Memory-System Requirements For Convolutional Neural Networks
PROCEEDINGS OF THE INTERNATIONAL SYMPOSIUM ON MEMORY SYSTEMS (MEMSYS 2018)(2018)
摘要
Energy efficiency of the underlying memory systems is a huge issue in most neural network accelerator designs. It is imperative to understand the characteristics and behavior of data in the algorithm of neural networks to gain an insight into their impact on memory-systems. The current work studies such impacts of convolutional neural networks on the energy efficiency of memory-systems by analyzing the structuring of datasets in convolutional neural networks, their memory space and bandwidth requirements, the energy efficiency of their accesses in DDR memory systems versus 3D-stacked DRAM systems and the future direction of this area. Also, one possible architectural implementation using the near-data processing capabilities of highly parallel heterogeneous 3D-stacked DRAM chips of Micron's Hybrid Memory Cube is demonstrated which shows an improvement of above 90% in energy efficiency for acceleration of convolutional neural networks.
更多查看译文
关键词
Convolutional neural network, 3D-stacked memory, near-data processing, acceleration
AI 理解论文
溯源树
样例
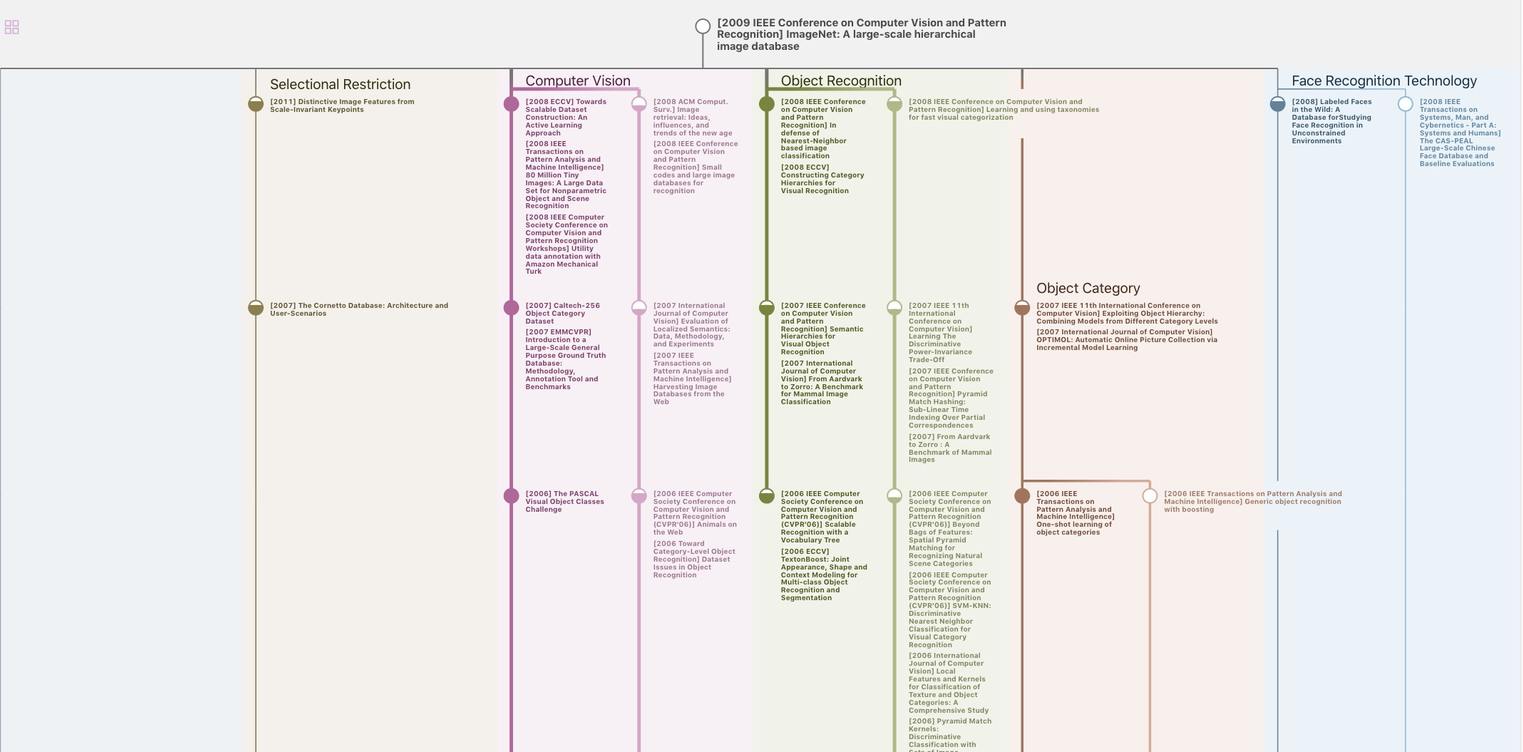
生成溯源树,研究论文发展脉络
Chat Paper
正在生成论文摘要