Learning Pose-Aware Models for Pose-Invariant Face Recognition in the Wild.
IEEE Trans. Pattern Anal. Mach. Intell.(2019)
摘要
We propose a method designed to push the frontiers of unconstrained face recognition in the wild with an emphasis on extreme out-of-plane pose variations. Existing methods either expect a single model to learn pose invariance by training on massive amounts of data or else normalize images by aligning faces to a single frontal pose. Contrary to these, our method is designed to explicitly tackle pose variations. Our proposed Pose-Aware Models (PAM) process a face image using several pose-specific, deep convolutional neural networks (CNN). 3D rendering is used to synthesize multiple face poses from input images to both train these models and to provide additional robustness to pose variations at test time. Our paper presents an extensive analysis on the IJB-A benchmark, evaluating the effects that landmark detection accuracy, CNN layer selection, and pose model selection all have on the performance of the recognition pipeline. It further provides comparative evaluations on the IARPA Janus Benchmarks A (IJB-A) and the PIPA dataset. These tests show that our approach outperforms existing methods, even surprisingly matching the accuracy of methods that were specifically fine-tuned to the target dataset. Parts of this work previously appeared in [1] and [2].
更多查看译文
关键词
Face,Three-dimensional displays,Benchmark testing,Face recognition,Training,Solid modeling,Rendering (computer graphics)
AI 理解论文
溯源树
样例
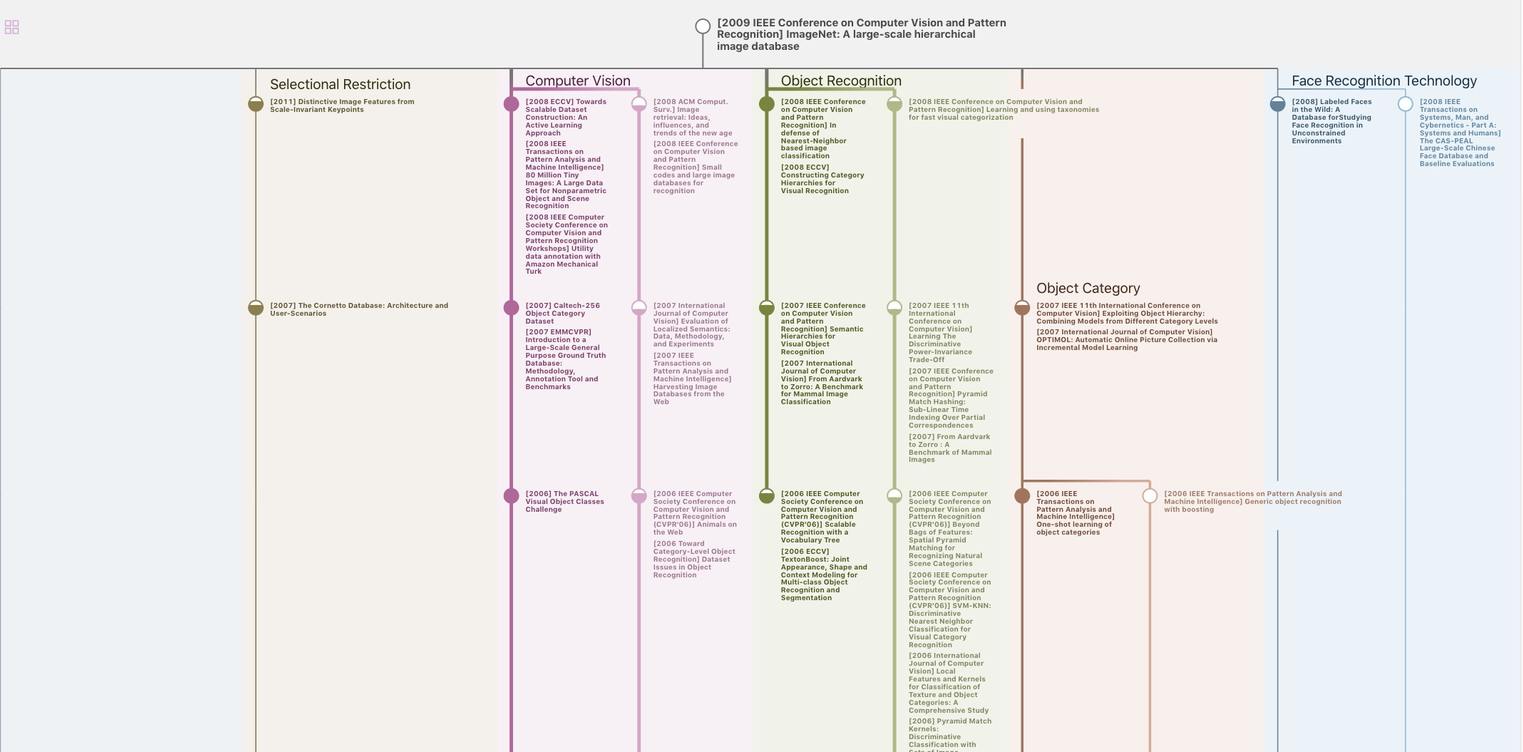
生成溯源树,研究论文发展脉络
Chat Paper
正在生成论文摘要