Spectrum Data Poisoning With Adversarial Deep Learning
2018 IEEE MILITARY COMMUNICATIONS CONFERENCE (MILCOM 2018)(2018)
摘要
Machine learning has been applied in wireless communications. In this paper, we consider the case that a cognitive transmitter senses the spectrum and transmits on idle channels determined by a machine learning algorithm. We then present an adversarial machine learning approach to launch a spectrum data poisoning attack. That is, an adversary learns the transmitter's behavior and attempts to falsify the spectrum sensing data over the air by transmitting for a short period of time when the channel is idle to manipulate the input for the decision mechanism of the transmitter. The cognitive engine at the transmitter is a deep neural network model that predicts idle channels with minimum sensing error for data transmissions. The transmitter collects spectrum sensing data and uses it as the input to its machine learning algorithm. In the meantime, the adversary also builds a cognitive engine using another deep neural network model to predict when the transmitter will have a successful transmission based on its spectrum sensing data. The adversary then performs the over-the-air spectrum data poisoning attack, which aims to change the channel occupancy status from idle to busy when the transmitter is sensing, so that the transmitter is fooled into making incorrect transmit decisions. This attack is more energy efficient and harder to detect compared to jamming of data transmissions. We show that this attack is very effective and reduces the throughput of the transmitter substantially.
更多查看译文
关键词
Adversarial machine learning, deep learning, cognitive radio, exploratory attack, spectrum data poisoning, spectrum data falsification
AI 理解论文
溯源树
样例
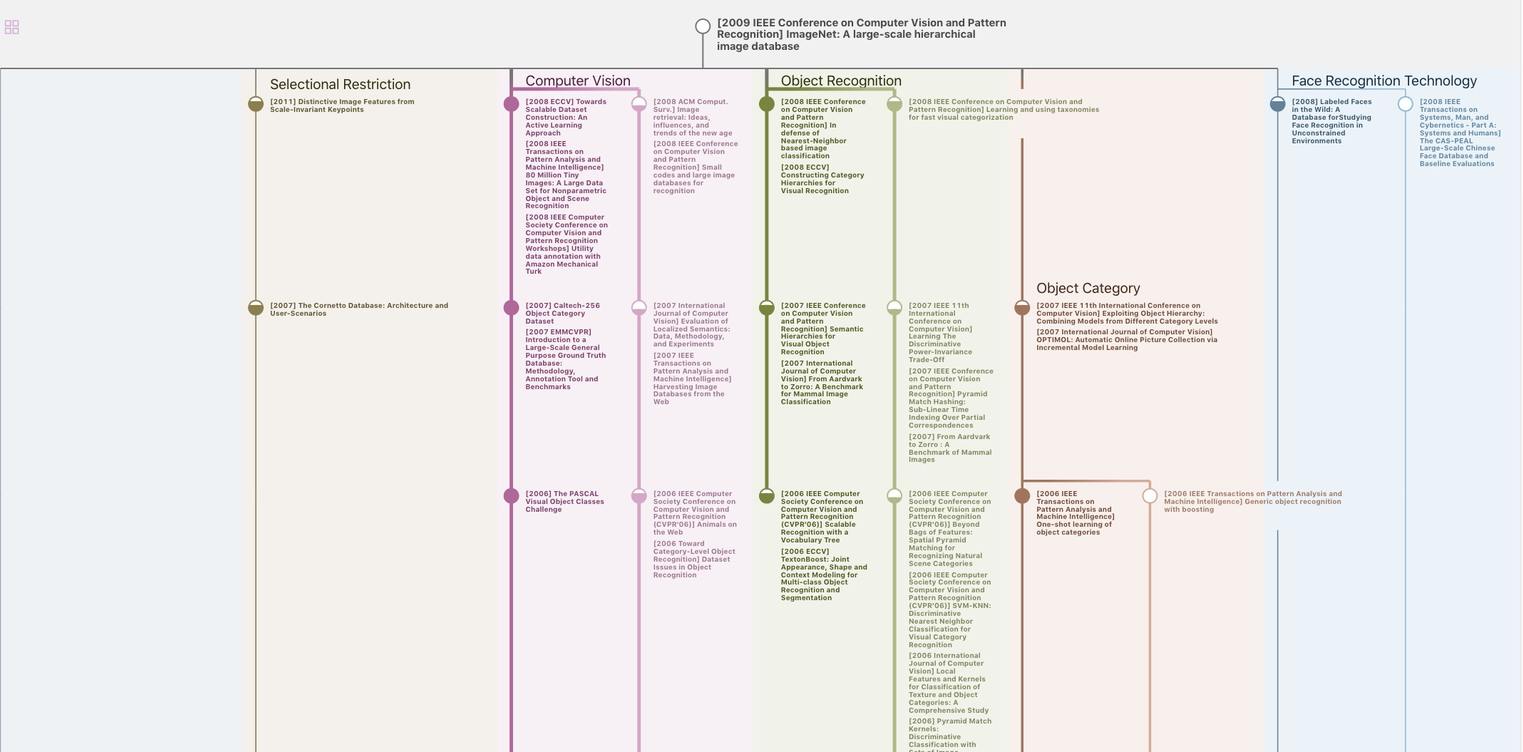
生成溯源树,研究论文发展脉络
Chat Paper
正在生成论文摘要