CONV-SRAM: An Energy-Efficient SRAM With In-Memory Dot-Product Computation for Low-Power Convolutional Neural Networks
IEEE Journal of Solid-State Circuits(2019)
摘要
This paper presents an energy-efficient static random access memory (SRAM) with embedded dot-product computation capability, for binary-weight convolutional neural networks. A 10T bit-cell-based SRAM array is used to store the 1-b filter weights. The array implements dot-product as a weighted average of the bitline voltages, which are proportional to the digital input values. Local integrating analog-to-digital converters compute the digital convolution outputs, corresponding to each filter. We have successfully demonstrated functionality (>98% accuracy) with the 10 000 test images in the MNIST hand-written digit recognition data set, using 6-b inputs/outputs. Compared to conventional full-digital implementations using small bitwidths, we achieve similar or better energy efficiency, by reducing data transfer, due to the highly parallel in-memory analog computations.
更多查看译文
关键词
Convolution,Random access memory,Computer architecture,Cloud computing,Edge computing,Neural networks,Feature extraction
AI 理解论文
溯源树
样例
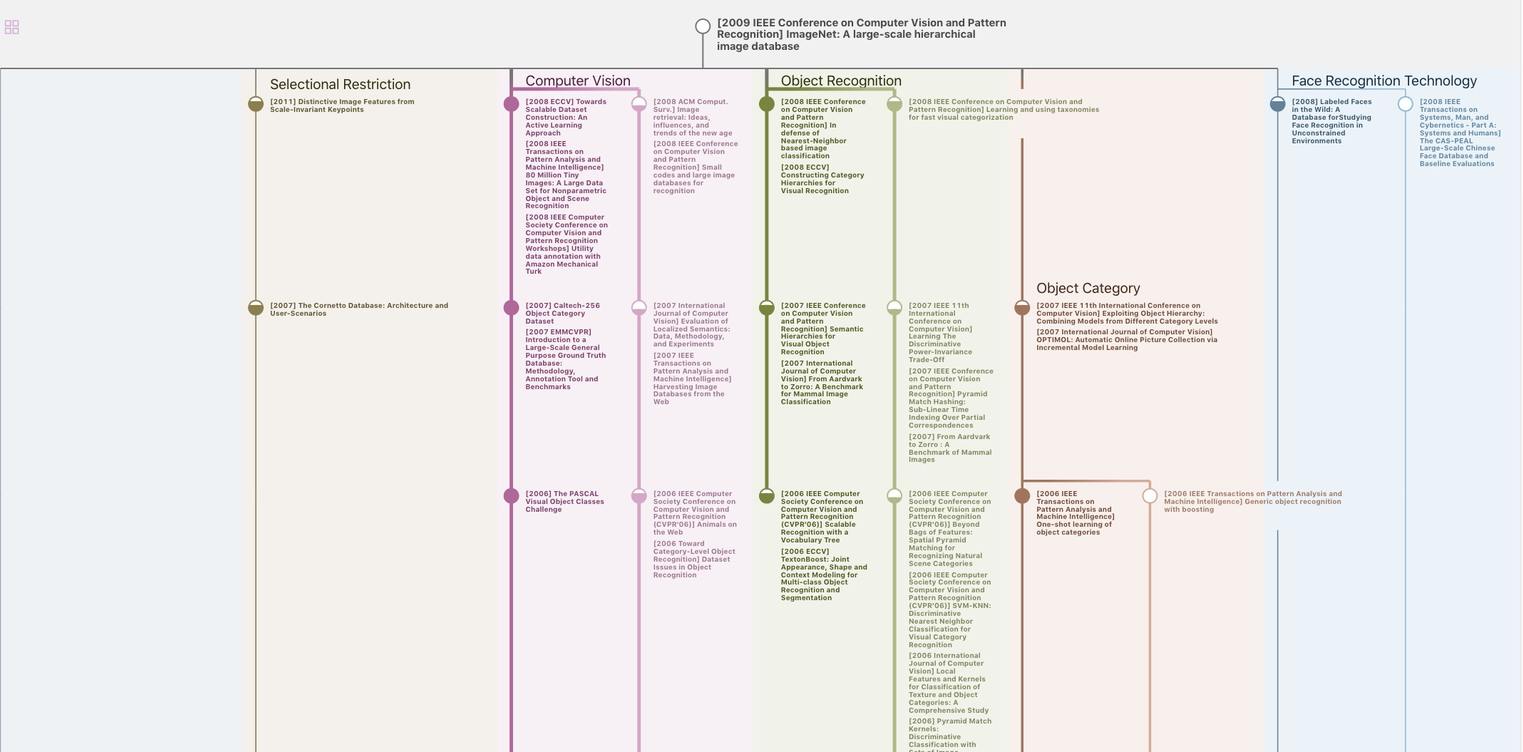
生成溯源树,研究论文发展脉络
Chat Paper
正在生成论文摘要