Learning Cheap and Novel Flight Itineraries
arXiv (Cornell University)(2018)
摘要
We consider the problem of efficiently constructing cheap and novel round trip flight itineraries by combining legs from different airlines. We analyse the factors that contribute towards the price of such itineraries and find that many result from the combination of just 30% of airlines and that the closer the departure of such itineraries is to the user's search date the more likely they are to be cheaper than the tickets from one airline. We use these insights to formulate the problem as a trade-off between the recall of cheap itinerary constructions and the costs associated with building them. We propose a supervised learning solution with location embeddings which achieves an AUC=80.48, a substantial improvement over simpler baselines. We discuss various practical considerations for dealing with the staleness and the stability of the model and present the design of the machine learning pipeline. Finally, we present an analysis of the model's performance in production and its impact on Skyscanner's users.
更多查看译文
关键词
flight,learning
AI 理解论文
溯源树
样例
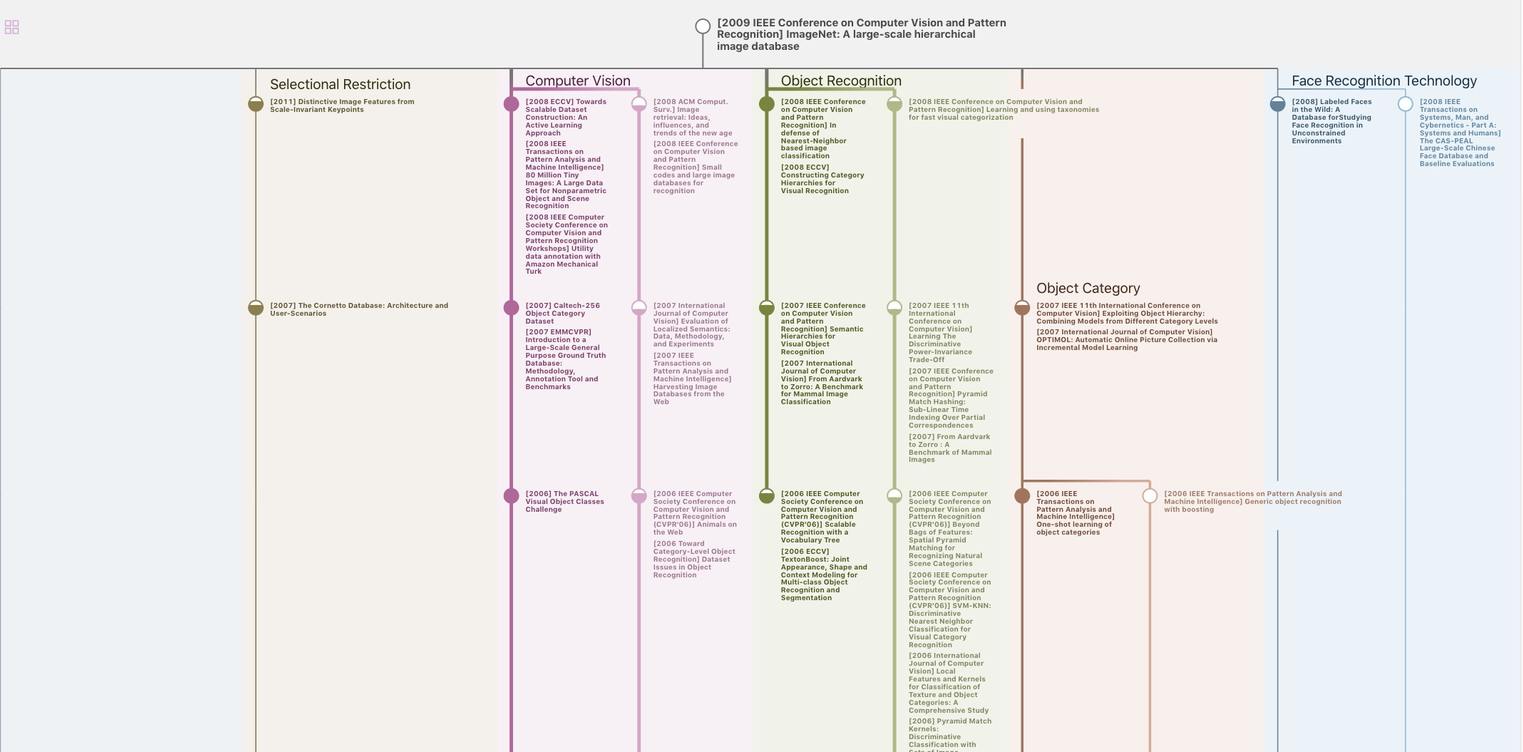
生成溯源树,研究论文发展脉络
Chat Paper
正在生成论文摘要