A Four-Stage Hybrid Feature Subset Selection Approach for Network Traffic Classification Based on Full Coverage.
SpaCCS(2018)
摘要
There is significant interest in network management and security to classify traffic flows. As the essential step for machine learning based traffic classification, feature subset selection is often used to realize dimension reduction and redundant information decrease. A four-stage hybrid feature subset selection method is proposed to improve the classification performance of hybrid methods at low evaluation consumption. The proposed algorithm is designed to dispose features in the level of block and evaluate every feature even the remaining ones which cannot provide much information by themselves to use the interactions among all of them. Additionally, a wrapper-based selection is designed in the last stage to further remove the redundant features. The performances are examined by two groups of experiments. Our theoretical analysis and experimental observations reveal that the proposed method selects feature subset with improved classification performance on every index while depleting fewer evaluations. Moreover, the evaluation consumption can keep at a low and stable level with different size of block.
更多查看译文
关键词
Full coverage, Machine learning, Hybrid feature subset selection, Network traffic classification, Network management
AI 理解论文
溯源树
样例
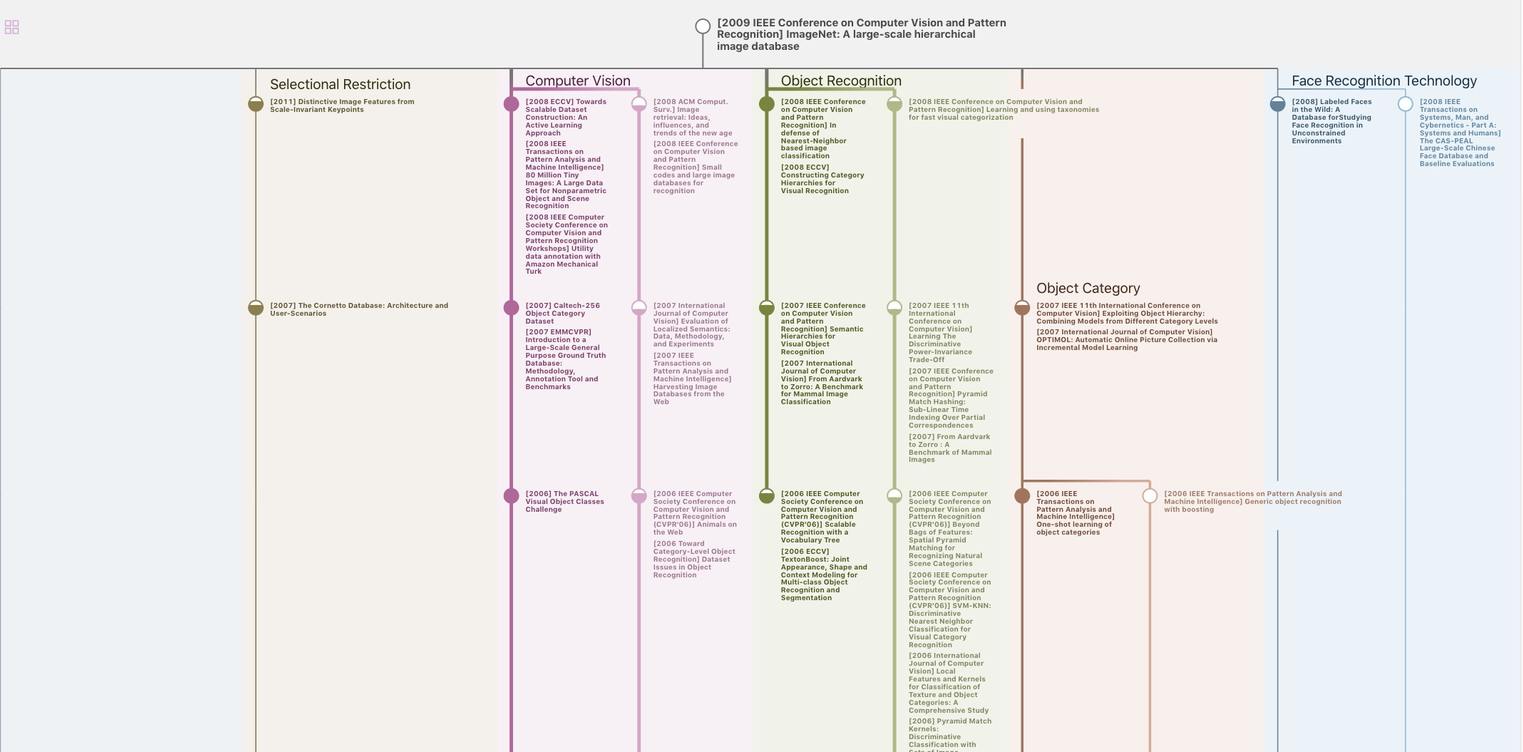
生成溯源树,研究论文发展脉络
Chat Paper
正在生成论文摘要