DVS Benchmark Datasets for Object Tracking, Action Recognition, and Object Recognition.
FRONTIERS IN NEUROSCIENCE(2016)
摘要
Benchmarks have played a vital role in the advancement of visual object recognition and other fields of computer vision (LeCun et al., 1998; Deng et al., 2009;). The challenges posed by these standard datasets have helped identify and overcome the shortcomings of existing approaches, and have led to great advances of the state of the art. Even the recent massive increase of interest in deep learning methods can be attributed to their success in difficult benchmarks such as ImageNet (Krizhevsky et al., 2012; LeCun et al., 2015). Neuromorphic vision uses silicon retina sensors such as the dynamic vision sensor (DVS; Lichtsteiner et al., 2008). These sensors and their DAVIS (Dynamic and Active-pixel Vision Sensor) and ATIS (Asynchronous Time-based Image Sensor) derivatives (Brandli et al., 2014; Posch et al., 2014) are inspired by biological vision by generating streams of asynchronous events indicating local log-intensity brightness changes. They thereby greatly reduce the amount of data to be processed, and their dynamic nature makes them a good fit for domains such as optical flow, object tracking, action recognition, or dynamic scene understanding. Compared to classical computer vision, neuromorphic vision is a younger and much smaller field of research, and lacks benchmarks, which impedes the progress of the field. To address this we introduce the largest event-based vision benchmark dataset published to date, hoping to satisfy a growing demand and stimulate challenges for the community. In particular, the availability of such benchmarks should help the development of algorithms processing event-based vision input, allowing a direct fair comparison of different approaches. We have explicitly chosen mostly dynamic vision tasks such as action recognition or tracking, which could benefit from the strengths of neuromorphic vision sensors, although algorithms that exploit these features are largely missing.A major reason for the lack of benchmarks is that currently neuromorphic vision sensors are only available as RD see Tan et al. (2015) for an informative review. Unlabeled DVS data was made available around 2007 in the jAER project1 and was used for development of spike timing-based unsupervised feature learning e.g., in Bichler et al. (2012). The first labeled and published event-based neuromorphic vision sensor benchmarks were created from the MNIST digit recognition dataset by jiggling the image on the screen (see Serrano-Gotarredona and Linares-Barranco, 2015 for an informative history) and later to reduce frame artifacts by jiggling the camera view with a pan-tilt unit (Orchard et al., 2015). These datasets automated the scene movement necessary to generate DVS output from the static images, and will be an important step forward for evaluating neuromorphic object recognition systems such as spiking deep networks (Perez-Carrasco et al., 2013; Ou0027Connor et al., 2013; Cao et al., 2014; Diehl et al., 2015), which so far have been tested mostly on static image datasets converted into Poisson spike trains. But static image recognition is not the ideal use case for event-based vision sensors that are designed for dynamic scenes. Recently several additional DVS datasets were made available in the Frontiers research topic “Benchmarks and Challenges for Neuromorphic Engineering”2; in particular for navigation using multiple sensor modalities (Barranco et al., 2016) and for developing and benchmarking DVS and DAVIS optical flow methods (Rueckauer and Delbruck, 2016).This data report summarizes a new benchmark dataset in which we converted established visual video benchmarks for object tracking, action recognition and object recognition into spiking neuromorphic datasets, recorded with the DVS output (Lichtsteiner et al., 2008) of a DAVIS camera (Berner et al., 2013; Brandli et al., 2014). This report presents our approach for sensor calibration and capture of frame-based videos into neuromorphic vision datasets with minimal human intervention. We converted four widely used dynamic datasets: the VOT Challenge 2015 Dataset (Kristan et al., 2016), TrackingDataset3, the UCF-50 Action Recognition Dataset (Reddy and Shah, 2012), and the Caltech-256 Object Category Dataset (Griffin et al., 2006). We conclude with statistics and summaries of the datasets.
更多查看译文
关键词
neuromorphic,event-based vision,AER,benchmarks,DVS,action recognition,object tracking,object recognition
AI 理解论文
溯源树
样例
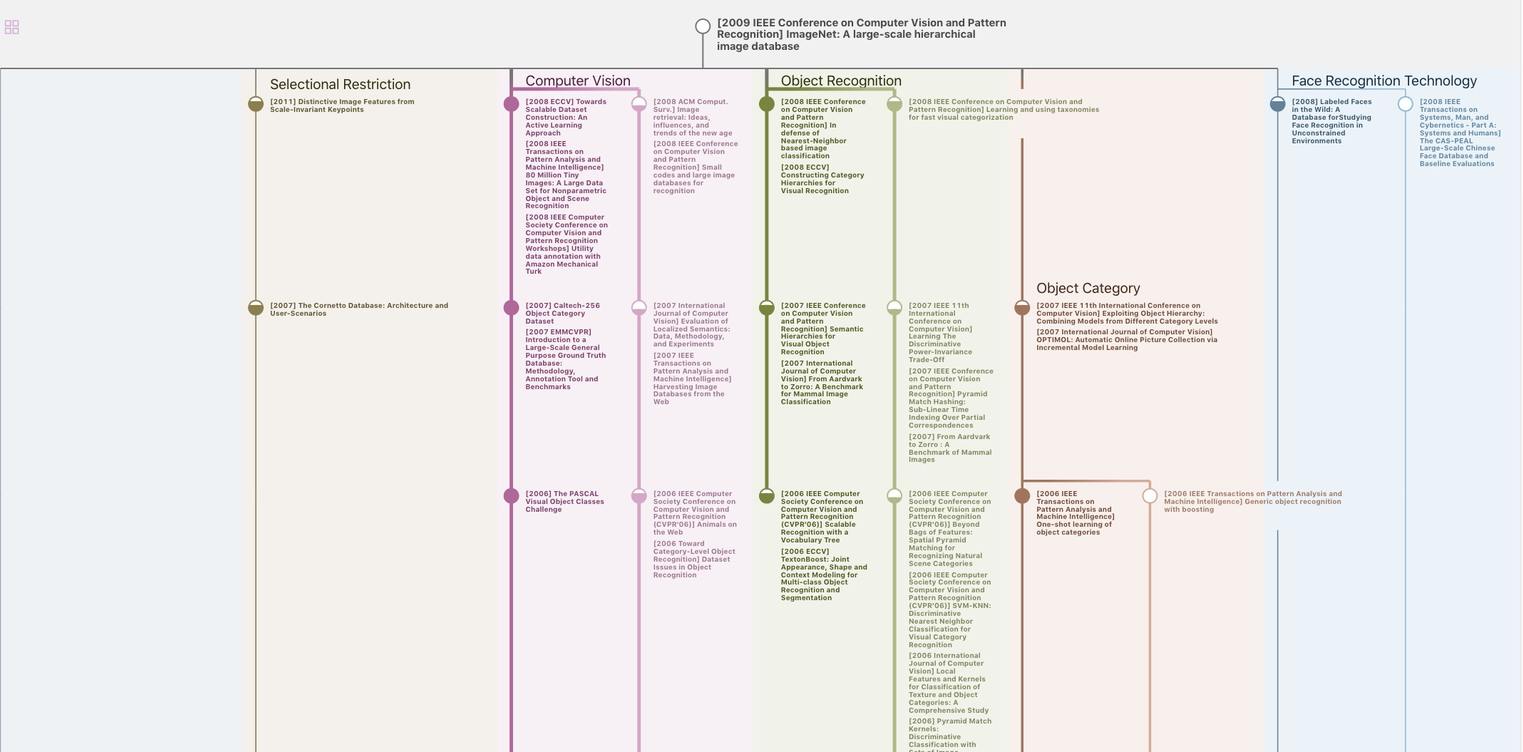
生成溯源树,研究论文发展脉络
Chat Paper
正在生成论文摘要