PReP: Path-Based Relevance from a Probabilistic Perspective in Heterogeneous Information Networks.
KDD'17: PROCEEDINGS OF THE 23RD ACM SIGKDD INTERNATIONAL CONFERENCE ON KNOWLEDGE DISCOVERY AND DATA MINING(2017)
摘要
As a powerful representation paradigm for networked and multi-typed data, the heterogeneous information network (HIN) is ubiquitous. Meanwhile, defining proper relevance measures has always been a fundamental problem and of great pragmatic importance for network mining tasks. Inspired by our probabilistic interpretation of existing path-based relevance measures, we propose to study HIN relevance from a probabilistic perspective. We also identify, from real-world data, and propose to model cross-meta-path synergy, which is a characteristic important for defining path-based HIN relevance and has not been modeled by existing methods. A generative model is established to derive a novel path-based relevance measure, which is data-driven and tailored for each HIN. We develop an inference algorithm to find the maximum a posteriori (MAP) estimate of the model parameters, which entails non-trivial tricks. Experiments on two real-world datasets demonstrate the effectiveness of the proposed model and relevance measure.
更多查看译文
关键词
Heterogeneous information networks,graph mining,meta-paths,relevance measures
AI 理解论文
溯源树
样例
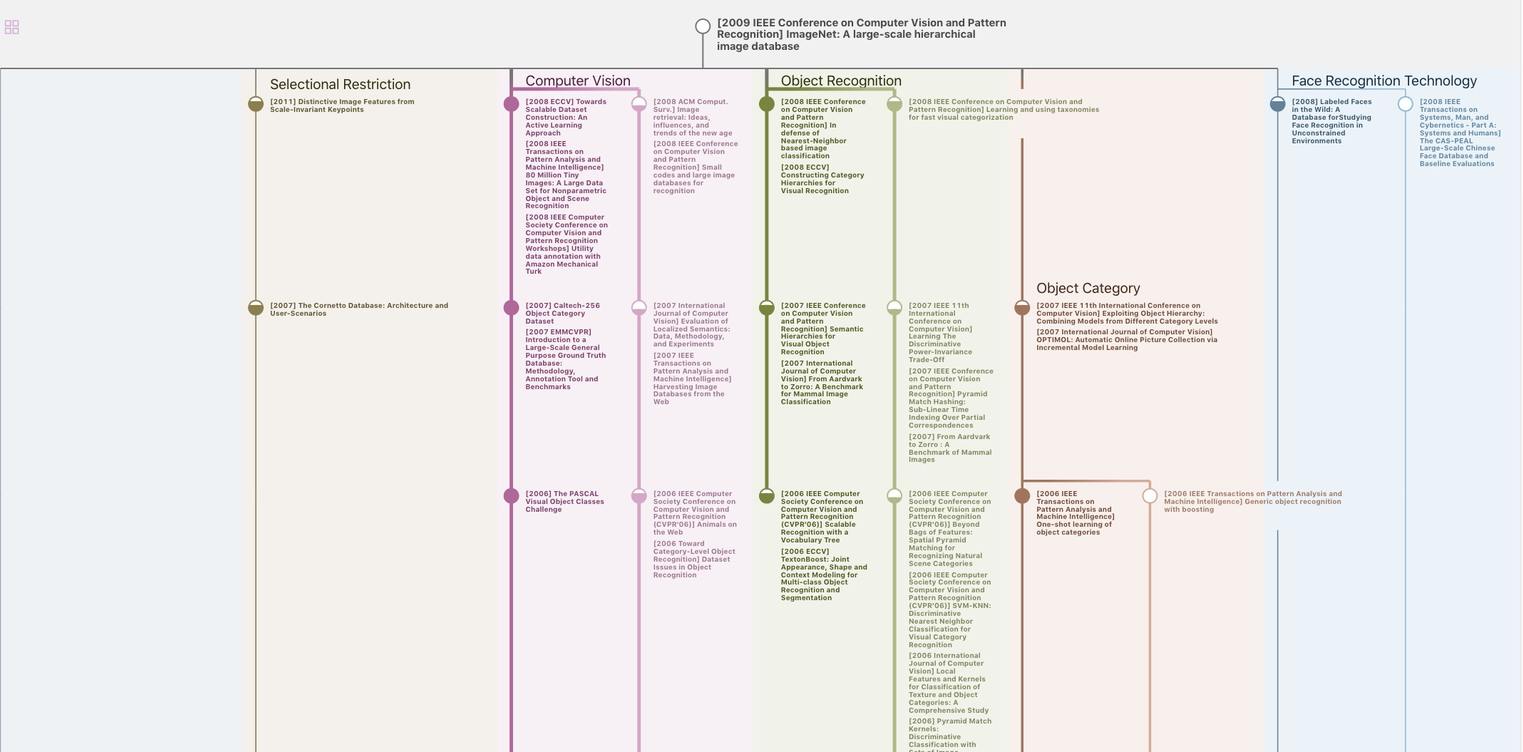
生成溯源树,研究论文发展脉络
Chat Paper
正在生成论文摘要