Local Similarity Matrix for Cysteine Disulfide Connectivity Prediction from Protein Sequences
IEEE/ACM Transactions on Computational Biology and Bioinformatics(2020)
摘要
AbstractAccurately predicting three dimensional protein structures from sequences would present us with targets for drugs via molecular dynamics that would treat cancer, viral infections, and neurological diseases. These treatments would have a far reaching impact to our economy, quality of life, and society. The goal of this research was to build a data mining framework to predict cysteine connectivity in proteins from the sequence and oxidation state of cysteines. Accurately predicting the cysteine bonding configuration improves the TM-Score, a quantitative measurement of protein structure prediction accuracy. We provided state of the art Qp and Qc on the PDBCYS and IVD-54 Datasets. Furthermore, we have produced a Local Similarity Matrix that compares favorably to the default PSSMs generated from PSI-Blast in a statistically significant way. Our Qp for SP39, PDBCYS, and IVD-54 were 90.6, 80.6, and 68.5, respectively.
更多查看译文
关键词
Cysteine disulfide connectivity,local similarity matrix,modeller,PDBCYS,position specific scoring matrix,PSSM,SP39
AI 理解论文
溯源树
样例
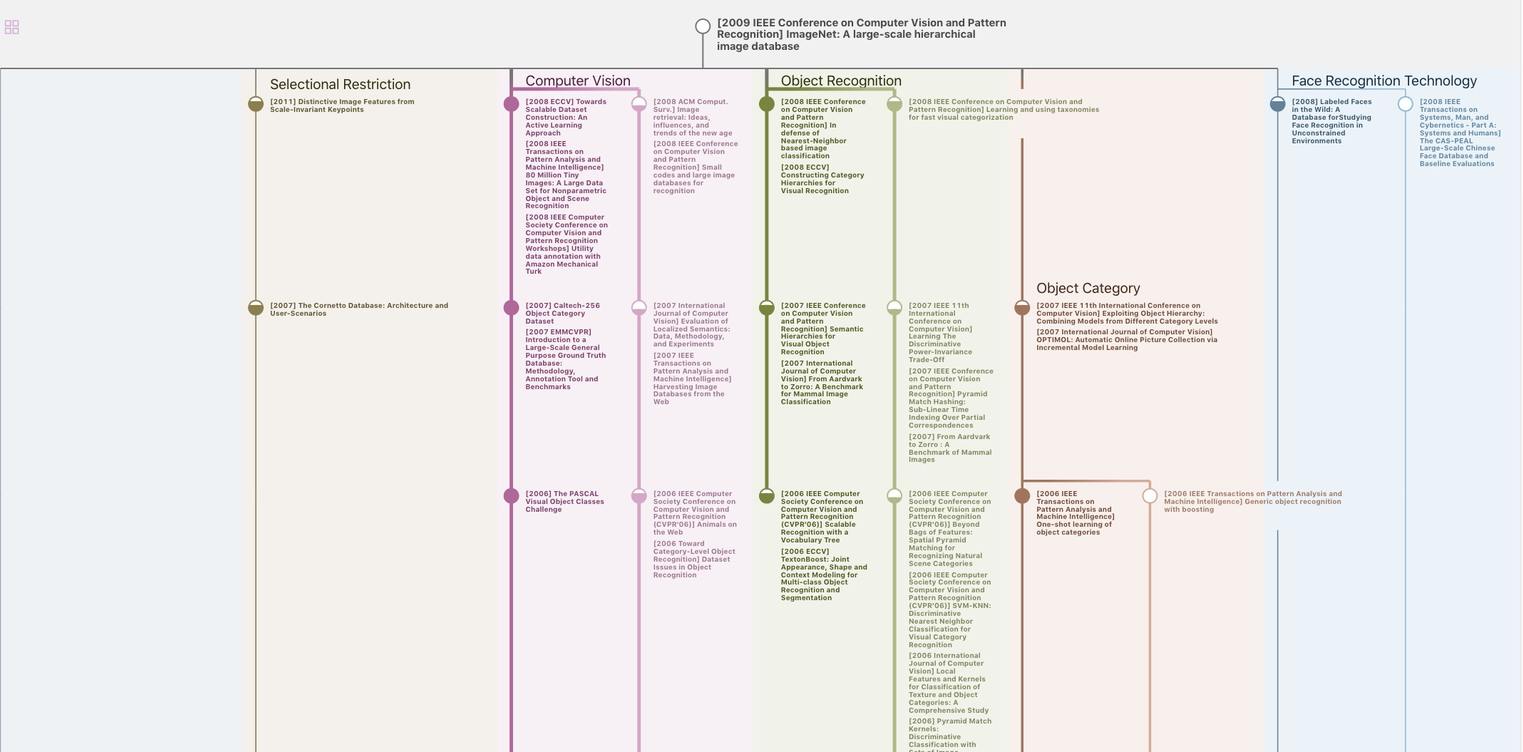
生成溯源树,研究论文发展脉络
Chat Paper
正在生成论文摘要