Using Discriminative Graphical Models for Insurance Recommender Systems
2018 17th IEEE International Conference on Machine Learning and Applications (ICMLA)(2018)
摘要
Recommender systems have become extremely important to various types of industries where customer interaction and feedback is paramount to the success of the business. For companies that face changes that arise with ever-growing markets, providing product recommendations to new and existing customers is a challenge. Furthermore, it is important to have an algorithm which is descriptive, scalable, agnostic to missing features, and robust in providing these recommendations. Directed graphical models meet all these demands; however, if the dimensionality of the features is high, structure learning and inference can become computationally prohibitive. In this work, we propose an algorithm with some novel aspects to learn the structure of a graphical model (e.g. Bayesian network), which considerably speeds up both training (from days to minutes in some cases) and inference run-times with respect to standard Bayesian structure learning approaches, while achieving similar accuracy. We also show that this approach produces more accurate predictions than a state-of-the-art matrix factorization algorithm in the absence of complete evidence on several insurance-related datasets.
更多查看译文
关键词
Recommender systems, Structure Learning, Bayesian Networks, Insurance domain, Deployed system
AI 理解论文
溯源树
样例
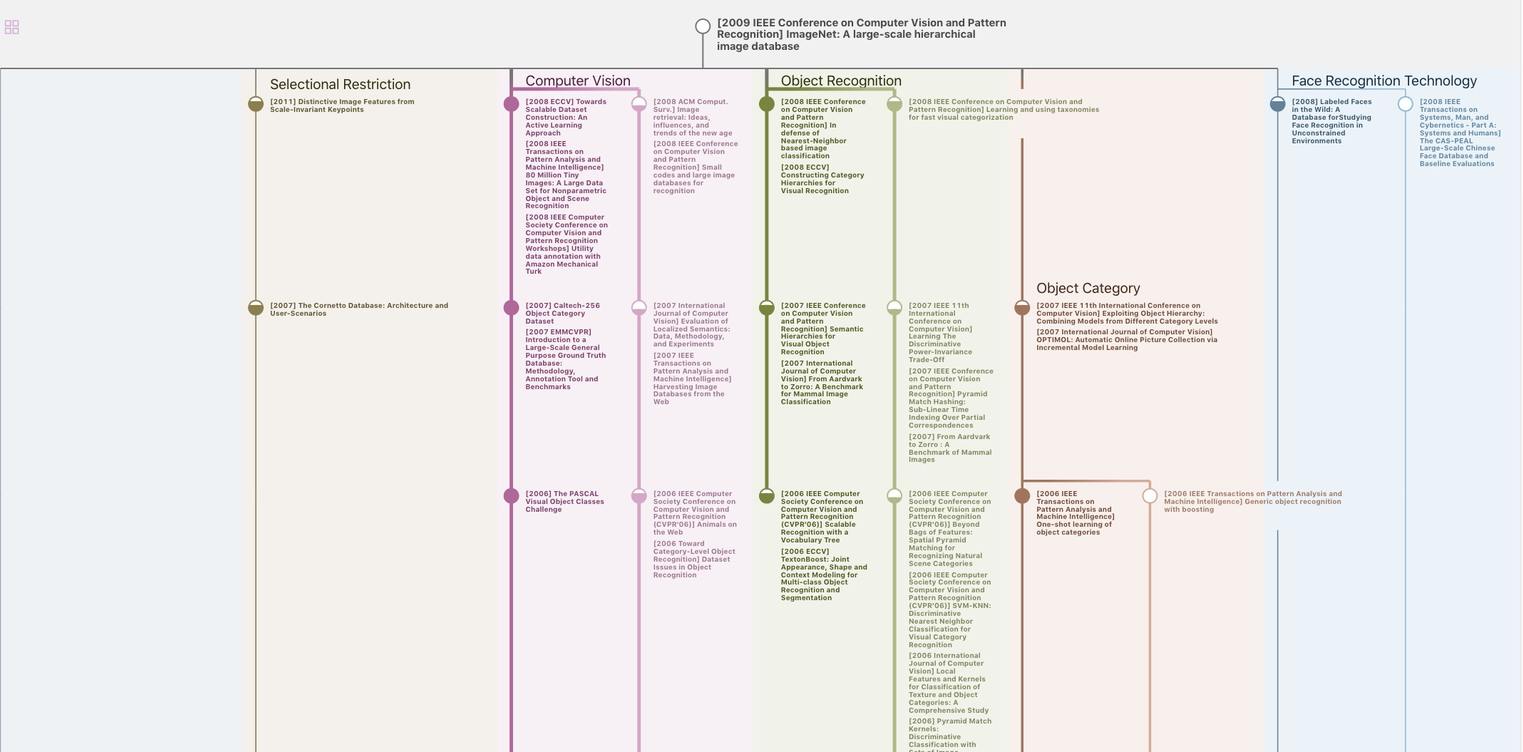
生成溯源树,研究论文发展脉络
Chat Paper
正在生成论文摘要