Intrinsically Motivated Self-Supervised Deep Sensorimotor Learning For Grasping
2018 IEEE/RSJ INTERNATIONAL CONFERENCE ON INTELLIGENT ROBOTS AND SYSTEMS (IROS)(2018)
摘要
Deep learning has been successful in a variety of applications that have high-dimensional state spaces such as object recognition, video games, and machine translation. Deep neural networks can automatically learn important features from high-dimensional state given large training datasets. However, the success of deep learning in robot systems in the real-world is limited due to the cost of obtaining these large datasets. To overcome this problem, we propose an information-theoretic, intrinsically motivated, self-labeling mechanism using closed-loop control states. Taking this approach biases exploration to informative interactions-as such, a robot requires much less training to achieve reliable performance. In this paper, we explore the impact such an approach has on learning how to grasp objects. We evaluate different intrinsic motivators present in the literature applied appropriately in our framework and discuss the benefits and drawbacks of each.
更多查看译文
关键词
high-dimensional state spaces,object recognition,video games,machine translation,deep neural networks,training datasets,deep learning,robot systems,closed-loop control states,motivated self-supervised deep sensorimotor learning,intrinsic motivators
AI 理解论文
溯源树
样例
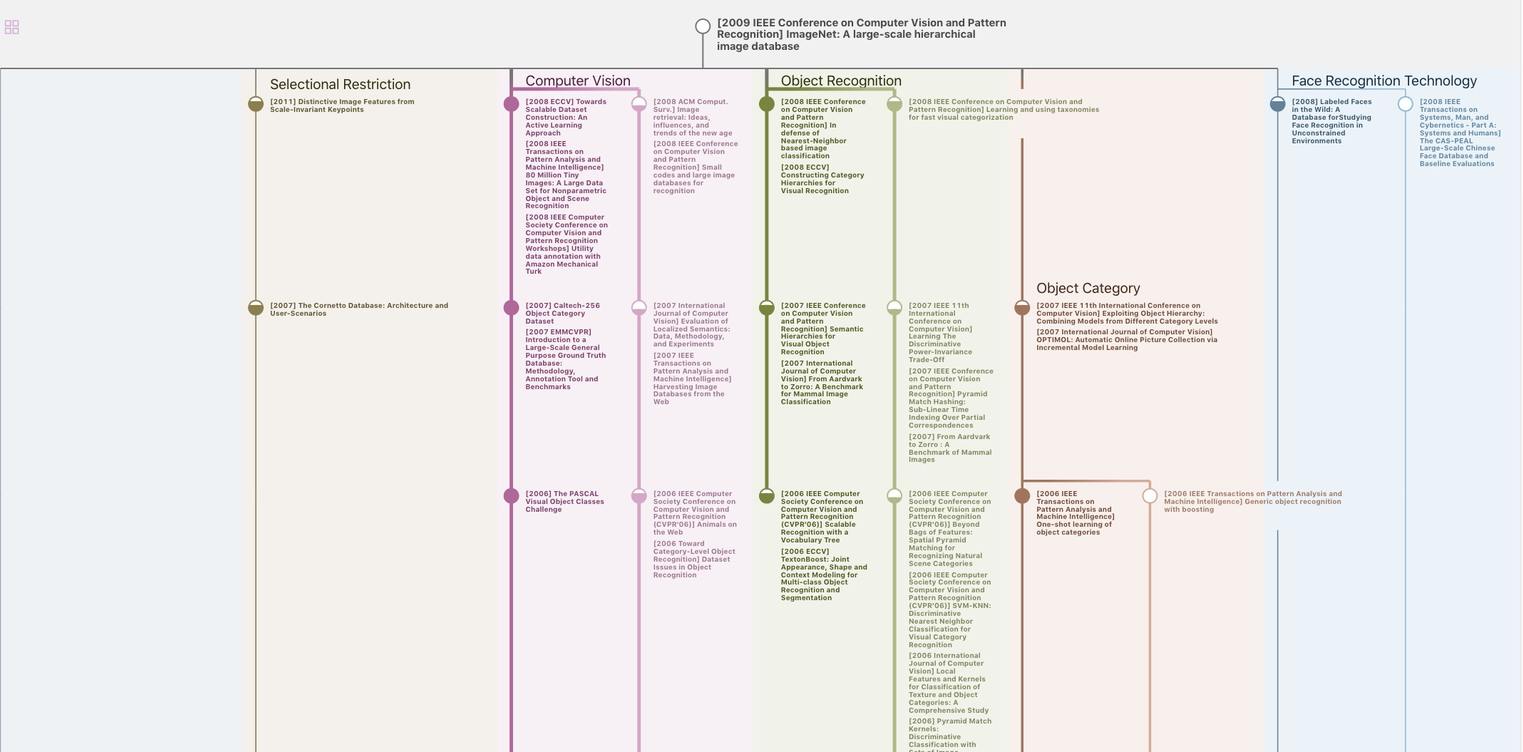
生成溯源树,研究论文发展脉络
Chat Paper
正在生成论文摘要