One-for-All: Grouped Variation Network-Based Fractional Interpolation in Video Coding
Periodicals(2019)
摘要
AbstractFractional interpolation is used to provide sub-pixel level references for motion compensation in the interprediction of video coding, which attempts to remove temporal redundancy in video sequences. Traditional handcrafted fractional interpolation filters face the challenge of modeling discontinuous regions in videos, while existing deep learning-based methods are either designed for a single quantization parameter (QP), only generating half-pixel samples, or need to train a model for each sub-pixel position. In this paper, we present a one-for-all fractional interpolation method based on a grouped variation convolutional neural network (GVCNN). Our method can deal with video frames coded using different QPs and is capable of generating all sub-pixel positions at one sub-pixel level. Also, by predicting variations between integer-position pixels and sub-pixels, our network offers more expressive power. Moreover, we perform specific measurements in training data generation to simulate practical situations in video coding, including blurring the down-sampled sub-pixel samples to avoid aliasing effects and coding integer pixels to simulate reconstruction errors. In addition, we analyze the impact of the size of blur kernels theoretically. Experimental results verify the efficiency of GVCNN. Compared with HEVC, our method achieves 2.2% in bit saving on average and up to 5.2% under low-delay P configuration.
更多查看译文
关键词
High efficient video coding (HEVC), fractional interpolation, convolutional neural network (CNN), grouped variation network
AI 理解论文
溯源树
样例
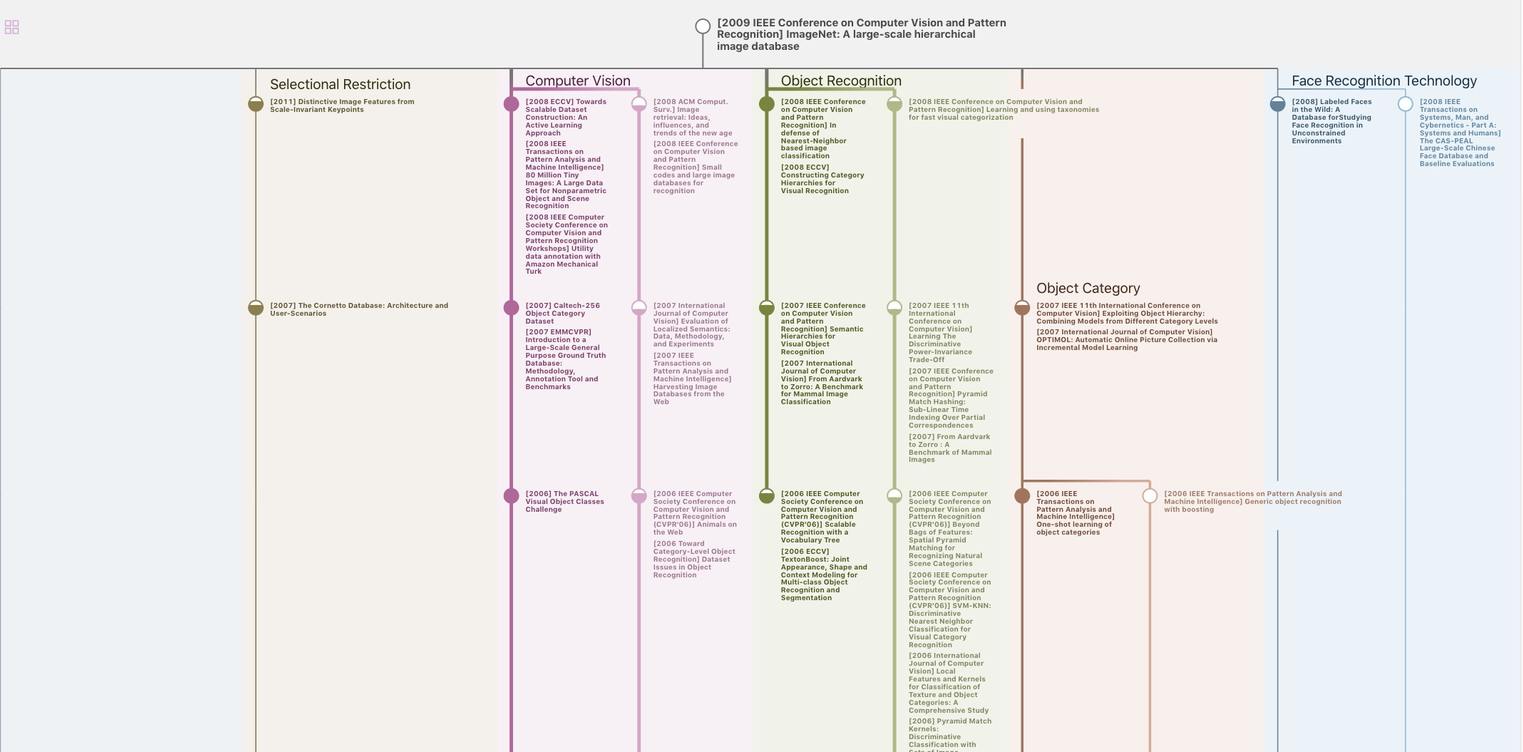
生成溯源树,研究论文发展脉络
Chat Paper
正在生成论文摘要