Synthesis of Constraints for Mathematical Programming With One-Class Genetic Programming
IEEE Transactions on Evolutionary Computation(2019)
摘要
Mathematical programming (MP) models are common in optimization of real-world processes. Models are usually built by optimization experts in an iterative manner: an imperfect model is continuously improved until it approximates the reality well-enough and meets all technical requirements (e.g., linearity). To facilitate this task, we propose a genetic one-class constraint synthesis method (GOCCS). Given a set of exemplary states of normal operation of a business process, GOCCS synthesizes constraints in linear programming or nonlinear programming form. The synthesized constraints can be then paired with an arbitrary objective function and supplied to an off-the-shelf solver to find optimal parameters of the process. We assess GOCCS on three families of MP benchmarks and conclude promising results. We also apply it to a real-world process of wine production and optimize that process.
更多查看译文
关键词
Linear programming,Computational modeling,Programming,Genetic programming,Mathematical model,Benchmark testing
AI 理解论文
溯源树
样例
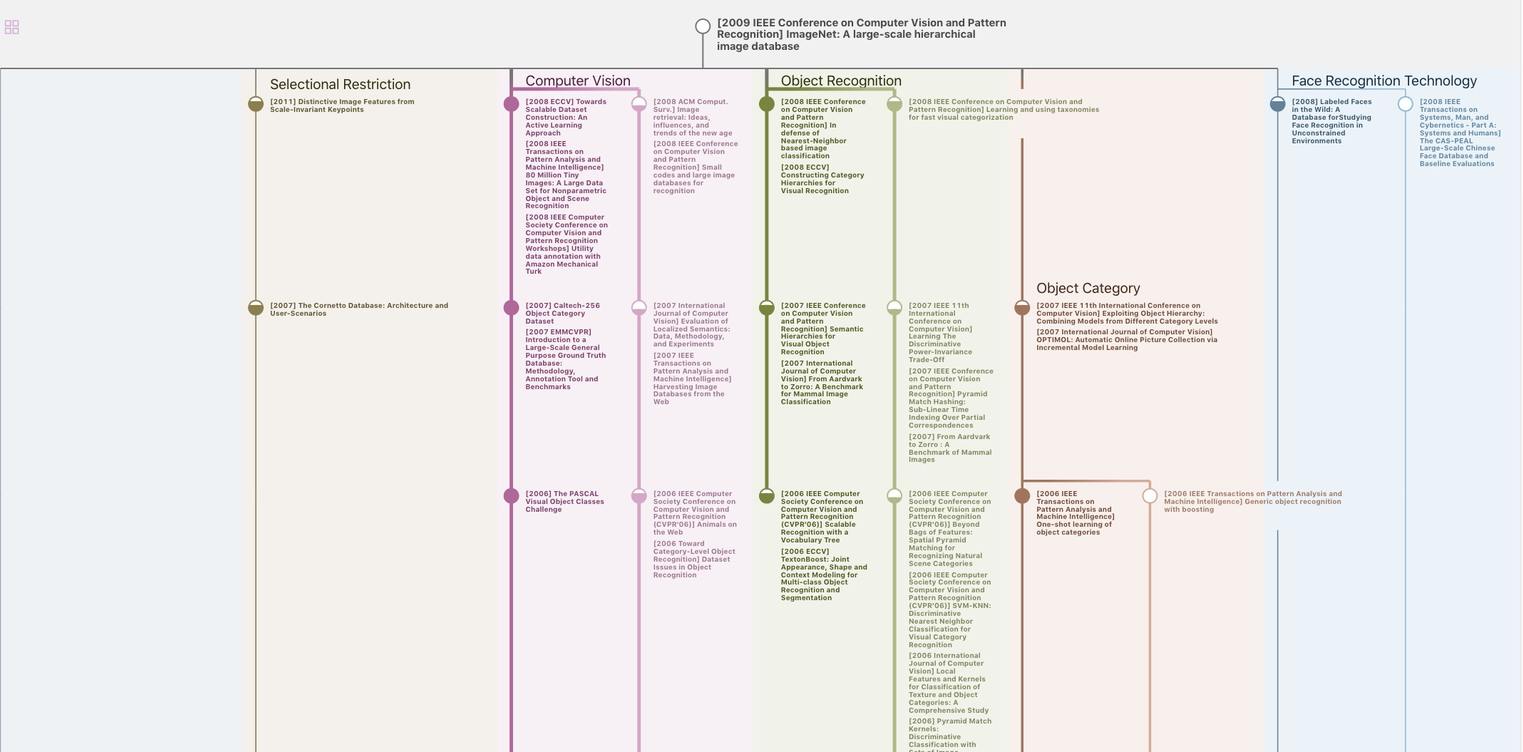
生成溯源树,研究论文发展脉络
Chat Paper
正在生成论文摘要