrian.
更多查看译文
关键词
Feature extraction,Proposals,Semantics,Object detection,Training,Adaptation models,Detectors
AI 理解论文
溯源树
样例
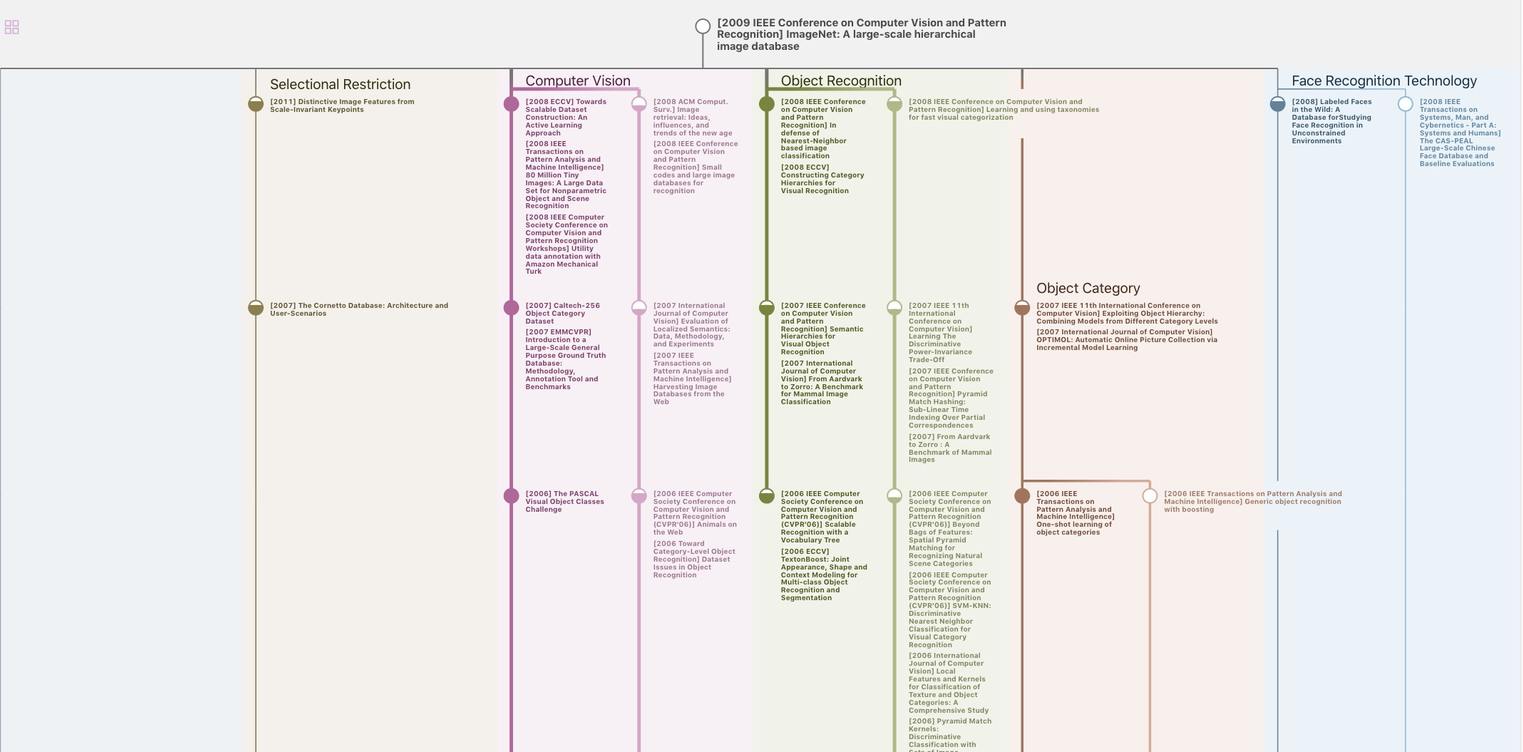
生成溯源树,研究论文发展脉络
Chat Paper
正在生成论文摘要