Starlord: Sliding Window Temporal Accumulate-Retract Learning For Online Reasoning On Datastreams
2018 17TH IEEE INTERNATIONAL CONFERENCE ON MACHINE LEARNING AND APPLICATIONS (ICMLA)(2018)
摘要
Nowadays, data sources, such as IoT devices, financial markets, and online services, continuously generate large amounts of data. Such data is usually generated at high frequencies and is typically described by non-stationary distributions. Querying these data sources brings new challenges for machine learning algorithms, which now need to be considered from the perspective of an evolving stream and not a static dataset. Under such scenarios, where data flows continuously, the challenge is how to transform the vast amount of data into information and knowledge, and how to adapt to data changes (i.e. drifts) and accumulate experience over time to support online decision-making. In this paper, we introduce STARLORD, a novel incremental computation method and system acting on data streams and capable of achieving low-latency (millisecond level) and high-throughput (thousands events/second/core) when learning from data streams. Moreover, the approach is able to adapt to data drifts and accumulate experience over time, and to use such knowledge to improve future learning and prediction performance, with resource usage guarantees. This is proven by our preliminary experiments where we built-in the framework in an open source stream engine (i.e. Apache Flink).
更多查看译文
关键词
Streaming Data, Online Machine Learning, Distributed Computation, Incremental Computation
AI 理解论文
溯源树
样例
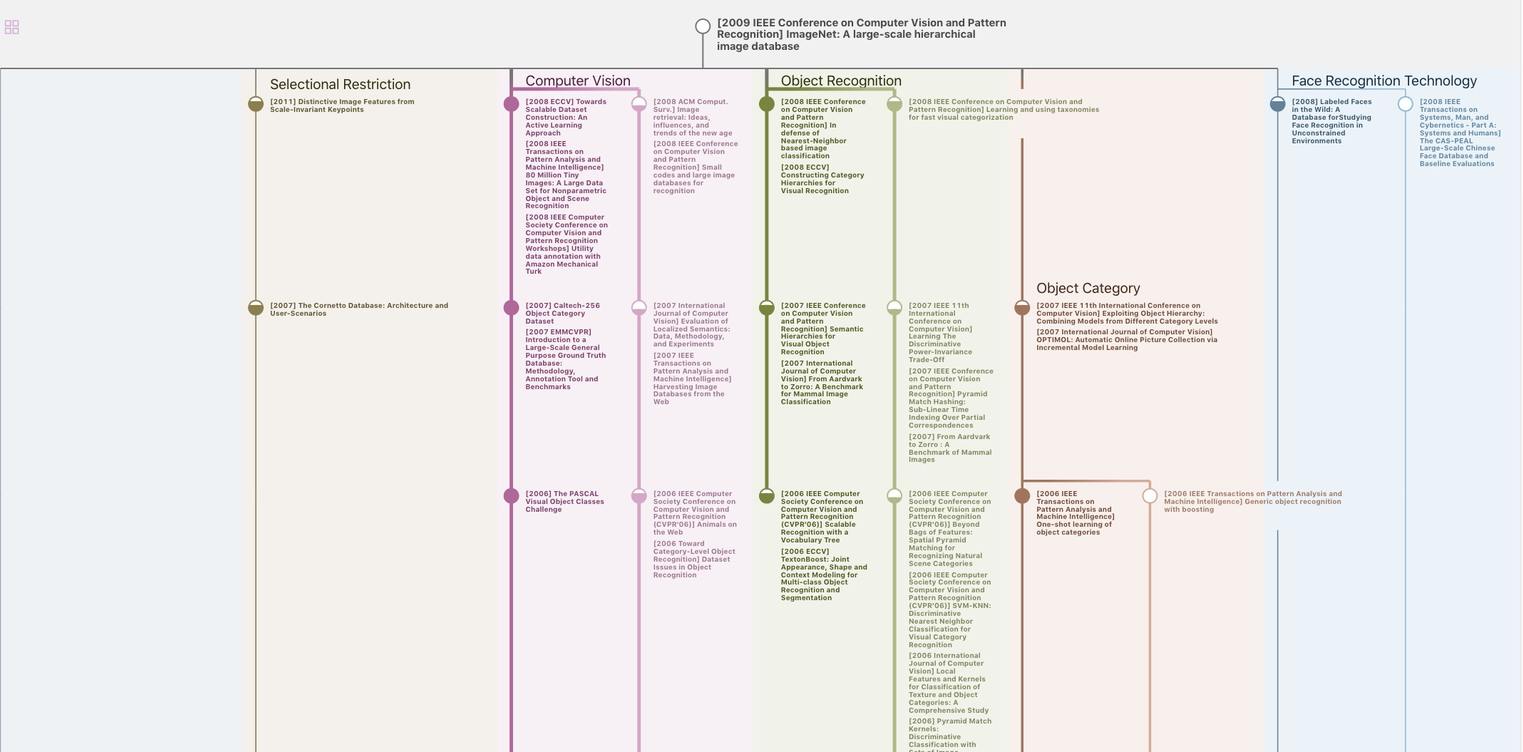
生成溯源树,研究论文发展脉络
Chat Paper
正在生成论文摘要