Comparing vector-based and Bayesian memory models using large-scale datasets: User-generated hashtag and tag prediction on Twitter and Stack Overflow.
PSYCHOLOGICAL METHODS(2016)
摘要
The growth of social media and user-created content on online sites provides unique opportunities to study models of human declarative memory. By framing the task of choosing a hashtag for a tweet and tagging a post on Stack Overflow as a declarative memory retrieval problem, 2 cognitively plausible declarative memory models were applied to millions of posts and tweets and evaluated on how accurately they predict a user's chosen tags. An ACT-R based Bayesian model and a random permutation vector-based model were tested on the large data sets. The results show that past user behavior of tag use is a strong predictor of future behavior. Furthermore, past behavior was successfully incorporated into the random permutation model that previously used only context. Also, ACT-R's attentional weight term was linked to an entropy-weighting natural language processing method used to attenuate high-frequency words (e.g., articles and prepositions). Word order was not found to be a strong predictor of tag use, and the random permutation model performed comparably to the Bayesian model without including word order. This shows that the strength of the random permutation model is not in the ability to represent word order, but rather in the way in which context information is successfully compressed. The results of the large-scale exploration show how the architecture of the 2 memory models can be modified to significantly improve accuracy, and may suggest task-independent general modifications that can help improve model fit to human data in a much wider range of domains.
更多查看译文
关键词
ACT-R declarative memory theory,vector-based models,LSA,machine learning
AI 理解论文
溯源树
样例
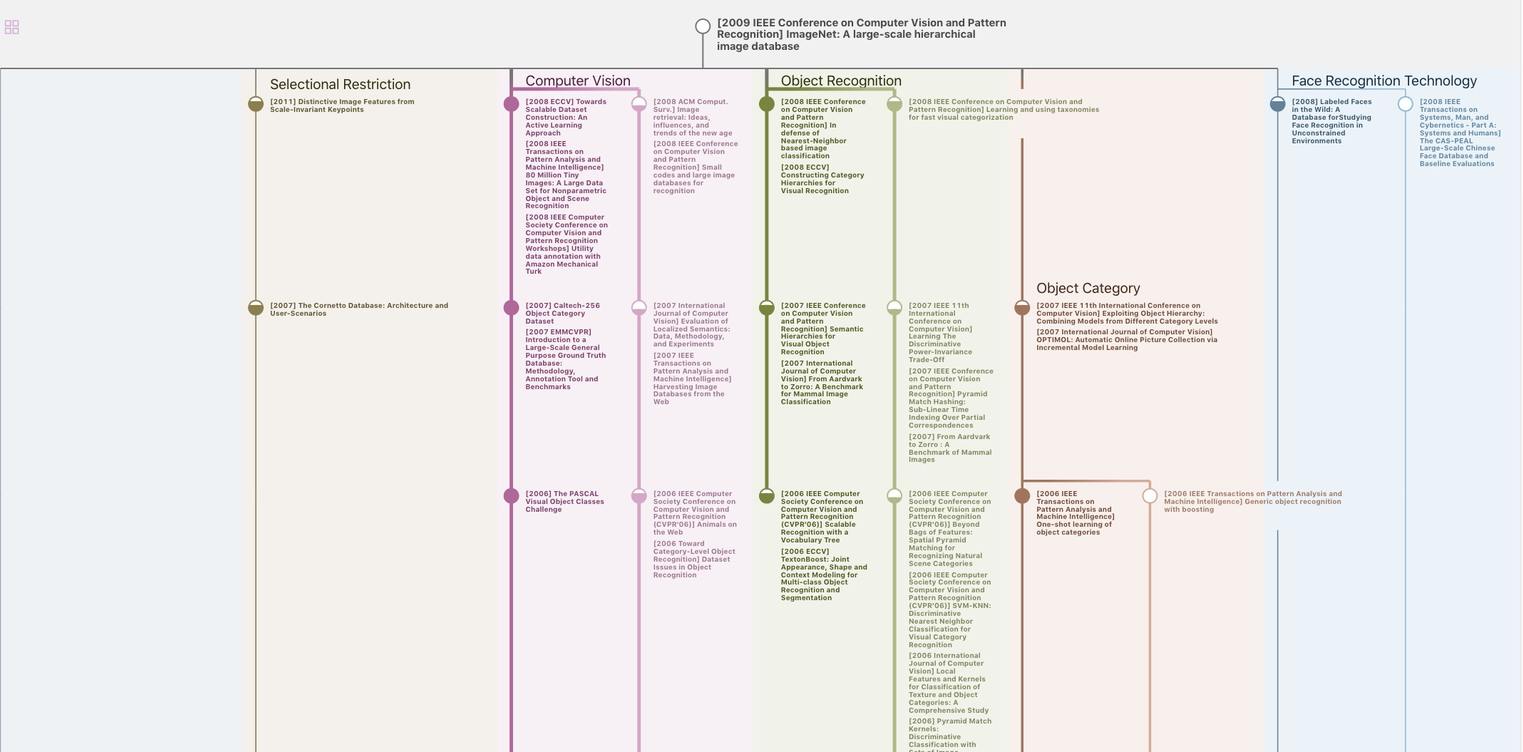
生成溯源树,研究论文发展脉络
Chat Paper
正在生成论文摘要