Variable Entropy Of Noise In Evaluation Of Effectiveness Of Context Usage By Machine Learning Methods
2018 IEEE INTERNATIONAL CONFERENCE ON SYSTEMS, MAN, AND CYBERNETICS (SMC)(2018)
摘要
Solving a problem requires knowledge of its context - the information needed to solve it. With the advent of Big Data and ML, huge amounts of data is collected in the desire to develop systems which will then find solutions to specified problems. This requires algorithms to discover and effectively use context hidden within provided data. A system more adept in this task can be regarded as more computationally aware of requirements, data sources and methods that are important to solve given problems. This is a highly desirable property which helps to achieve goals. In this article we present a method for estimating the effectiveness of context usage of machine learning algorithms. It is based on comparison of machine learning models trained on data containing various forms of injected context created with use of noise of varying entropy levels. Finally we give results of using this solution on selected machine learning algorithms and benchmark problems from ICxS Contextual Data repository.
更多查看译文
关键词
context awareness, context injection, context search effectiveness, entropy, distributed random forest, deep learning
AI 理解论文
溯源树
样例
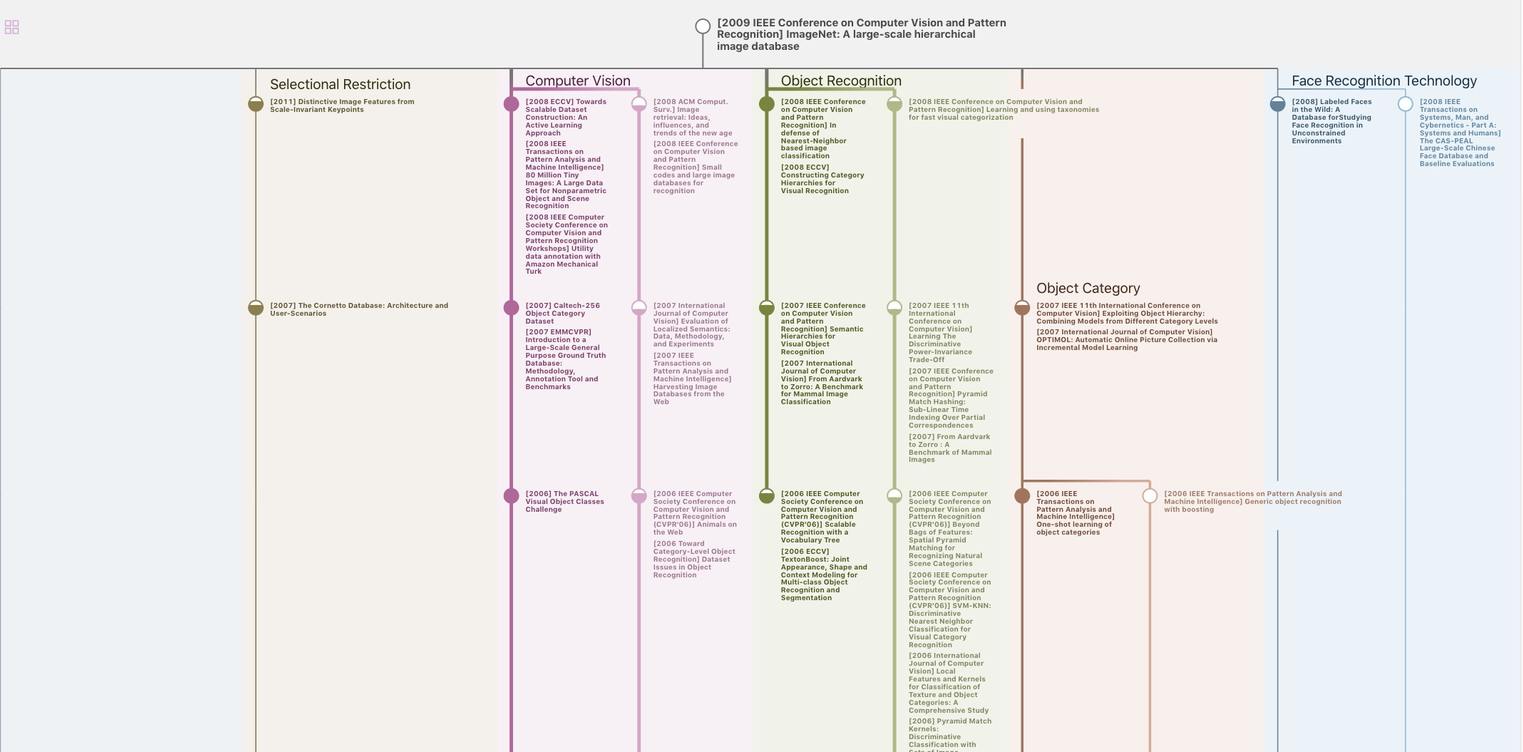
生成溯源树,研究论文发展脉络
Chat Paper
正在生成论文摘要