Tau-Safe (I, K)-Diversity Privacy Model For Sequential Publication With High Utility
IEEE ACCESS(2019)
摘要
Preserving privacy while maintaining high utility during sequential publication for data providers and data users in mathematical statistics, scientific researching, and organizations making decisions play an important role recently. The tau-safety model is the state-of-the-art model in sequential publication. However, it is based on the generalization technique, which has some drawbacks such as heavy information loss and difficulty of supporting marginal publication. Besides, the privacy of individuals is the major aspect that needs to be protected in privacy preserving data publishing. In this paper, to protect the privacy of individuals in sequential publication, we develop a new tau-safe (l, k)-diversity privacy model based on generalization and segmentation by record anonymity satisfying l-diversity and individual anonymity satisfying k-anonymity. This privacy model ensures that each record's signatures keep consistency or have no intersection in all releases. It can get high data utility while resisting the linking attacks due to arbitrary updates. In addition, it can also be applied to a dataset where individual has multiple records and arbitrary marginal publication. The results of our experiments show that the proposed privacy model achieves better anonymization quality and query accuracy in comparison with the m-invariance and tau-safety model in the sequential publication with arbitrary updates.
更多查看译文
关键词
Sequential publication,privacy,anonymization,bucketization,(l, k)-diversity
AI 理解论文
溯源树
样例
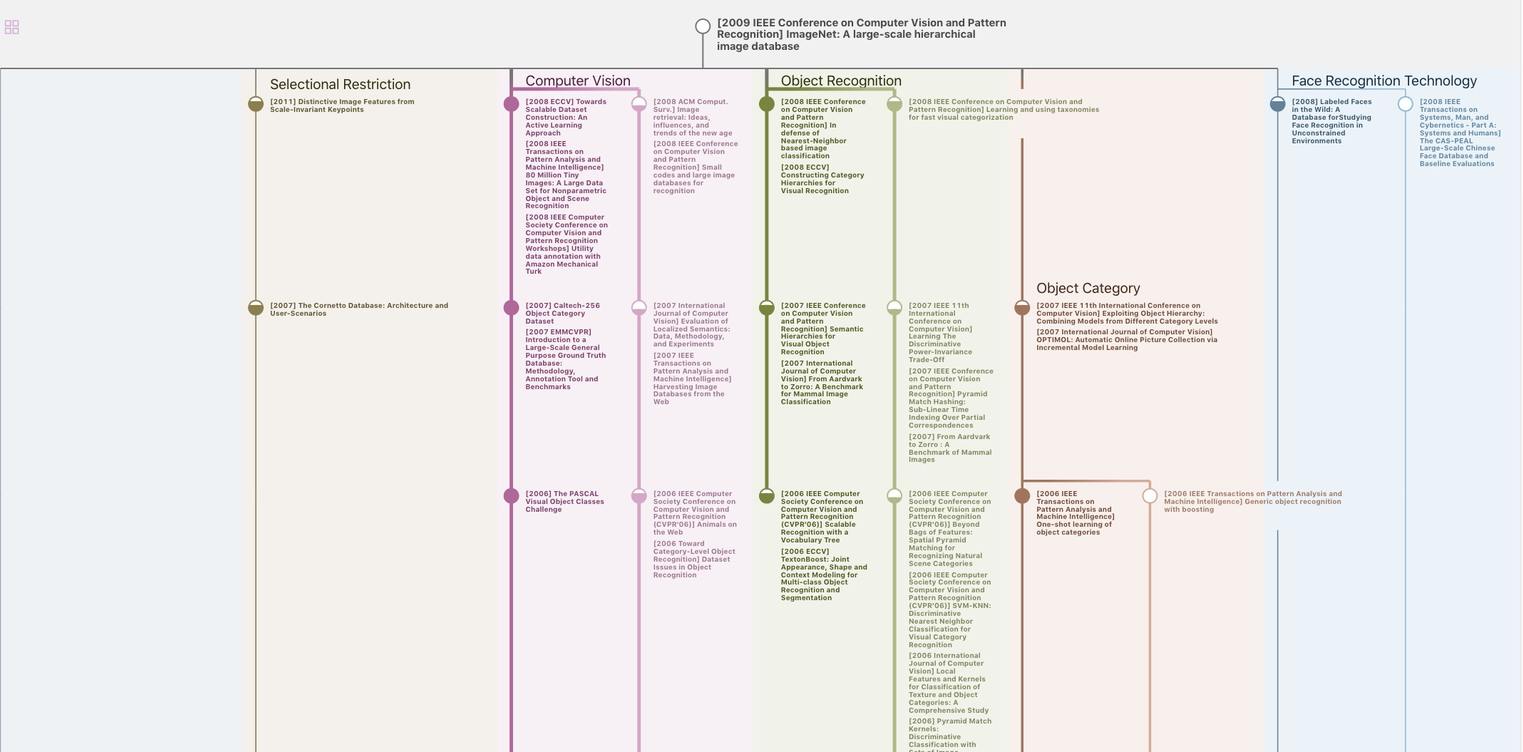
生成溯源树,研究论文发展脉络
Chat Paper
正在生成论文摘要