Kinome-Wide Profiling Prediction of Small Molecules.
CHEMMEDCHEM(2018)
摘要
Extensive kinase profiling data, covering more than half of the human kinome, are available nowadays and allow the construction of activity prediction models of high practical utility. Proteochemometric (PCM) approaches use compound and protein descriptors, which enables the extrapolation of bioactivity values to thus far unexplored kinases. In this study, the potential of PCM to make large-scale predictions on the entire kinome is explored, considering the applicability on novel compounds and kinases, including clinically relevant mutants. A rigorous validation indicates high predictive power on left-out kinases and superiority over individual kinase QSAR models for new compounds. Furthermore, external validation on clinically relevant mutant kinases reveals an excellent predictive power for mutations spread across the ATP binding site.
更多查看译文
关键词
kinases,machine learning,PCM,proteochemometrics,QSAR,random forest
AI 理解论文
溯源树
样例
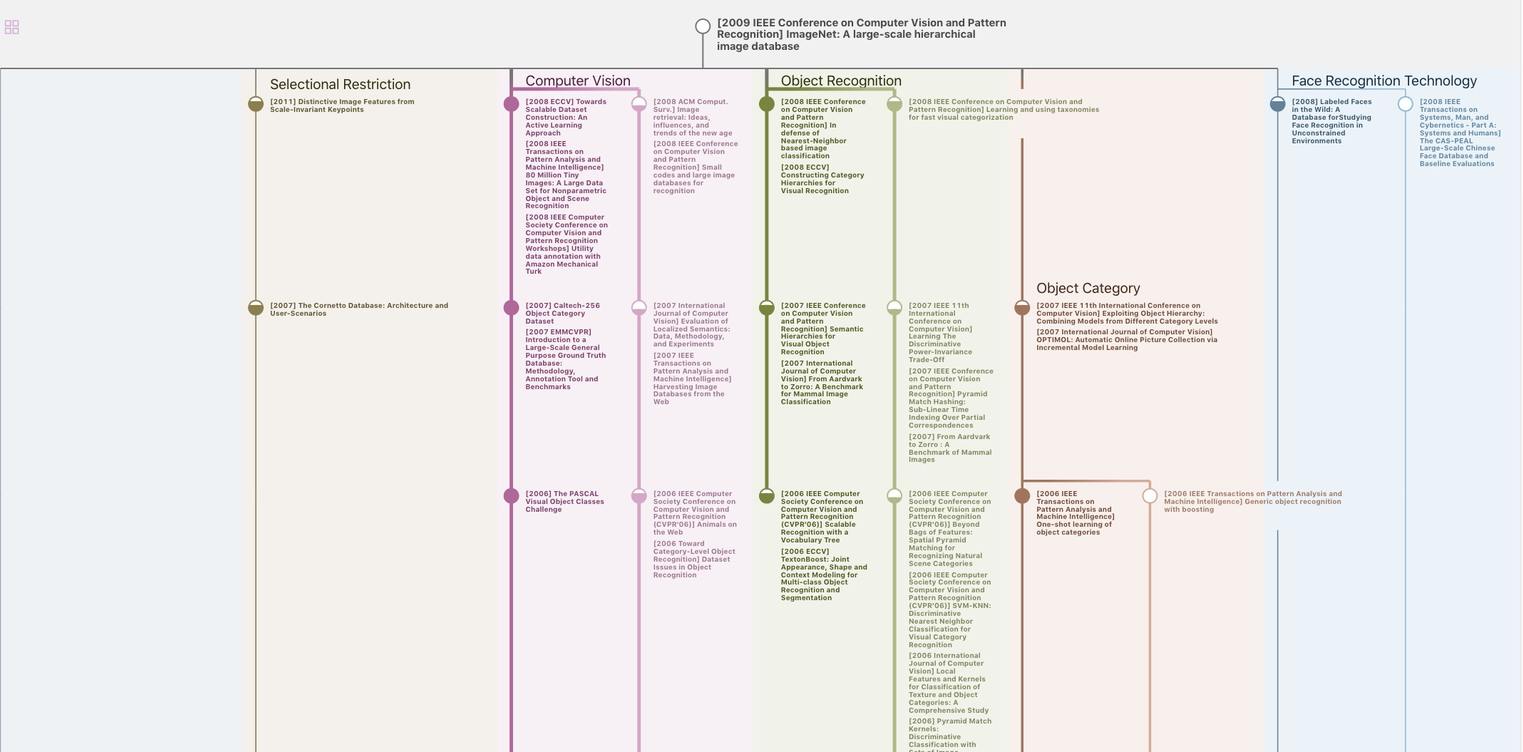
生成溯源树,研究论文发展脉络
Chat Paper
正在生成论文摘要