Advanced Heterogeneous Feature Fusion Machine Learning Models and Algorithms for Improving Indoor Localization.
SENSORS(2019)
摘要
In the era of the Internet of Things and Artificial Intelligence, the Wi-Fi fingerprinting-based indoor positioning system (IPS) has been recognized as the most promising IPS for various applications. Fingerprinting-based algorithms critically rely on a fingerprint database built from machine learning methods. However, currently methods are based on single-feature Received Signal Strength (RSS), which is extremely unstable in performance in terms of precision and robustness. The reason for this is that single feature machines cannot capture the complete channel characteristics and are susceptible to interference. The objective of this paper is to exploit the Time of Arrival (TOA) feature and propose a heterogeneous features fusion model to enhance the precision and robustness of indoor positioning. Several challenges are addressed: (1) machine learning models based on heterogeneous features, (2) the optimization of algorithms for high precision and robustness, and (3) computational complexity. This paper provides several heterogeneous features fusion-based localization models. Their effectiveness and efficiency are thoroughly compared with state-of-the-art methods.
更多查看译文
关键词
indoor localization,heterogeneous features fusion (HFF),machine learning,optimization
AI 理解论文
溯源树
样例
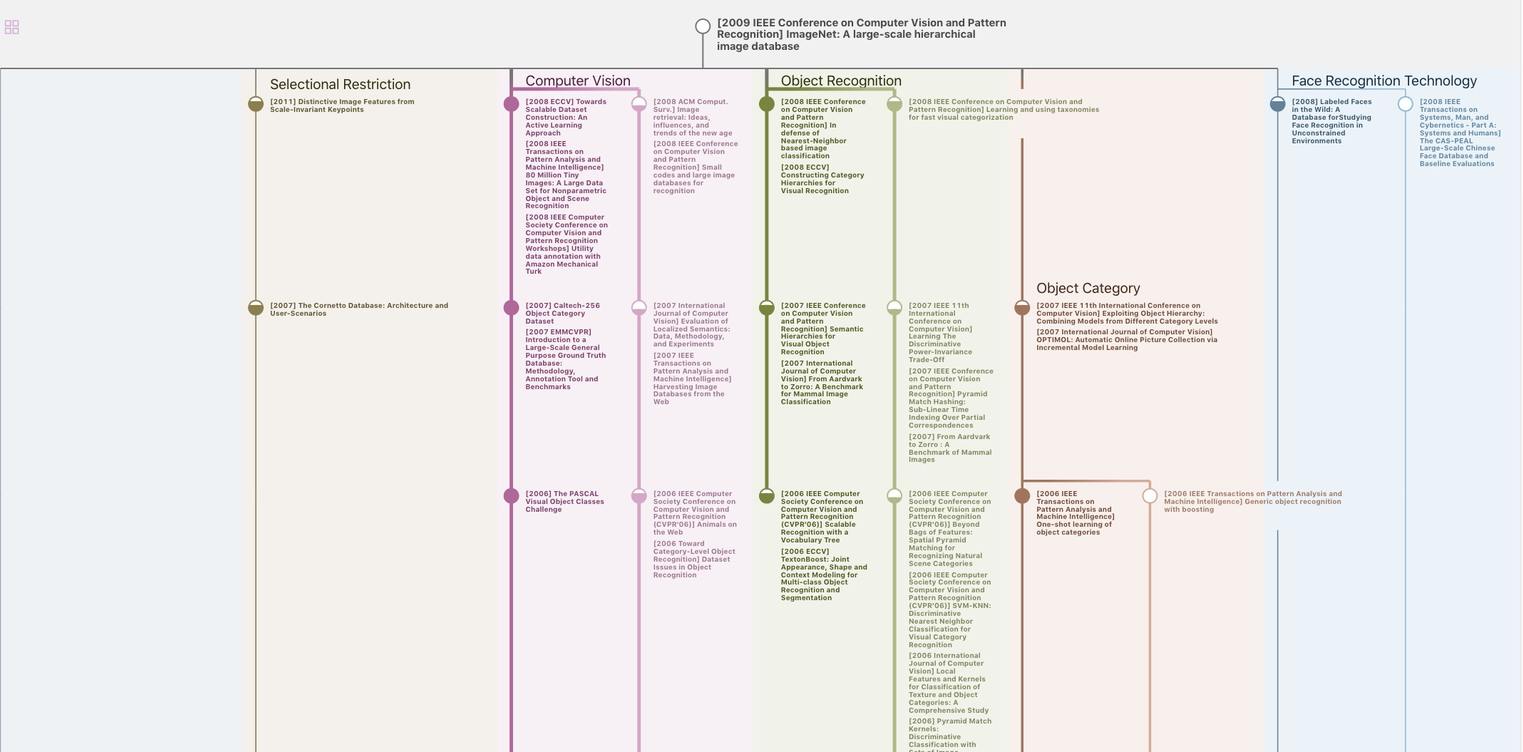
生成溯源树,研究论文发展脉络
Chat Paper
正在生成论文摘要