A network-pathway based module identification for predicting the prognosis of ovarian cancer patients
Journal of ovarian research(2016)
摘要
Background This study aimed to screen multiple genes biomarkers based on gene expression data for predicting the survival of ovarian cancer patients. Methods Two microarray data of ovarian cancer samples were collected from The Cancer Genome Atlas (TCGA) database. The data in the training set were used to construct Reactome functional interactions network, which then underwent Markov clustering, supervised principal components, Cox proportional hazard model to screen significantly prognosis related modules. The distinguishing ability of each module for survival was further evaluated by the testing set. Gene Ontology (GO) functional and pathway annotations were performed to identify the roles of genes in each module for ovarian cancer. Results The network based approach identified two 7-gene functional interaction modules (31: DCLRE1A , EXO1 , KIAA0101 , KIN , PCNA , POLD3 , POLD2 ; 35: DKK3 , FABP3 , IRF1 , AIM2 , GBP1 , GBP2 , IRF2 ) that are associated with prognosis of ovarian cancer patients. These network modules are related to DNA repair, replication, immune and cytokine mediated signaling pathways. Conclusions The two 7-gene expression signatures may be accurate predictors of clinical outcome in patients with ovarian cancer and has the potential to develop new therapeutic strategies for ovarian cancer patients.
更多查看译文
关键词
Ovarian cancer,Reactome functional interactions,Markov clustering,Supervised principal components,Prognosis
AI 理解论文
溯源树
样例
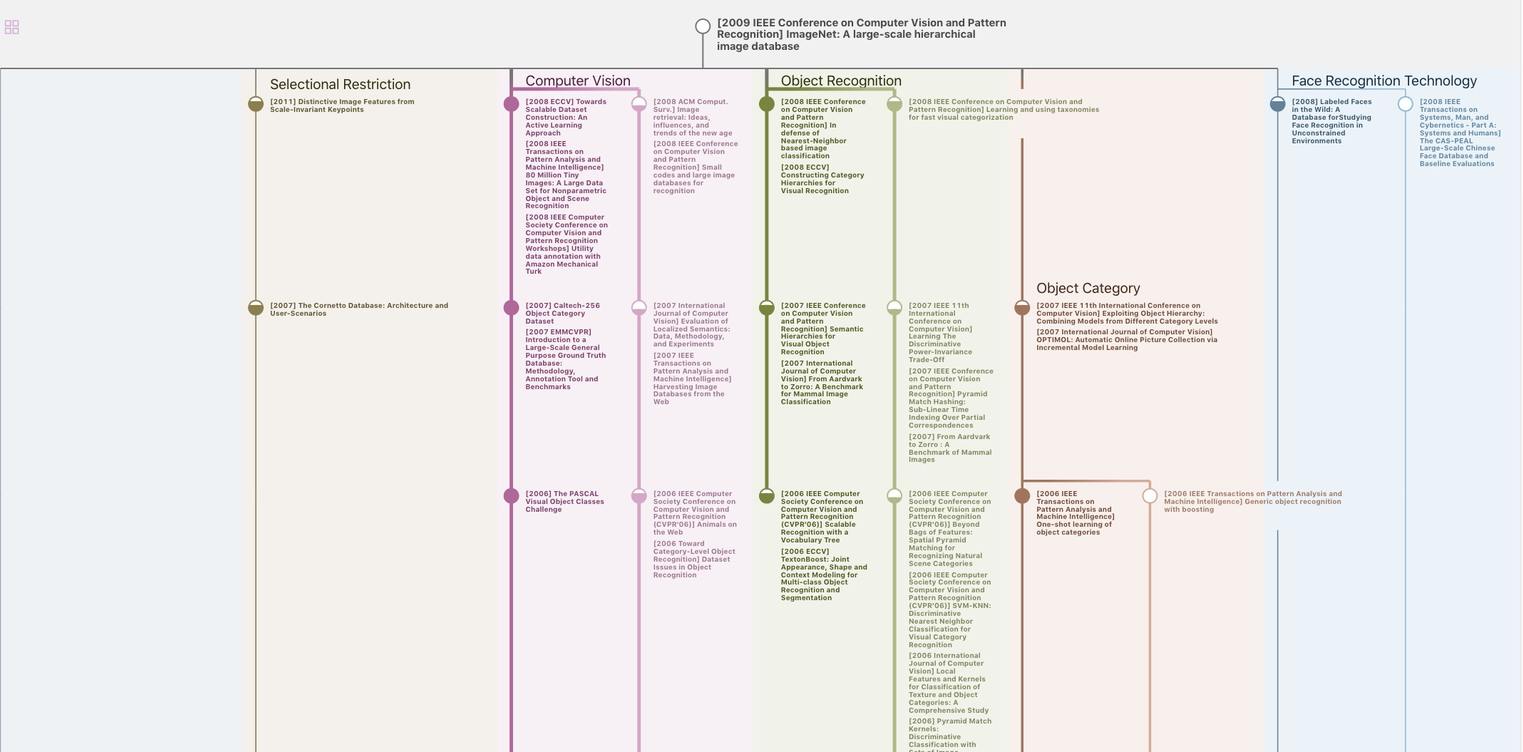
生成溯源树,研究论文发展脉络
Chat Paper
正在生成论文摘要