An Integrated Approach Based On Eeg Signals Processing Combined With Supervised Methods To Classify Alzheimer'S Disease Patients
PROCEEDINGS 2018 IEEE INTERNATIONAL CONFERENCE ON BIOINFORMATICS AND BIOMEDICINE (BIBM)(2018)
摘要
Alzheimer's Disease (AD) is the most widespread and incurable neurodegenerative disorder, and together with its preliminary stage - Mild Cognitive Impairment (MCI) - its detection still remains a challenging issue. Electroencephalography (EEG) is a non-invasive and repeatable technique to diagnose brain abnormalities. However, the analysis of EEG spectra is still carried out manually by experts and effective computer science methods to extract relevant information from these signals become a necessity. Through a data mining approach, which guides the automated knowledge discovery process, we aim to achieve an automatic patients classification from the EEG biomedical signals of AD and MCI, in order to support medical doctors in the diagnosis formulation. Specifically, we design an integrated procedure that encompasses the following steps: (1) data collection; (2) data preprocessing of EEG-signals data; (3) features extraction by applying time-frequency transforms on EEG-signals (Fourier and Wavelet analysis); and (3) a supervised learning approach to classify samples in patients suffering from AD, patients affected by MCI, and healthy control (HC) subjects. By applying our procedure, we are able to extract human-interpretable classification models that allow to automatically assign the patients into their belonging class. In particular, by exploiting a Wavelet feature extraction we achieve 83%, 92%, and 79% of accuracy when dealing with HC vs AD, HC vs MCI, and MCI vs AD classification problems, respectively. By comparing the classification performances with both feature extraction methods, we find out that Wavelets analysis outperforms Fourier. Thus, we suggest it in combination with supervised methods for automatic patients classification based on their EEG signals for aiding the medical diagnosis of dementia. We provided processed data from our study at ftp://bioinformatics.iasi.cnr.it/public/EEG/.
更多查看译文
关键词
EEG signals analysis, supervised learning, Alzheimer's Disease
AI 理解论文
溯源树
样例
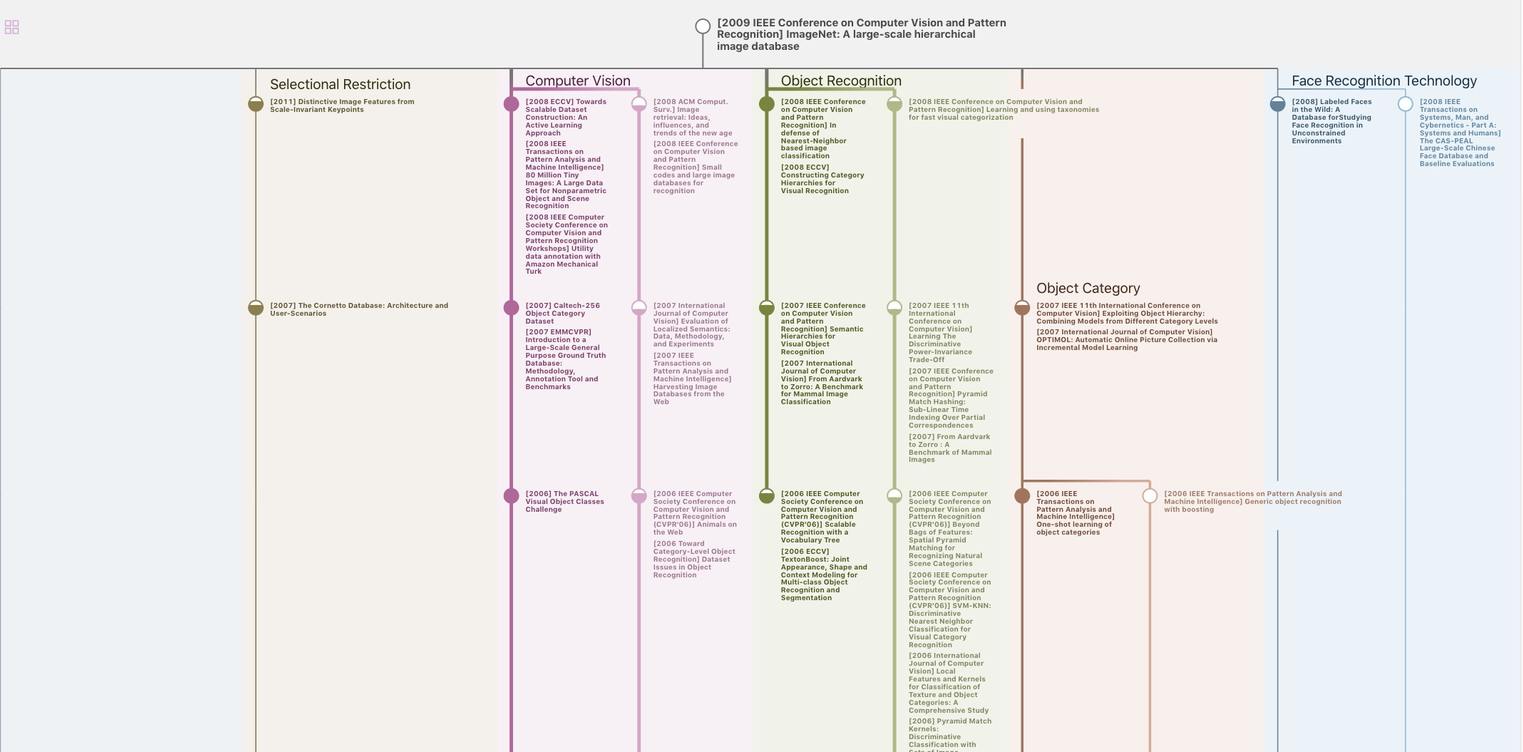
生成溯源树,研究论文发展脉络
Chat Paper
正在生成论文摘要