Geometric Deep Learning Autonomously Learns Chemical Features That Outperform Those Engineered by Domain Experts.
MOLECULAR PHARMACEUTICS(2018)
摘要
Artificial Intelligence has advanced at an unprecedented pace, backing recent breakthroughs in natural language processing, speech recognition, and computer vision: domains where the data is euclidean in nature. More recently, considerable progress has been made in engineering deep-learning architectures that can accept non-Euclidean data such as graphs and manifolds: geometric deep learning. This progress is of considerable interest to the drug discovery community, as molecules can naturally be represented as graphs, where atoms are nodes and bonds are edges. In this work, we explore the performance of geometric deep-learning methods in the context of drug discovery, comparing machine learned features against the domain expert engineered features that are mainstream in the pharmaceutical industry.
更多查看译文
关键词
artifical intelligence,geometric deep learning,drug discovery,pharmaceutics
AI 理解论文
溯源树
样例
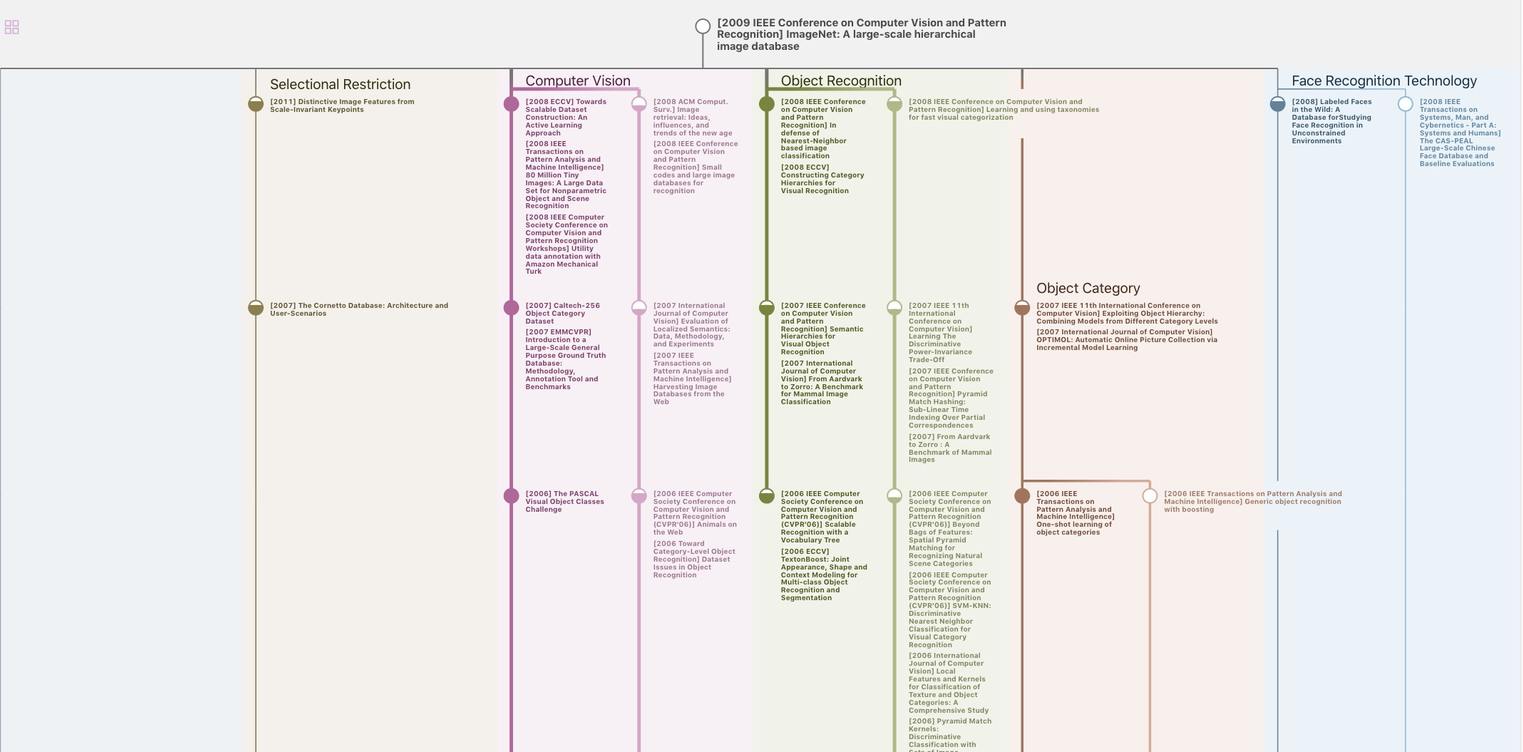
生成溯源树,研究论文发展脉络
Chat Paper
正在生成论文摘要