A Novel Unsupervised Segmentation Approach Quantifies Tumor Tissue Populations Using Multiparametric MRI: First Results with Histological Validation
Molecular imaging and biology : MIB : the official publication of the Academy of Molecular Imaging(2016)
摘要
Purpose We aimed to precisely estimate intra-tumoral heterogeneity using spatially regularized spectral clustering (SRSC) on multiparametric MRI data and compare the efficacy of SRSC with the previously reported segmentation techniques in MRI studies. Procedures Six NMRI nu/nu mice bearing subcutaneous human glioblastoma U87 MG tumors were scanned using a dedicated small animal 7T magnetic resonance imaging (MRI) scanner. The data consisted of T2 weighted images, apparent diffusion coefficient maps, and pre- and post-contrast T2 and T2* maps. Following each scan, the tumors were excised into 2–3-mm thin slices parallel to the axial field of view and processed for histological staining. The MRI data were segmented using SRSC, K -means, fuzzy C-means, and Gaussian mixture modeling to estimate the fractional population of necrotic, peri-necrotic, and viable regions and validated with the fractional population obtained from histology. Results While the aforementioned methods overestimated peri-necrotic and underestimated viable fractions, SRSC accurately predicted the fractional population of all three tumor tissue types and exhibited strong correlations (r necrotic = 0.92, r peri-necrotic = 0.82 and r viable = 0.98) with the histology. Conclusions The precise identification of necrotic, peri-necrotic and viable areas using SRSC may greatly assist in cancer treatment planning and add a new dimension to MRI-guided tumor biopsy procedures.
更多查看译文
关键词
Tumor heterogeneity,Multiparametric MRI,Spectral clustering,K-means,Fuzzy C-means,Gaussian mixture modeling
AI 理解论文
溯源树
样例
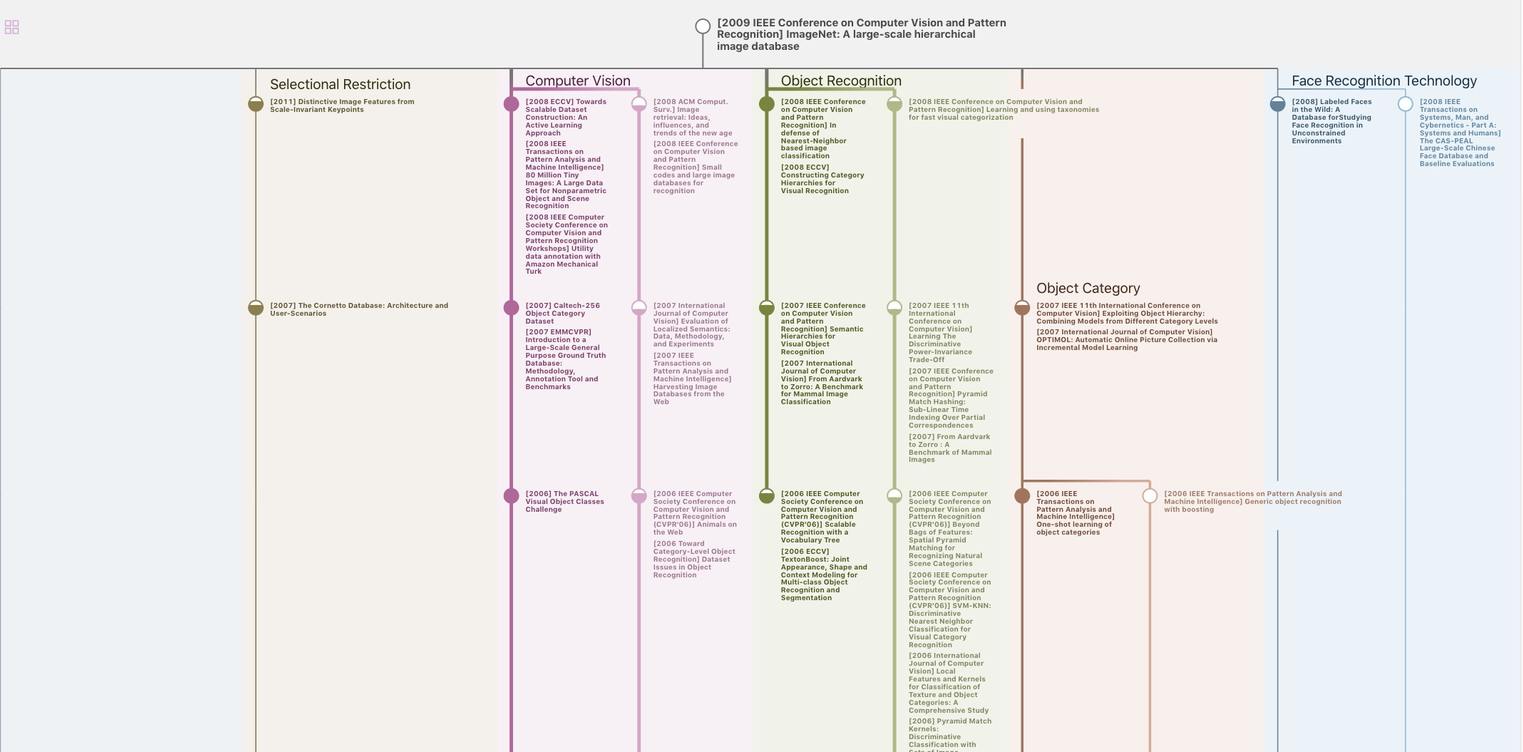
生成溯源树,研究论文发展脉络
Chat Paper
正在生成论文摘要