Evaluation of Distributed Machine Learning Algorithms for Anomaly Detection from Large-Scale System Logs: A Case Study
2018 IEEE INTERNATIONAL CONFERENCE ON BIG DATA (BIG DATA)(2018)
摘要
Anomaly detection is a valuable feature for detecting and diagnosing faults in large-scale, distributed systems. These systems usually provide tens of millions of lines of logs that can be exploited for this purpose. However, centralized implementations of traditional machine learning algorithms fall short to analyze this data in a scalable manner. One way to address this challenge is to employ distributed systems to analyze the immense amount of logs generated by other distributed systems. We conducted a case study to evaluate two unsupervised machine learning algorithms for this purpose on a benchmark dataset. In particular, we evaluated distributed implementations of PCA and K-means algorithms. We compared the accuracy and performance of these algorithms both with respect to each other and with respect to their centralized implementations. Results showed that the distributed versions can achieve the same accuracy and provide a performance improvement by orders of magnitude when compared to their centralized versions. The performance of PCA turns out to be better than K-means, although we observed that the difference between the two tends to decrease as the degree of parallelism increases.
更多查看译文
关键词
log analysis, distributed systems, parallel processing, anomaly detection, big data, machine learning
AI 理解论文
溯源树
样例
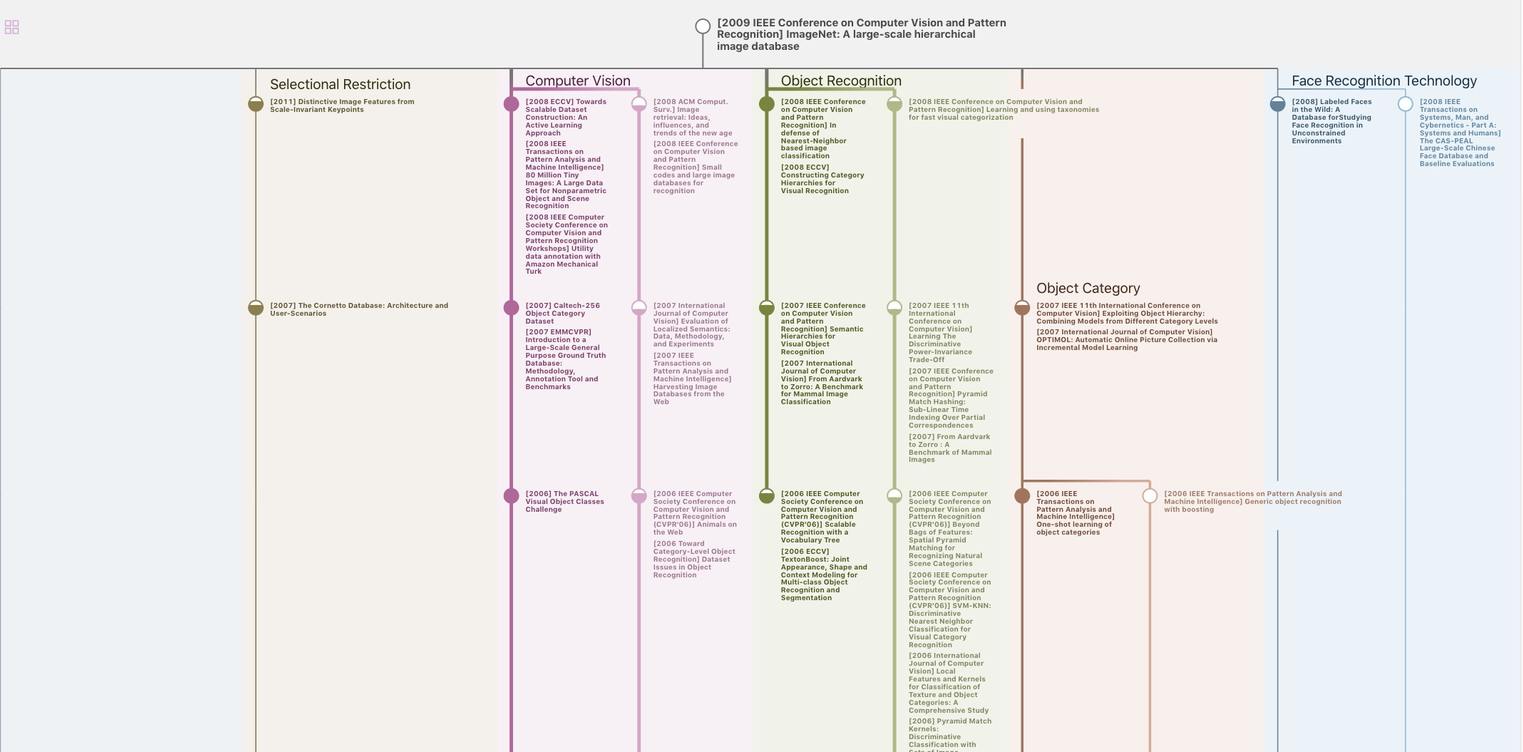
生成溯源树,研究论文发展脉络
Chat Paper
正在生成论文摘要