Comparison of Model Averaging and Model Selection in Dose Finding Trials Analyzed by Nonlinear Mixed Effect Models
The AAPS journal(2018)
摘要
In drug development, pharmacometric approaches consist in identifying via a model selection (MS) process the model structure that best describes the data. However, making predictions using a selected model ignores model structure uncertainty, which could impair predictive performance. To overcome this drawback, model averaging (MA) takes into account the uncertainty across a set of candidate models by weighting them as a function of an information criterion. Our primary objective was to use clinical trial simulations (CTSs) to compare model selection (MS) with model averaging (MA) in dose finding clinical trials, based on the AIC information criterion. A secondary aim of this analysis was to challenge the use of AIC by comparing MA and MS using five different information criteria. CTSs were based on a nonlinear mixed effect model characterizing the time course of visual acuity in wet age-related macular degeneration patients. Predictive performances of the modeling approaches were evaluated using three performance criteria focused on the main objectives of a phase II clinical trial. In this framework, MA adequately described the data and showed better predictive performance than MS, increasing the likelihood of accurately characterizing the dose-response relationship and defining the minimum effective dose. Moreover, regardless of the modeling approach, AIC was associated with the best predictive performances.
更多查看译文
关键词
dose-response relationship,model averaging,model selection,nonlinear mixed effect models
AI 理解论文
溯源树
样例
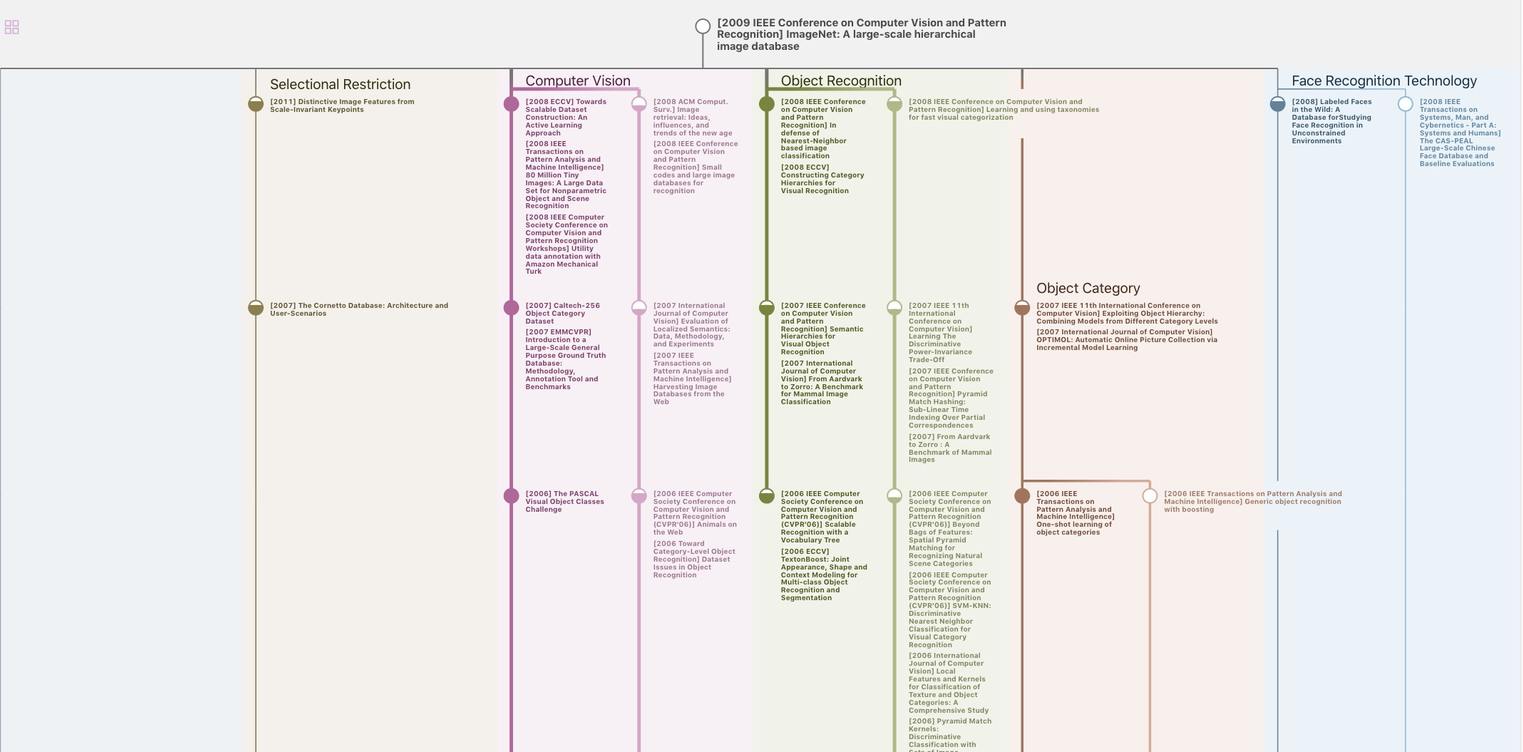
生成溯源树,研究论文发展脉络
Chat Paper
正在生成论文摘要