Visual Localization In Changing Environments Using Place Recognition Techniques
2018 24TH INTERNATIONAL CONFERENCE ON PATTERN RECOGNITION (ICPR)(2018)
摘要
This paper proposes a visual localization system combining Convolutional Neural Networks (CNNs) and sparse point features to estimate the 6-DOF pose of the robot. The challenges of visual localization across time lie in that the same place captured across time appears dramatically different due to different illumination and weather conditions, viewpoint variations and dynamic objects. In this paper, a novel CNN-based place recognition approach is proposed, which requires no time-consuming feature generation process and no task-specific training. Moreover, we demonstrate that the rich semantic context information obtained from place recognition can greatly improve the subsequent feature matching process for pose estimation. The semantic constraint performs much better than traditional Bag-of-Words based methods for establishing correspondences between the query image and the map. To evaluate the robustness of the algorithm, the proposed system is integrated into ORB-SLAM2 and verified on the data collected over various illumination and weather conditions. Extensive experimental results show that even with weak ORB descriptors, the proposed system can significantly improve the success rate of localization under severe appearance changes.
更多查看译文
关键词
feature matching process,convolutional neural networks,CNN-based place recognition approach,sparse point features,visual localization system,place recognition techniques,Bag-of-Words based methods,semantic constraint,pose estimation,rich semantic context information,task-specific training,time-consuming feature generation process,dynamic objects
AI 理解论文
溯源树
样例
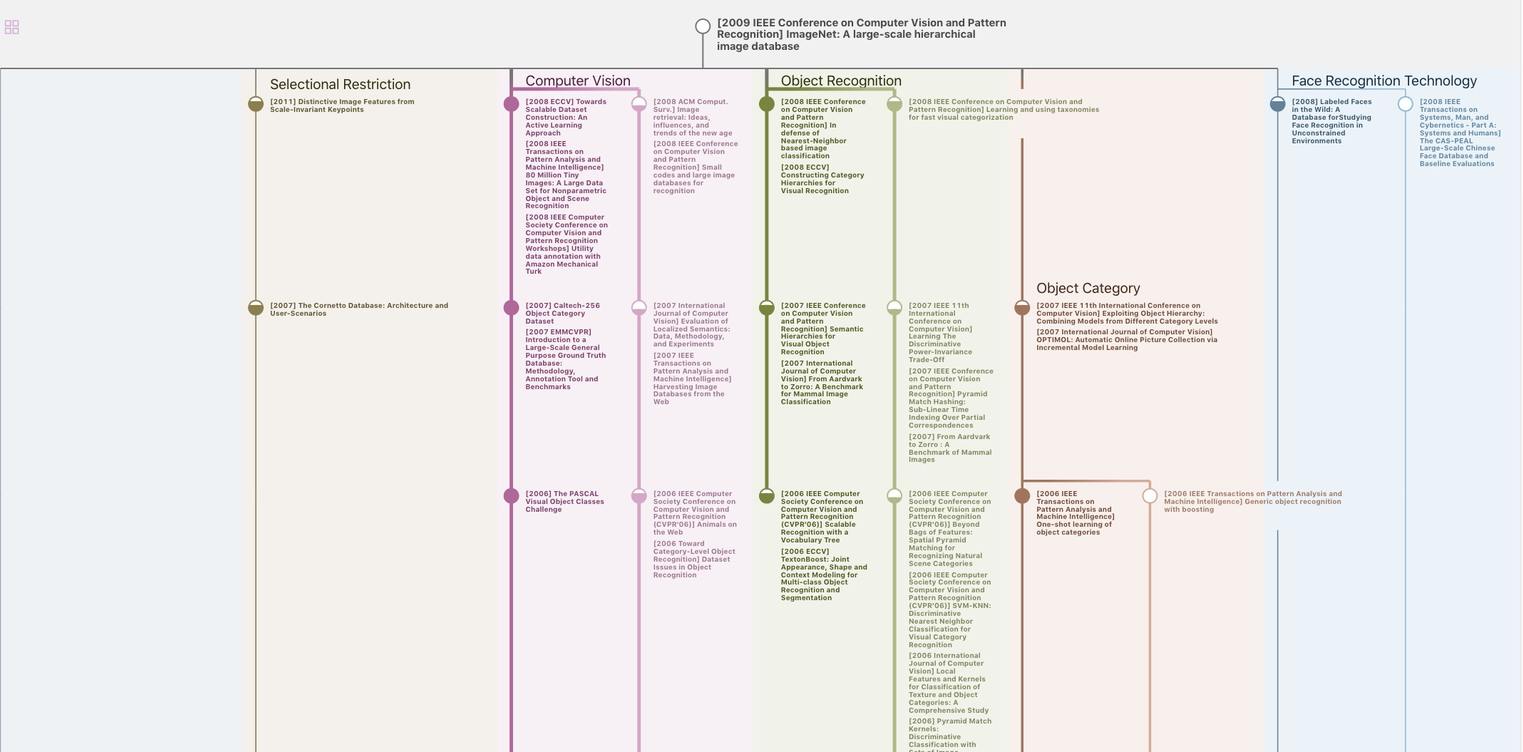
生成溯源树,研究论文发展脉络
Chat Paper
正在生成论文摘要